350 rub
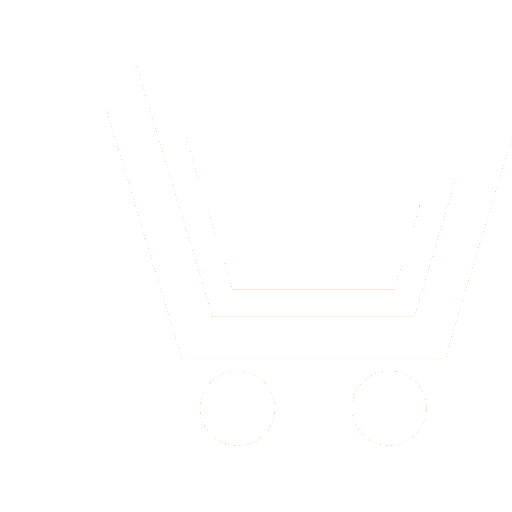
Journal Neurocomputers №8 for 2010 г.
Article in number:
Analysis of the football performance: from classical methods to neural network
Authors:
Yu. Yu. Petrunin
Abstract:
Today's Football and economic sector, and the scope of public interest, and a cultural global society. Statistics show performance and trends of development of the sport. However, the use of simple statistical methods and models may distort the true picture. Thus, the use of the mean of the impact of FIFA World Cup, leads to the conclusion it gradual decline over the past 80 years. However, if applied to analyze the impact of chart span of interval estimation of an average performance and standard deviation, no trend is observed. In this case it is advisable to use the techniques of cluster analysis showing the frequency similar to each other the world championships. Taking as variables for cluster analysis of mean performance of each championship since 1930, the standard deviation as a measure of dispersion and skewness of the distribution of goals scored in each World Cup, there are three types (cluster) championship performance. One cluster formed tournaments 1930, 1934, 1938, 1950, 1954 and 1958. It championships with high productivity, a large spread of values and a small skewness ("goal-scoring fiesta"). They can be interpreted as spectacular sports forums with a wide range of results. The last cluster is, in a sense, the opposite of the first. It fell race 1962, 1966, 1982, 1986, 1990 and 2002. This championship with a low impact and high skewness, ie the presence of individual matches with a large (relative to the total mass of games) the number of goals scored. We can say that the cluster is characterized by low productivity of a few unexpected help of bursts of activity. In the second cluster were the world championships 1970, 1978, 1994, 1998 and 2006. The impact is slightly higher than in the third cluster, scatter a little less, a very small skewness. In short, more spectacular (offensive) game, a good predictability of the number of goals scored in the match. The disadvantage of cluster analysis in this case is that the variables used to it, are not mutually independent. With increasing skewness, in particular, the information content of the mean falls. It is advisable in this case a more flexible and adequate method of self-learning neural networks (Kohonen network). Clusters obtained by self-organizing maps, differ from those obtained by classical cluster analysis. Especially important is the fact that the pre-war championship in 1934 was close to championships in 1970, 1978, 1994, 1998 and 2006, which means that the clustering is based not solely on the chronological order. The variance within the clusters for each of the variables shows that if the classical taxonomy of the decisive role in attributing to a particular cluster is the mean performance index, the neural network clustering is much more into accoutn the contribution of skewness and the standard deviation in the process of grouping similar objects. The results confirm that the development of high performance is not a one-dimensional linear process, but a more complicated pattern.
Pages: 54-62
References
- Есенин К. С. Футбол: рекорды, парадоксы, трагедии, сенсации. М.: Молодая гвардия. 1968.
- Адоян Г. А., Адоян Г. Г. Особенности изменения результативности игр в футболе // Теория и практика физической культуры. 2002. № 11. С. 37-38.
- Адоян Г. А., Адоян Г. Г. Итоги прошедшего века, или эволюция результативности футбола. М.: Спутник+. 2001.
- Адоян Г. А., Адоян Г. Г. Оценка уровня групповых турниров соревнований Кубка Мира и Лиги Чемпионов Европы по футболу // Теория и практика футбола. 2003. № 4. С. 8-10.
- Адоян Г. А., Адоян Г. Г. Влияние количества команд на уровень турнира при круговой системе соревнований по футболу // Теория и практика футбола. 2004. № 2. с. 12-13.
- Адоян Г. А., Адоян Г. Г. Механизмы и парадоксы формирования результативности в футболе и хоккее // Аспирант и соискатель. 2006. № 6. С. 101-107.
- Дебок Г., Кохонен Т. Анализ финансовых данных с помощью самоорганизующихся карт. М.: Издательский дом Альпина. 2001.
- Петрунин Ю. Ю. Классификация при ограниченной информации: микроанализ данных // Вестник МГУ. Серия 21. Управление (государство и общество). 2006. № 2. С. 30-54.
- Петрунин Ю. Ю., Зернова Ю. А. Статистические и нейросетевые методы исследования политической ситуации во Франции на примере региональных выборов 1998 и 2004 гг. // Государственное управление. Электронный вестник. 2008. № 14. http://e-journal.spa.msu.ru/14_2008Petrunin_Zernova.html
- Петрунин Ю. Ю. Исследование электорального поведения: самоорганизующиеся карты Кохонена versus статистического анализа - // Вестник МГУ. Серия 21. Управление (государство и общество). 2009. № 3. С. 45-55.
- Kohonen, N., Self-Organizing Maps. N.Y. 1995.
- Чемпионат мира по футболу 2002: Календарь-справочник. М.: ООО «Издательство АСТ»: ООО «Издательство Астрель». 2002.
- Мировой футбол 2002-03. Справочник.Составитель И. В. Гольдес. М.: Терра-спорт. 2002.
- ru.wikipedia.org/wiki/ЧМ-2006
- Rotshtein, A., Posner, M., Rakytyanska, H., Prediction of the results of football games based on fuzzy model with genetic and neuro tuning // eastern european journal of enterprise technologies. 2003. 2 (2). P. 10-18.
- Rotshtein, A. P., Posner, M., Rakityanskaya, A. B., Football Predictions Based on a Fuzzy Model with Genetic and Neural Tuning // Cybernetics and Systems Analysis Journal. 2005. V. 41. No. 4. P. 619-630.
- Loeffelholz, B., Bednar, E., Bauer, K. W., Predicting NBA Games Using Neural Networks // Journal of Quantitative Analysis in Sports. 2009. V. 5: Issue 1, Article 7.
- Halicioglu, F., Can we predict the outcome of the international football tournaments: the case of EURO 2000 - // Dogus University Journal. 2005. V. 6 (1). P. 59-69.
- Halicioglu, F., Forecasting the professional team sporting events: evidence from Euro 2000 and 2004 football tournaments // Fifth International Conference on Sports and Culture: Economic, Management and Marketing Aspects, 2005.
- http://www.soccerstats.com/
- http://www.european-football-statistics.co.uk/
- http://www.365stats.com/football
- www.fstat.obolon.ua
- stats.betportal.org
- www.fussballdaten.de
- the-english-football-archive.com
- www.rsssf.com
- www.footstat.ru