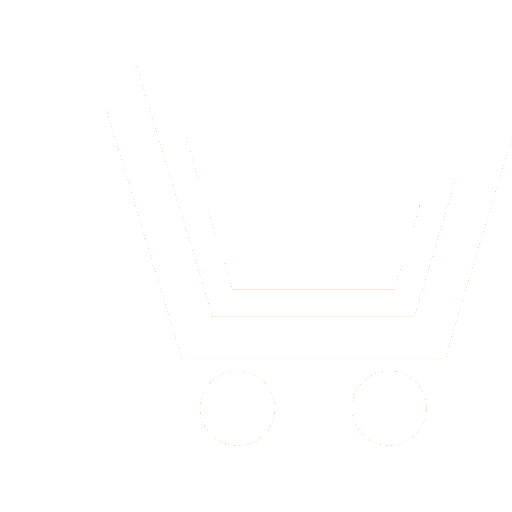
N.A. Andriyanov1, A. N. Alyunov2, M.A. Morozov3
1,2,3 Financial University under the Government of Russian Federation (Moscow, Russia)
1 naandriyanov@fa.ru, 2 analyunov @fa.ru, 3 mikal12@yandex.ru
Formulation of the problem. The task of recognizing road signs in an unmanned vehicle is, on the one hand, a well-known problem for which deep neural networks have been proposed, but, on the other hand, it is a new and difficult task in terms of implementing such computational algorithms on embedded or single-board devices. At the same time, connecting a powerful server to a car that requires a lot of calculations can be very difficult.
Target. The main goal of this work is the implementation of high-precision algorithms for detecting and recognizing traffic signs and their transfer for execution on a single-board computer NVIDIA Jetson Nano 2 GB. To achieve this goal, the algorithms themselves are first developed based on convolutional neural networks, and then the trained model is distilled so that it is more lightweight and works in a productive mode on a single-board computer.
Results. The article discusses methods for recognizing and detecting objects in images, and also implements models based on convolutional networks of the R-CNN family. High recognition metrics were obtained up to 98% F-score on the workstation and up to 92% F-score after distillation of the model.
Practical significance. The developed distilled models can be useful when implementing a recognition system in mobile devices, since such models do not require large computing power and are much less demanding on energy costs, which, for example, plays a very important role in the case of introducing such systems in unmanned vehicles.
Andriyanov N.A., Alyunov A.N., Morozov M.A. Development of an intelligent traffic sign recognition system based on computer vision and distillation methods. Information-measuring and Control Systems. 2023. V. 21. № 5. P. 27−35. DOI: https://doi.org/10.18127/j20700814-202305-04 (in Russian)
- Komzalov A.M., Shilov N.G. Primenenie sovremennykh tekhnologii v sistemakh pomoshchi voditelyu avtomobilya. Izvestiya vysshikh uchebnykh zavedenii. Priborostroenie. 2017. № 60(11). S. 1077−1082. (in Russian)
- Smirnov A., Lashkov I. State-of-the-art analysis of available advanced driver assistance systems. Proc. of the 17th Conf. Open Innovations Assocciation Fruct. 2015. S. 345−349.
- Andriyanov N.A., Orlov E.A. Razrabotka modeli mashinnogo obucheniya dlya otsenki sostoyaniya glaz voditelya. Inzhenernyi vestnik Dona. 2022. № 5(89). S. 142−159. (in Russian)
- Andriyanov N.A. Application of Computer Vision Systems for Monitoring the Condition of Drivers Based on Facial Image Analysis. Pattern Recognit. Image Anal. 2021. 31. P. 489−495. DOI: 10.1134/S1054661821030020.
- Triki N., Karray M., Ksantini M. A Real-Time Traffic Sign Recognition Method Using a New Attention-Based Deep Convolutional Neural Network for Smart Vehicles. Appl. Sci. 2023. 13. 4793. DOI: 10.3390/app13084793.
- Andriyanov N.A., Dementev V.E., Tashlinskii A.G. Obnaruzhenie ob'ektov na izobrazhenii: ot kriteriev Baiesa i Neimana–Pirsona k detektoram na baze neironnykh setei EfficientDet. Kompyuternaya optika. 2022. T. 46. № 1. S. 139−159. DOI: 10.18287/2412-6179-CO-922. (in Russian)
- Zhou W., Fan H., Zhu J., Wen H., Xie Y. Research on Generalized Hybrid Probability Convolutional Neural Network. Appl. Sci. 2022. 12. 11301. DOI: 10.3390/app122111301.
- Sikorskii O.S. Obzor svertochnykh neironnykh setei dlya zadachi klassifikatsii izobrazhenii. Novye informatsionnye tekhnologii v avtomatizirovannykh sistemakh. 2017. № 20. S. 37−42. (in Russian)
- Kamalova Yu.B., Andriyanov N.A. Raspoznavanie mikroskopicheskikh izobrazhenii pyltsevykh zeren s pomoshchyu svertochnoi neironnoi seti VGG-16. Vestnik Yuzhno-Uralskogo gosudarstvennogo universiteta. Seriya: Kompyuternye tekhnologii, upravlenie, radioelektronika. 2022. № 22(3). S. 39−46. (in Russian)
- Nepomnyashchii O.V., Khantimirov A.G., Al-sagir M.M.I., Shabir S. Ispolzovanie svertochnoi neironnoi seti pri analize elektrokardiogramm. Neirokompyutery: razrabotka, primenenie. 2023. T. 25. № 2. S. 58−65. DOI: 10.18127/j19998 554-202302-05. (in Russian)
- Akhmetzyanov K.R., Tur A.I., Kokoulin A.N., Yuzhakov A.A. Optimizatsiya vychislenii neironnoi seti. Vestnik Permskogo natsionalnogo issledovatelskogo politekhnicheskogo universiteta. Elektrotekhnika, informatsionnye tekhnologii, sistemy upravleniya. 2020. № 36. S. 117−130. (in Russian)
- Andriyanov N.A., Papakostas Dzh. Optimizatsiya svertochnykh setei s pomoshchyu kvantizatsii i OpenVINO pri raspoznavanii snimkov bagazha. Cb. trudov po materialam VIII Mezhdunar. konf. i molodezhnoi shkoly "Informatsionnye tekhnologii i nanotekhnologii (ITNT-2022). Samara. 2022. S. 33052. (in Russian)
- Guo J.-M., Yang J.-S., Seshathiri S., Wu H.-W. A Light-Weight CNN for Object Detection with Sparse Model and Knowledge Distillation. Electronics 2022. 11. 575. DOI: 10.3390/electronics11040575.
- Vasilev K.K., Dementev V.E. Dvazhdy stokhasticheskaya filtratsiya prostranstvenno neodnorodnykh izobrazhenii. Radiotekhnika i elektronika. 2020. T. 65. № 5. S. 487−494. (in Russian)
- Vasilev K.K., Dementev V.E., Andriyanov N.A. Analiz effektivnosti otsenivaniya izmenyayushchikhsya parametrov dvazhdy stokhasticheskoi modeli. Radiotekhnika. 2015. № 6. S. 12−15. (in Russian)
- URL: https://www.kaggle.com/datasets/meowmeowmeowmeowmeow/gtsrb-german-traffic-sign (data obrashcheniya 12.06.2023)
- Ren S., He K., Girshick R., Sun J. Faster R-CNN: Towards real-time object detection with region proposal networks. Proc. 29th Conf on Neural Information Processing Systems (NeurIPS). 2015. V. 1. P. 91−99.