350 rub
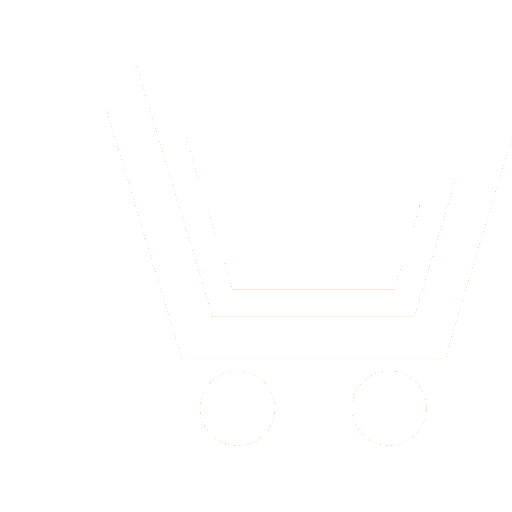
Journal Neurocomputers №6 for 2010 г.
Article in number:
Empirical mode decomposition and its application to informative component identification and signal prediction with the use of neural networks
Authors:
D. M. Klionsky, N. I. Oreshko, V. V. Geppener, I. V. Neunivakin
Abstract:
The present paper is devoted to the novel signal processing technology on the basis of empirical mode decomposition (EMD) and its applications connected with neural networks. EMD-based technology is currently widely used in various scientific applications. Its main advantage is high adaptivity to any kinds of signals processed. EMD has been found useful in preprocessing tasks, structural analysis (including component analysis and time-frequency one) and intellectual analysis of signals. The paper encompasses the main theoretical aspects of EMD and the key ideas behind the algorithm. Also several approaches to informative component extraction (involving information criteria and Hilbert-Huang spectrum) and signal prediction with the help of neural networks are expounded. Simulations were performed on the economic data reflecting the demand for curds and, as a result, prediction results were obtained for different time periods.
Pages: 43-50
References
- Клионский Д. М., Орешко Н. И., Геппенер В. В.Декомпозиция на эмпирические моды и ее использование при анализе дробного броуновского движения // Цифровая Обработка Сигналов. 2008. № 3. С. 37-45.
- Klionsky, D. M., Oreshko, N. I., Geppener, V. V., Applications of Empirical Mode Decomposition for Processing Nonstationary Signals // Pattern Recognition and Image Analysis. 2008. V. 13. No. 3. P. 390-399.
- Klionsky, D. M., Oreshko N. I., Geppener V. V., Empirical Mode Decomposition in Segmentation and Clustering of Slowly and Fast Changing Non-Stationary Signals // Pattern Recognition and Image Analysis. 2009. V. 19. No. 1. P. 14-29.
- Huang, N., Shen, S., Hilbert-Huang Transform and Its Applications // World Scientific. 2005.
- Huang, N. E., etc. The Empirical Mode Decomposition and The Hilbert Spectrum for Non-linear and Non-stationary // Time Series Analysis, Proc. Royal Soc. London. 1998. V. 454. P. 903-995.
- Хайкин С. Нейронные сети. Полный курс. М. - СПб. - Киев: Вильямс. 2006.