350 rub
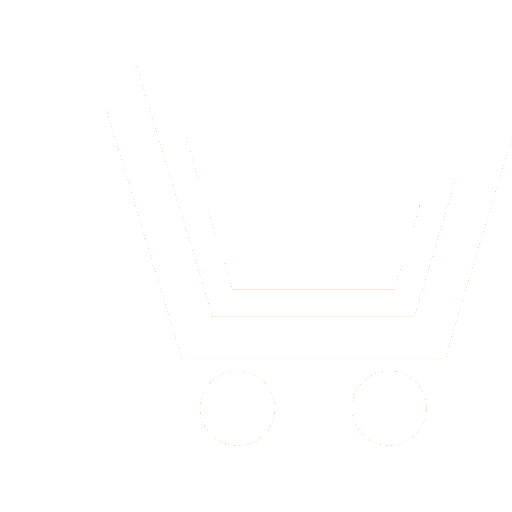
Journal Neurocomputers №12 for 2010 г.
Article in number:
Comparison of the efficiency of recognition of images for the Bayes algorithm, correlation and modified Hopfield network
Keywords:
the modified Hopfield Network
Bayes algorithm
correlation algorithm
characteristics of correct Recognition of images
Authors:
Yu. A. Basistov, Yu. G. Yanovsky
Abstract:
We consider the problem of recognition of two binary images of dimension 23x23 noised by additive uncorrelated noise. We have to solve this problem using the Bayesian algorithm, the correlation and a modified Hopfield neural network. For the distribution of interference such as "salt and pepper," the performance of the correlation algorithm and a modified Hopfield network was shown. For equal probabilities of images from each class of images, Gaussian noise distribution with known mean and known correlation matrix of 1000 realizations for each fixed ratio "signal / noise» produced statistical estimates of the probability of correct recognition for the Bayes algorithm, correlation and a modified Hopfield network. Recognition characteristics are constructed in the form of dependence of the probability of correct recognition to ratio "signal / noise». From the analysis of characteristics, it follows that the modified Hopfield network reached the limit of quality equal to the quality of recognition for the Bayes algorithm. For learning the modified Hopfield network only a single presentation of reference images of each class of images was used. The correlation algorithm has lost about 3% of the probability of correct recognition to the Bayes algorithm, and to the modified Hopfield network. If we do not have a priori distribution of interference, the alleged modified Hopfield network is better on the probability than correct recognition to the Bayes algorithm, which is reflected on the characteristics of recognition for the different probabilities of anomalous emission in the distribution of interference.
Pages: 37-44
References
- Gonzales, R. C., Woods, R. E., Digital Image Processing // 2nd ed, Prentice Hall. Upper Saddle River. NG. 2002.
- Гонзалес Р., Вудс Р., Эддинс С. Цифровая обработка изображений в среде MATLAB. М.: «Техносфера». 2006.
- Цивлин И., Форштер А., Кадышев С. Особенности автоматического распознавания реальных радиолокационных изображений // Фазотрон. 2006. № 3-4 (7). С. 27-30.
- Хайкин С. Нейронные сети. М. - СПб. - Киев: Вильямс. 2006.
- Новости с Российского рынка нейрокомпьютеров. http://neurnews.iu4.bmstu.ru.
- Двухпроцессорный встраиваемый модуль МЦ 4.01 (NM1). http://www.module.ru.
- Басистов Ю. А., Яновский Ю. Г. Оценки вероятностей правильного распознавания изображений для алгоритмов Байеса, корреляционного и модифицированной сети Хопфилда // Тр. XVМеждународной конференции по нейрокибернетике. Т. 2. «3-йМеждународныйсимпозиумпонейроинформатикеинейрокомпьютерам». С. 49-51.
- Li, J., Michel, A. N., and Porod, W., Analysis and Systhesis of a Class of Neural Networks: Linear Systems Operating on a Closed Hypercube // IEEE Transactions on Circuits and Systems. 1989. V. 36, No. 11. P. 1405-1422.