350 rub
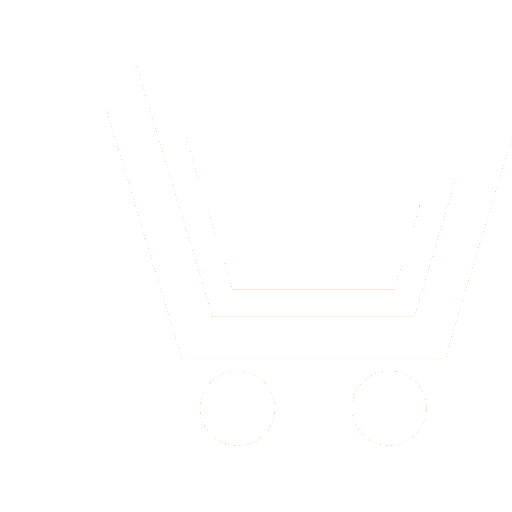
Journal Neurocomputers №12 for 2010 г.
Article in number:
Synthesis and identification of hidden Markov models for fatigue damage diagnostics
Authors:
L. S. Kuravsky, S. N. Baranov, G. A. Yuryev
Abstract:
Under consideration are technologies for synthesis and identification of hidden Markov models which are based on a novel technique and applied for fatigue damage diagnostics. The approach can be used for both continuous- and discrete-time systems. An initial rough model results from statistical multivariate analysis of observed data or their analysis by means of Kohonen self-organizing feature maps. Then it suffers proper corrections. Histograms of observed frequencies of being in different system states after the given exploitation periods are employed to train the constructed models. Free model parameters are identified by the method of chi-square minimum. The synthesis may be carried out in case of some uncertainties: absence of complete information on system states and their connections is assumed. Undefined observed cases are partially identified by the method of classification propagation with the aid of either self-organizing feature maps or cluster analysis. Connections between different system states are determined according to contiguity of their clusters or areas of winning units on the topological maps and statistical goodness-of-fit tests. In case of models represented by discrete-time structures (Markov chains) conversion to continuous-time trained structures (Markov networks) is performed to obtain more reliable results with the following return to discrete time and identification of transition probabilities. The methods presented are available for specialists responsible for maintenance of technical products. Obtained Markov models are useful for diagnostics, forecasting structure service lives and establishing the justified routine maintenance. An example of synthesis and identification of Markov models representing fatigue damages of an aircraft air-intake panel is given for illustration.
Pages: 20-36
References
- Baranov S. N.and Kuravsky, L. S.,Acoustic vibrations: modeling, optimization and diagnostics, 2nd Edition, Enlarged, Moscow: Rusavia. 224 pp. 2006.
- Baum, L. E., Petrie, T., Soules, G., and Weiss, N., A maximization technique occurring in the statistical analysis of probabilistic functions of Markov chains // Ann. Math. Statist. 1970. V. 41. No. 1. P. 164-171.
- Bendat, J. S. and Piersol, A. G.,Random data. Analysis and measurement procedures. New York: John Wiley & Sons. 1986.
- Bishop, Y. M. M., Fienberg, S. E., and Holland, P. W., Discrete multivariate analysis: Theory and practice. Cambridge. MA: M. I. T. Press. 1975.
- Bogdanoff, J. L. and Kozin, F.,Probabilistic Models of Cumulative Damage. New York: John Wiley & Sons. 1985.
- Brousset, C. and Baudrillard, G.,Neural network for automating diagnosis in aircraft inspection // Review of Progress in Quantitative Nondestructive Evaluation (Ed. by D. O. Thompson and D. E. Chimenti), Plenum Press. New York. 1993. V. 12. P.797-802.
- Cramer, H.,Mathematical methods of statistics. Princeton: Princeton University Press. 1946.
- Kohonen, T.,Self-organizing maps. Heidelberg: SpringerVerlag. 1995.
- Куравский Л. С., Баранов С. Н.Применение нейронных сетей для диагностики и прогнозирования усталостного разрушения тонкостенных конструкций // Нейрокомпьютеры: разработка и применение. 2001. № 12. С. 47-63.
- Kuravsky, L. S., and Baranov, S. N., Condition monitoring of the structures suffered acoustic fatigue failure and forecasting their service life // Proc. ConditionMonitoring. 2003. Oxford.UnitedKingdom.p. 256-279. July.
- Куравский Л. С., Баранов С. Н.Дискриминантные сети в задачах диагностики // Нейрокомпьютеры: разработка и применение.2003. № 8-9. с. 3-9.
- Kuravsky, L. S.and Baranov, S. N., Neural networks in fatigue damage recognition: Diagnostics and statistical analysis. // Proc. 11th international congress on sound and vibration.St.-Petersburg.Russia. p. 2929-2944. July. 2004.
- Kuravsky L. S. and Baranov S. N. Synthesis of Markov networks for forecasting fatigue failures // Proc. condition monitoring 2003. Oxford. United Kingdom. p. 76-91. July.
- Kuravsky L. S.and Baranov S. N.,The concept of multifactor Markov networks and its application to forecasting and diagnostics of technical systems // Proc. condition monitoring. 2005. Cambridge, Unitedkingdom.p. 111-117. July.
- Куравский Л.С., Баранов С. Н., Малых С. Б. Нейронные сети в задачах прогнозирования, диагностики и анализа данных: Учеб. пособие. М.: РУСАВИА. 2003.
- Лоули Д., Максвелл А.Факторный анализ как статистический метод. М.: Мир. 1967.
- Marple, S. L., Jr.,Digital spectral analysis with applications. New Jersey: Prentice-Hall. 1987.
- Pidaparti, R. M. V. and Palakal, M. J.,Neural network approach to fatigue-crack-growth predictions under aircraft spectrum loadings // Journal of Aircraft. V. 32. P. 825-831. 1995.
- Rabiner, L. R., A tutorial on hidden Markov models and selected applications in speech recognition // Proc. IEEE. 1989. V. 77. No. 2. P. 257-286.
- Viterbi, A. J., Error bounds for convolutional codes and an asymptotically optimum decoding algorithm. IEEE Transactions on Information Theory. 1967. V. 13. No. 2 P. 260-269.