350 rub
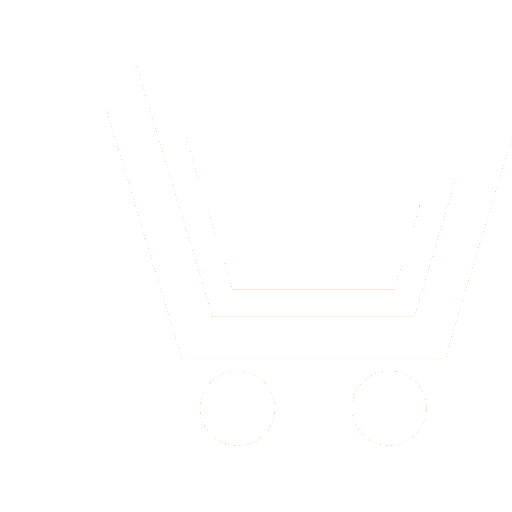
Journal Neurocomputers №9 for 2009 г.
Article in number:
Application of trained multifactor Markov networks to speech phonological awareness study
Authors:
L.S. Kuravsky, E.G. Ivanova
Abstract:
Trained multifactor Markov networks that make it possible to represent subtle features of development of psychological characteristics and study their interaction are under consideration. These structures may be treated as a special kind of neural networks. Decomposition technique simpliying significantly both the model identification and calculation of state probability functions is presented. Following this technique, an initial system under study is decomposed into certain derived subsystems of fewer dimensions, with the states representing state sets of the former system. Each subsystem is identified separately. As a result, obtained probability functions of the state sets may be recalculated into ones of the initial system. Shown examples of 2-D and 3-D multifactor Markov networks representing development and interaction of phonological awareness characteristics demonstrate new types of characteristics suitable for further psychological analysis. The concept in question yields new information for analysis that cannot be obtained with the aid of other approaches.
Pages: 33-38
References
- Bollen K.A. Structural equations with latent variables.New York. John Wiley. 1989.
- Branum-Martin L., Carlson C.D., Carlo M., Fletcher J.M., Francis D.J., Mehta P.D., Ortiz A. Bilingual phonological awareness: Multilevel construct validation among Spanish-speaking kindergarteners in transitional bilingual education classrooms // Journal of Educational Psychology. 2006. V. 98. No. 1. Р. 170-181.
- Jöreskog K.G. Estimation and testing of simplex models // British Journal of Mathematical and Statistical Psychology. 1970. V. 23. Р. 121-145.
- Kuravsky L. S., Baranov S. N. Condition monitoring of the structures suffered acoustic fatigue failure and forecasting their service life // In: Proc. Condition Monitoring 2003. Oxford, United Kingdom. July 2003. Р. 256-279.
- Kuravsky L. S., Baranov S. N. Synthesis of Markov networks for forecasting fatigue failures // In: Proc. Condition Monitoring 2003. Oxford. United Kingdom. July 2003. Р. 76-91.
- Kuravsky L. S., Malykh S. B. Application of Markov models for analysis of development of psychological characteristics // Australian Journal of Educational & Developmental Psychology. 2004. V. 2. P. 29-40.
- Kuravsky
L. S., Malykh S. B. On the application of queuing theory for analysis of
twin data // Twin Research. 2000. V. 3.
P. 92-98. - Kuravsky L.S. and Baranov S.N. The concept of multifactor Markov networks and its application to forecasting and diagnostics of technical systems // In: Proc. Condition Monitoring 2005. Cambridge. United Kingdom. July 2005. P. 111-117.
- Kuravsky L.S., Baranov S.N. Neural networks in fatigue damage recognition: diagnostics and statistical analysis // In: Proc. 11th International Congress on Sound and Vibration. St.-Petersburg. Russia. July 2004. P. 2929-2944.
- Крамер Г. Математические методы статистики. М.: Мир. 1976.
- Куравский Л.С., Баранов С. Н., Малых С. Б. Нейронные сети в задачах прогнозирования, диагностики и анализа данных: Учеб. пособие. М.: РУСАВИА. 2003.