350 rub
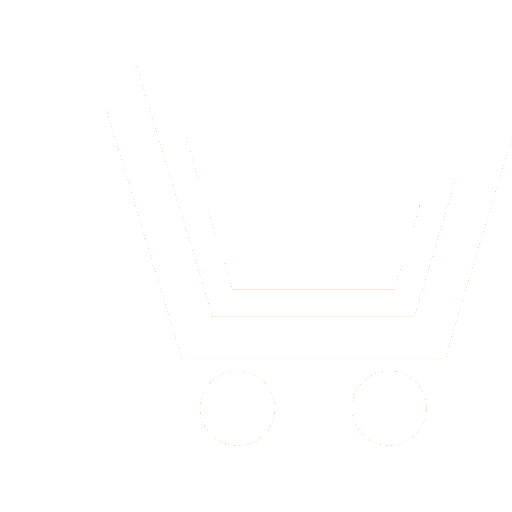
Journal Neurocomputers №4 for 2010 г.
Article in number:
Neural Network Classification in Non-homogeneous Feature Space
Authors:
A. Yu. Dorogov, V. Yu. Lesnykh, M. Yu. Shestopalov
Abstract:
Forward-propagation neural networks give a good result in classification tasks when training data fits with homogeneous behavior of an object and data classes make compact areas in feature space. An idea of usage collective classifiers arose solving the problem of pattern classification. The most difficult phase of this approach is competent areas selection for local classifiers. The competent area is a feature space object subset where the incidence of local classifier with given subset of pattern recognition is specified. The main problem is that there is no any reliable homogeneity criterion for feature field of the competent area.
Empirical data is usually introduced as «object-property» table. It covers a set of single objects regarding as individual observations of a complex system. One raw in the table with a set of characteristics corresponds to one target. Data analysis usually represents to estimation of statistical dependences between feature space variables. Using typical solutions based on correlation measures connection graph may be constructed, where degree of interdependence is expressed in pair correlation coefficients. The data may be consisting of object subsets which define different system behaviors. Statistical approach removes this pair connection differences, but transforms them to a structure level, where they appears in ternary and n-ary relations between characteristics. The task is using structure information to determine characteristic subsets presenting homogeneous behavior areas (localities), to separate typical system statuses and verify them in data table. The result observation object subsets will specify competent areas for local classifiers.
In the first section of the paper the problem of structure localization of homogeneous areas is considered. To solve the problem is suggested to use a clipping version of connection graph with connection degree equals to +1 or -1 (or 0 for no connection). Connection graph is formed on base of statistic dependences between variables of feature space. Sociological system researches pioneered the use of the graph. In models based on sign graph the task is to detect sign imbalance and find the way of removal. A new approach to the problem uses the sign graph model for reconstructive analysis of complex system. Within the bounds of new paradigm a problem of structure localization of homogeneous areas comes up to local balancing of sign graph. Primary system elements - separating structures (shares), which express logical principles of symmetry and two factor interaction, could be used as a selection tool.
Ways of designing collective neural network classifiers for non-homogeneous feature space and methods of generating integrated decisions are described in second part of the paper. It is suggested to use methods of sign graph local balancing and algorithms of system behavior stereotype selection for construction of competent areas of local classifiers. Decisions of local classifiers are integrated according to vote principle. Also experimental results for real data base with high degree of non-homogeneity are shown.
Pages: 20-33
References
- Растригин Л. А., Эренштейн Р. Х. Метод коллективного распознавания. М.: Энергоиздат. 1981(Библиотекапоавтоматике. Вып. 615).
- Cartwright, Dorwin, and Harary, F., Structural balance: A generalization of Heider-s theory. PsychologicalReview63: 277-292. 1956.
- Качанова Т. Л., Фомин Б. Ф. Метатехнология системных реконструкций: СПб: СПбГЭТУ «ЛЭТИ». 2002.
- Качанова Т. Л., Фомин Б. Ф. Технология системных реконструкций. СПб.: Политехника, 2003. (Проблемы инновационного развития. Вып. 2).
- Бахвалов Н. С. Численные методы (анализ, алгебра, обыкновенные дифференциальные уравнения). М.: Наука. 1975.
- Воеводин В. В., Кузнецов А. Ю. Матрицы и вычисления. М.: Наука. 1984.
- Дорогов А. Ю., Курбанов Р. Г., Разин В. В. Быстродействующий алгоритм семантической классификации JPEG-изображений // Нейроинформатика. Электронный журнал. 2006. Т. 1. № 2. С. 124-144. http://www.ni.iont.ru/Journal/N2/.
- Дорогов А. Ю. Математические основы методов оптимально частичной балансировки знаковых графов. Деп. в ВИНИТИ 23.07.2007, №760-В2007.