350 rub
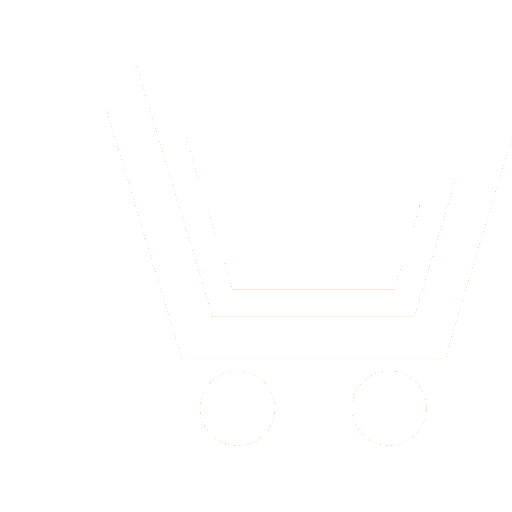
Journal Neurocomputers №3 for 2010 г.
Article in number:
Neural network as a tool for implementation of piecewise-linear classifier in mass screening of molecules in the problem of «structure-property»
Keywords:
QSAR
QSPR
SAR
Quantitative Structure Activity Relationship
artificial neural networks
fuzzy molecular descriptors
molecular graphs
Authors:
E. I. Prokhorov, A. V. Perevoznikov, L. A. Ponomareva, M. I. Kumskov
Abstract:
It is proposed to use artificial neural network (ANN) as a tool for the realization of a generalized decision tree created as a model for solving the «structure-property» problem. Radial basis neurons are used to represent the cluster structure of the training set: within existing clusters introduced the rule to refuse the forecast. In this paper, the rules-constructing task and their implementation in the form of an ANN are proposed. Proposed a solution that uses the cluster structure of the training sample. To find this structure is proposed to use the composition of two different algorithms for cluster analysis: an algorithm for constructing a minimum spanning tree and the classical k-means clustering algorithm. Calculating the distance between the molecular graphs is carried out using special space descriptors, different from the space in which we construct a function of the forecast. The results of computational experiments analyzing a sample of glycosides, which illustrate the practical effectiveness of the methodology, are proposed.
Pages: 39-45
References
- Джурс О. П., Айзенауэр Т. Распознавание образов в химии: Пер.с англ. М.: Мир. 1977.
- Стьюпер Э., Брюггер У., Джурс П. Машинный анализ связи химической структуры и биологической активности: Пер.с англ. М.: Мир. 1982.
- Baskin, I. I., Palyulin, V. A., and Zefirov, N. S., NASA. A computer program for performing QSAR/QSPR studies using artificial neural networks // QSAR and Molecular Modeling: Concepts, Computational Tools and Biological Applications. Barcelona: Prous Science Publ. 1995. P. 30-31.
- Baskin, I. I., Keshtova, S. V., Palyulin, V. A., and Zefirov, N. S., Combining Molecular Modeling with the Use of Artificial Neural Networks as an Approach to Predict Substituent Constants and Bioactivity // Molecular Modeling and Prediction of Bioactivity; K. Gundertofte; F.S. Jorgensen, Eds. Klumer Academic / Plenum Publishers: NY. 2000. Р. 468-469.
- Karelson, M., Molecular Descriptors in QSAR/QSPR. Wiley-Interscience, 2000.
- Кумсков М. И., Смоленский Е. А., Пономарева Л. А., Митюшев Д. Ф., Зефиров Н. С. Системы структурных дескрипторов для решения задач «структура-свойство» // Докл. РАН. 1994. Р. 336.
- Kumskov, M. I., Mityushev, D. F., Oshchepkov, E. N., and Kolovanov, E. A., Computer-Aided Extraction and Application of Knowledge about Structure-Property Relationship in Factographical Chemical-Structure Data Bases // Pattern Recognition and Image Analysis. 1995. V. 5. No.4. P. 589-601.
- ГОСТ Р ИСО/МЭК 12207-99. Информационная технология. Процессы жизненного цикла программных средств. М.: ИПК Издательство стандартов. 2000.
- Айвазян С. А., Бухштабер В. М., Енюков И. С., Мешалкин Л. Д. Прикладная статистика. Т. 3: Классификация и снижение размерности: Справ. изд. / под ред. С. А. Айвазяна. М.: Финансы и статистика. 1989.
- Минский М. Л., Пейперт С. Персептроны: Пер. с англ. М.: Мир. 1971.
- Handbook of Brain Theory and Neural Networks / Second Edition, Ed. by M. A Arbib.NY, MIT Press. 2002.
- Nigrin, A., Neural Networks for Pattern Recognition. NY. MITPress. 1993.
- Дюран Б., Оделл П. Кластерный анализ. М.: Статистика. 1977.
- Классификация и кластер / Под ред. Дж. Райзина. М.: Мир. 1980.
- Haykin, S., Neural Networks: a Comprehensive Foundation - Macmillan. NY. 1994.
- Буркерт У., Эллинджер Н. Л. Молекулярная механика. М.: Мир, 1986.
- Кумсков М. И. Методология прогнозирования свойств химических соединений и ее программная реализация // Автореф. дисс. - докт. физ.-мат. наук. М. 1997.
- Дидэ Э., Боши С., Бросье Ж. и др. Методы анализа данных. Подход, основанный на методе динамических сгущений: Пер. с франц. М.: Финансы и статистика. 1985.
- Деветьяров Д. А., Кумсков М. И. Использование нейронных сетей в задаче «структура-свойство» с использованием нечеткого описания пространственных структур молекул / Нейрокомпьютеры: разработка и применение. 2010. № 3.
- Прохоров Е. И., Перевозников А. В., Воропаев И. Д., Кумсков М. И., Пономарева Л. А. Поиск представления молекул и методы прогнозирования активности в задаче «структура-свойство» // Докл. XIV Всеросс. конф. «Математические методы распознавания образов (ММРО-14)». М.: МАКС Пресс. 2009. С. 589-591.
- Деветьяров Д. А., Кумсков М. И., Апрышко Г. Н., Носеевич Ф. M. и др. Сравнительный анализ применения нечетких дескрипторов при решении задачи «структура-активность» для выборки гликозидов // Докл. XIV Всеросс. конф. «Математическиеметодыраспознаванияобразов(ММРО-14)». М.: МАКСПресс. 2009. С. 511-514.