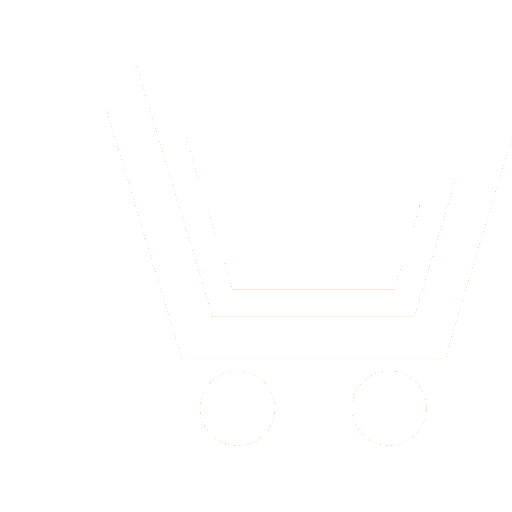
V.V. Melentiev1, D.V. Serdechnyi2, P.V. Nikitin3, S.A. Korchagin4
1 Engels Institute of Technology (branch) of Yuri Gagarin State Technical University of Saratov (Engels, Russia),
2−4 Financial University under the Government of the Russian Federation (Moscow, Russia)
1 melen2004@inbox.ru, 2dvserdechny@fa.ru, 3pvnikitin@fa.ru, 4sakorchagin@fa.ru
To conduct an intellectual analysis of government contracts, build high-quality models for predicting the execution of contracts, search for patterns and other valuable information, we need data on the contracts themselves, which are publicly available in various information systems. At present, such collection of such data is a laborious task. The parsing system allows you to automate the process of collecting data and pre-processing them for the subsequent construction of machine learning models and intellectual analysis.
The aim of the work is to develop a parsing system for automatically collecting and processing information about government contracts, as well as preparing data for their use in machine learning algorithms. As a result of the study, a parsing system was created that allows collecting information about government contracts from various sources, as well as conducting their automatic analysis using machine learning methods. The practical significance of this work lies in the possibility of using the developed system to improve the efficiency of public procurement management and increase their transparency.
Melentiev V.V., Serdechnyi D.V., Nikitin P.V., Korchagin S.A. Development of a parsing system for the analysis of government contracts. Information-measuring and Control Systems. 2023. V. 21. № 5. P. 36−47. DOI: https://doi.org/10.18127/j20700814-202305-05 (in Russian)
- Puzyrev S.A., Solukova A.A. Analiz mekhanizma regulirovaniya gosudarstvennykh zakupok v Evropeiskom soyuze. Vestnik Moskovskogo universiteta MVD Rossii. 2019. № 5. S. 263−267. (in Russian)
- Popova A.A., Serdechnyi D.V., Korchagin S.A., Nikitin P.V. Ekonomicheskie aspekty vnedreniya avtomaticheskikh sistem upravleniya v deyatelnost proizvodstvennykh organizatsii. Vestnik Altaiskoi akademii ekonomiki i prava. 2023. № 2-1. S. 86−90. (in Russian)
- Samuels D. Government procurement and changes in firm transparency. The Accounting Review. 2021. T. 96. № 1. S. 401−430.
- Jiménez A., Hanoteau J., Barkemeyer R. E-procurement and firm corruption to secure public contracts: The moderating role of governance institutions and supranational support. Journal of Business Research. 2022. T. 149. S. 640−650.
- Zhang X., Yousaf H.M.A.U. Green supply chain coordination considering government intervention, green investment, and customer green preferences in the petroleum industry. Journal of Cleaner Production. 2020. T. 246. S. 118984.
- Malkina M.Yu., Vinogradova A.V. Kak rossiiskie instituty vliyayut na effektivnost goszakaza. Vseros. ekonomicheskii zhurnal EKO. 2020. № 3 (549). S. 8−29. (in Russian)
- Molokanov A.I. Sovershenstvovanie roli bankov v sisteme gosudarstvennogo zakaza v usloviyakh razvitiya innovatsionnykh finansovykh tekhnologii. Nauka i biznes: puti razvitiya. 2020. № 9. S. 183−186. (in Russian)
- Akhmedov R.T. Korruptsiya v sfere gosudarstvennykh zakazov. Forum molodykh uchenykh. 2020. № 1 (41). S. 47−54. (in Russian)
- Felbermayr G. et al. The global sanctions data base. European Economic Review. 2020. T. 129. S. 103561.
- Moldobaev T.Sh. Tsifrovye instrumenty analiza dannykh po somnitelnym kontragentam. Tsifrovaya transformatsiya dlya ukrepleniya ekonomicheskogo potentsiala strany i uluchsheniya kachestva zhizni lyudei. S. 29. (in Russian)
- Engin Z., Treleaven P. Algorithmic government: Automating public services and supporting civil servants in using data science technologies. The Computer Journal. 2019. T. 62. № 3. S. 448−460.
- Janssen M. et al. A framework for analysing blockchain technology adoption: Integrating institutional, market and technical factors. International Journal of Information Management. 2020. T. 50. S. 302−309.
- Gurin O. Iyulskie popravki k Zakonu № 44−FZ: novaya konfiguratsiya kontraktnoi sistemy. Progoszakaz.RF. 2021. № 9. S. 6. (in Russian)
- Fetisova Ya.R. Obzor praktiki: naibolee rasprostranennye sposoby zakupok po 223−FZ i 44−FZ. StudNet. 2022. T. 5. № 1. S. 743−753. (in Russian)
- Aristarkhova M.K., Zueva O.K., Perevezentseva A.Yu. Metodika otsenki effektivnosti ispolneniya gosudarstvennogo zakaza. Journal of new economy. 2017. № 1 (69). S. 47−62. (in Russian)
- Styrin E.M., Rodionova Yu.D. Edinaya informatsionnaya sistema v sfere zakupok kak gosudarstvennaya tsifrovaya platforma: sovremennoe sostoyanie i perspektivy. Voprosy gosudarstvennogo i munitsipalnogo upravleniya. 2020. № 3. S. 49−70. (in Russian)
- Kushnarev V.V. Organizatsionno-ekonomicheskii mekhanizm logistizatsii sistemy goszakupok. Vestnik Rostovskogo gosudarstvennogo ekonomicheskogo universiteta (RINKh). 2014. № 2 (46). S. 69−72. (in Russian)
- Lyabakh A.Yu., Kulikov V.S. Analiz edinoi informatsionnoi sistemy v sfere zakupok i puti ee sovershenstvovaniya. GosReg: gosudarstvennoe regulirovanie obshchestvennykh otnoshenii. 2017. № 2. S. 18−18. (in Russian)
- Thivaharan S., Srivatsun G., Sarathambekai S. A survey on python libraries used for social media content scraping. 2020 International Conference on Smart Electronics and Communication (ICOSEC). IEEE. 2020. S. 361−366.
- Patel J.M., Patel J.M. Web scraping in python using beautiful soup library. Getting Structured Data from the Internet: Running Web Crawlers/Scrapers on a Big Data Production Scale. 2020. S. 31−84.
- Nagpal A., Gabrani G. Python for data analytics, scientific and technical applications. 2019 Amity international conference on artificial intelligence (AICAI). IEEE. 2019. S. 140−145.
- Hug N. Surprise: A Python library for recommender systems. Journal of Open Source Software. 2020. T. 5. № 52. S. 2174.
- Gayoso A. et al. A Python library for probabilistic analysis of single-cell omics data. Nature biotechnology. 2022. T. 40. № 2. S. 163−166.
- Ravasi M., Vasconcelos I. PyLops – A linear-operator Python library for scalable algebra and optimization. SoftwareX. 2020. T. 11. S. 100361.
- Cam N.T. et al. Detect malware in android firmware based on distributed network environment. 2019 IEEE 19th International Conference on Communication Technology (ICCT). IEEE. 2019. S. 1566−1570.
- Doroshenko I.A. Creating a virtual assistant using Python. LinguaNet. 2021. S. 15−18.
- Ilichev V.Yu., Yurik E.A. Analiz massivov dannykh s ispolzovaniem biblioteki Pandas dlya Python. Nauchnoe obozrenie. Tekhnicheskie nauki. 2020. № 4. S. 41−45. (in Russian)
- Harris C.R. et al. Array programming with NumPy. Nature. 2020. T. 585. № 7825. S. 357−362.
- Sial A.H., Rashdi S.Y.S., Khan A.H. Comparative analysis of data visualization libraries Matplotlib and Seaborn in Python. International Journal. 2021. T. 10. № 1.
- Timokhin P.Yu., Mikhailyuk M.V. Metod izvlecheniya poverkhnostei urovnya na GPU s pomoshchyu programmiruemoi tesselyatsii. Programmirovanie. 2020. T. 490. № 3. S. 66−72. (in Russian)