350 rub
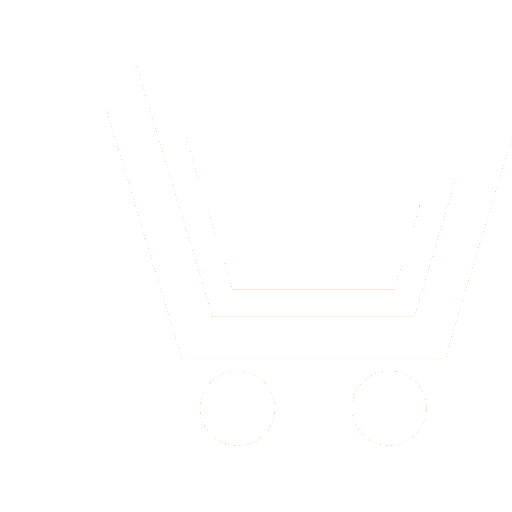
Journal Neurocomputers №10 for 2010 г.
Article in number:
Rise of the degree of functional completeness neural-like units of model of the neural network
Authors:
E. A. Samoilin
Abstract:
As it is known, models of artificial neural networks (NN) are under construction on the multilayered architecture as single-layered networks, as well as lying in their basis neural-like units (NLU) are limited in approximating (approach, implementation) some simple functions which have received the name "is linear-inseparable". For example, the problem which has become by a classical example «eXcluding OR» visually shows impossibility of approximating NLU of two logic (Boolean) functions (inadequacy and equivalence) from a totality =16 from n=2 variables.
Proceeding from it, the purpose of offered operation improvement of the common approximating possibilities of models of NN on the basis of rise of a degree of their functional entirety NLU. Thus the degree of functional entirety of a network is understood as the ratio of number of functions from beforehand set (complete) set which the NN model is capable to approximate (after appropriate procedures of training), to a totality of these functions.
In operation rise of a degree of functional completeness NLU on the basis of usage biorthosigmoid nonlinearities as function of activation NLU is considered. For the first time the following theorem is formulated and proved: there are such numbers a, b and c, a set of values of scales , at which NLU the low order, a having additional input , and also biorthosigmoid function of activation of sort , in case of provides
functional -completeness, i.e. entirety in a class of logical functions from n variables
The proof of the given theorem resulted in article, is grounded on the analysis of compatibility of the equations in all set of equationses constructed according to mathematical model NLU and each of 16 approximable functions.
The mechanism of training NLU with biorthosigmoid the activation function, grounded on gradient a rule of the quickest descent is considered. Speakers of setup of weight coefficients, and also surfaces of response НПЭ are resulted at approximating training to is linear-inseparable functions of inadequacy («eXcluding OR») and equivalence.
According to the formulated and proved theorem, and also results of modelling of learning process, NLU with biorthosigmoid function of activation in case of provides functional -completeness in a class of 16 logical functions from 2 variables. For a case of three-dimensional Boolean functions ( ), rise of a degree of functional entirety has made with to , i.e. the number realized NLU functions has increased with 104 to 176 (in 1.7 times).
Pages: 17-26
References
- Rutkowska, D. , Pilinski, M., Rutkowski, L., Sieci neuronowe, algorytmy genetyczne I systemy rozmyte. Wydawnictwonaukowepwn. Warszawa LODZ, 1999. (Рус. перевод: Рутковская Д., Пилиньский М., Рутковский Л. Нейронные сети, генетические алгоритмы и нечеткие системы: Пер. с польск. И. Д. Рудинского.М.: Горячая линия-Телеком. 2004.)
- Osowski, S., Sieci neuronowe do przetwarzania informacji // Oficyna mydawnicza politechniki Warszawskiej, Warszawa, 2000. (Рус. перевод: Осовский С. Нейронные сети для обработки информации: Пер. с польск. И. Д. Рудинского. М: Финансы и статистика. 2004.)
- Круглов В. В., Борисов В. В. Искусственные нейронные сети. Теория и практика. М.: Горячая линия - Телеком. 2001.
- Wasserman, P.,Neurocomputing. Theoryandpractice, NostramReinhold. 1990. (Рус. перевод: Уоссермен Ф. Нейрокомпьютерная техника: теория и практика: Пер. с англ. Ю. А. Зуев, В. А. Точенов. М.: Мир. 1992.)
- Патент РФ № 2269155; от 27.01.2007. Модель нейрона, реализующая логическую функцию неравнозначности // Самойлин Е.А. 2006.
- Патент РФ № 2308758. от 20.10.2007. Способ реализации нейроном логической функции неравнозначности // Самойлин Е.А. 2007.
- Stone, M. N., The Generalized Weierstrass Approximation Theorem // Math. Mag. 1948. V. 21. P. 167-183, 237-254.
- Колмогоров А. Н.О представлении непрерывных функций нескольких переменных в виде суперпозиции непрерывных функций одного переменного // Докл. АН СССР. 1957. Т. 114. № 5. С. 953-956.
- Арнольд В. И. О представлении функций нескольких переменных в виде суперпозиции функций меньшего числа переменных // Математическое просвещение. 1958. Вып. 3. С. 41-61.
- Cybenko, G., Approximation by Superposition of a Sigmoidal Function // Mathematics of Control, Signals, and Systems. 1989. V. 2. P. 303-314.
- Терехов С. А., Горбань А.Н., Дунин-Барковский В.Л., Кирдин А.Н. и др. Нейросетевые информационные модели сложных инженерных систем // Сб. Нейроинформатика. Новосибирск: Наука. Сибирская издательская фирма РАН. 1998. С. 103-141.
- Карпов Ю. Г. Теория автоматов. СПб.: Питер. 2003.