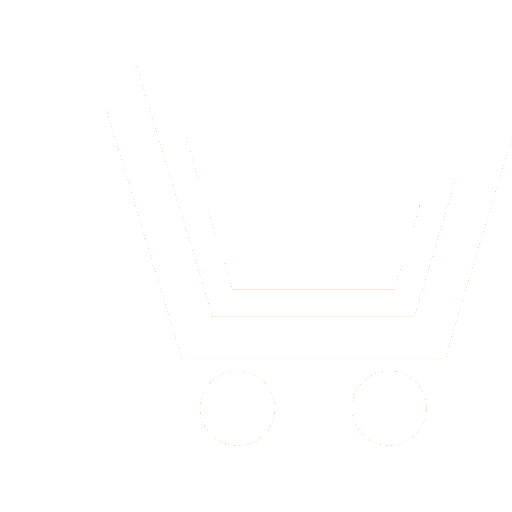
A.A. Zhurina1, A.A. Kochkarov2, R.A. Kochkarov3
1−3 Financial University under the Government of the Russian Federation (Moscow, Russia)
1 alena21081998@yandex.ru, 2 akochkarov@fa.ru, 3 rkochkarov@fa.ru
To analyze social network data, it is necessary to recognize their structure in the form of a graph and find the characteristics of the entire graph and its individual parts.
Goal – conduct a review of well-known social networks, offer characteristics for social graphs, test on one of the social networks.
Concomitant factors of information analysis in social networks are proposed. A review of tools for analyzing social networks, including data in the form of text and structural links, was carried out. Automatic analysis tools built into social networks and third-party developers are proposed. The metrics of the analysis of social graphs are considered, the social graph is visualized on the example of one of the users of the social network VK, significant nodes are determined.
The proposed tools and algorithm of actions will make it possible to carry out a structural analysis of the social graphs of individual users or large graphs of known social networks.
Zhurina A.A., Kochkarov A.A., Kochkarov R.A. Analysis of the structural characteristics of social networks. Information-measuring and Control Systems. 2023. V. 21. № 5. P. 63−72. DOI: https://doi.org/10.18127/j20700814-202305-08 (in Russian)
- Khotilin M.I., Blagov A.V. Vizualnoe predstavlenie i klasternyi analiz sotsialnykh setei.
URL: http://repo.ssau.ru/bitstream/Informacionnye-tehnologii-i-nanotehnologii/Vizualnoe-predstavlenie-i-klasternyi-analiz-socialnyh-setei-60918/1/1067-1072.pdf (data obrashcheniya: 23.06.2023). (in Russian) - Tan W., Blake M.W., Saleh I., Dustdar S. Social-network-sourced big data analytics. IEEE Internet Computing. 2013. № 5. P. 62−69.
- Bernovski M.M., Kuzyurin N.N. Sluchainye grafy, modeli i generatory bezmasshtabnykh grafov. Trudy Instituta sistemnogo programmirovaniya RAN. 2012. T. 22. URL: https://www.ispras.ru/proceedings/docs/2012/22/isp_22_2012_419.pdf (data obrashcheniya: 23.06.2023). (in Russian)
- Batura T.V. Metody avtomaticheskoi klassifikatsii tekstov. Programmnye produkty i sistemy. 2017. T. 30. № 1. S. 85−99. (in Russian)
- Garshin V.V., Kalabukhov K.S., Stepantsov V.A., Smotrov S.V. Razrabotka sistemy analiza tonalnosti tekstovoi informatsii. URL: http://www.vestnik.vsu.ru/pdf/analiz/2017/03/2017-03-21.pdf (data obrashcheniya: 23.06.2023). (in Russian)
- Blagov A., Rytcarev I., Strelkov K., Khotilin M. Big Data Instruments for Social Media Analysis. Proceedings of the 5th International Workshop on Computer Science and Engineering. 2015. P. 179−184.
- Korshunov A., Beloborodov I., Buzun N. i dr. Analiz sotsialnykh setei: metody i prilozheniya. Trudy Instituta sistemnogo programmirovaniya RAN. 2014. T. 26. № 1. S. 439−456. (in Russian)
- Allen-Perkins A., Pastor J.M., Estrada E. Two-walks degree assortativity in graphs and networks. Applied Mathematics and Computation. 2017. V. 255. P. 1−15.
- Twitter US Airline Sentiment. URL: https://www.kaggle.com/crowdflower/twitter-airline-sentiment#Tweets.csv (data obrashcheniya: 23.06.2023).
- Savvateev A. Modeli interneta i sotsialnykh setei. URL: https://habr.com/ru/post/458454/ (data obrashcheniya: 26.06.2023). (in Russian)
- Analiz druzheskikh svyazei VK s pomoshchyu Python. URL: https://habr.com/ru/post/221251/ (data obrashcheniya: 26.06.2023). (in Russian)
- Opisanie metodov API. URL: https://vk.com/dev/methods (data obrashcheniya: 26.06.2023). (in Russian)