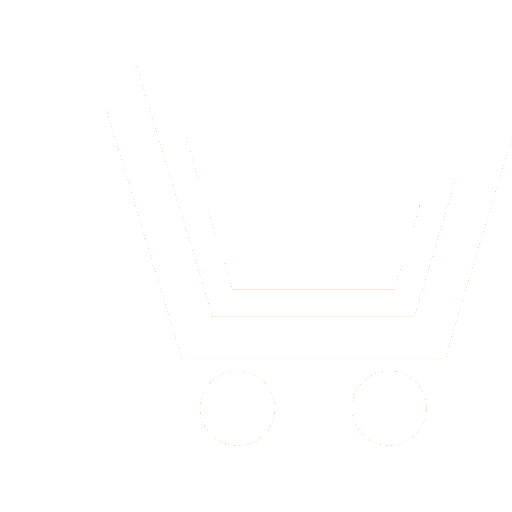
S.A. Korchagin1
1 Financial University under the Government of the Russian Federation (Moscow, Russia)
1 sakorchagin@fa.ru
To determine the quality and composition of nanocomposites, as well as the class to which the material belongs, laborious analysis is required. The creation of a computer vision system for the classification of nanocomposites can significantly simplify this process, reduce time and reduce costs for processing images of the topography of material surfaces.
Goal – develop a computer vision system that allows automatic classification of nanocomposites based on the topography images of the surface of materials.
A computer vision system has been developed that allows automatic classification of nanocomposites with high accuracy. A machine learning model is proposed, which showed the best results for this task, in comparison with known models.
The developed computer vision system can be used in new generations of microscopes that allow automatic classification of nanocomposites.
Korchagin S.A. Computer vision system for automatic classification of nanocomposites. Information-measuring and Control Systems. 2023. V. 21. № 5. P. 16−26. DOI: https://doi.org/10.18127/j20700814-202305-03 (in Russian)
- Ayed E.B. et al Vinyltriethoxysilane-functionalized starch nanocrystals as Pickering stabilizer in emulsion polymerization of acrylic monomers. Application in nanocomposites and pressure-sensitive adhesives. Journal of Colloid and Interface Science. 2020. T. 578. P. 533−546.
- Bharadwaz A., Jayasuriya A.C. Recent trends in the application of widely used natural and synthetic polymer nanocomposites in bone tissue regeneration. Materials Science and Engineering. 2020. T. 110. P. 110698.
- Korchagin S.A., Terin D.V. Metod modelirovaniya dielektricheskoi pronitsaemosti anizotropnogo ierarkhicheski postroennogo nanokompozita s periodicheskoi strukturoi. Pisma v Zhurnal tekhnicheskoi fiziki. 2021. T. 47. № 16. S. 3−5. (in Russian)
- Milosev I., Kapun B. He corrosion resistance of Nitinol alloy in simulate physiological solutionsn. Mater. Sci. Eng. 2012. V. 32. P. 1087−1090.
- Othman N.H., Ismail M.C., Mustapha M., Sallih N., Kee K.E., Jaal R.A. Graphene-based polymer nanocomposites as barrier coatings for corrosion protection. Progress in Organic Coatings. 2019. V. 135. P. 82−99.
- Kausar A. Corrosion prevention prospects of polymeric nanocomposites: A review. Journal of Plastic Film & Sheeting. 2019. V. 35 (2). P. 181−202.
- Liu H., Jian R., Chen H., Tian X., Sun C., Zhu J., Wang C. Application of biodegradable and biocompatible nanocomposites in electronics: Current status and future directions. Nanomaterials. 2019. V. 9(7). P. 950.
- Han L., Cui S., Yu H.Y., Song M., Zhang H., Grishkewich N., Tam K.M. Self-healable conductive nanocellulose nanocomposites for biocompatible electronic skin sensor systems. ACS Applied Materials & Interfaces. 2019. V. 11(47). P. 44642−44651.
- Danilyuk I.R. i dr. Granulometricheskii analiz nanokompozitnykh plenok po izobrazheniyam atomno-silovoi mikroskopii. Novoe v Magnetizme i Magnitnykh Materialakh. 2021. S. 73−75. (in Russian)
- Sarmanova O.E. i dr. Metod y mashinnogo obucheniya v reshen i i zadachimonitoringa vyvedeniya teranosticheskikhfluorestsentnykh nanokompozitov iz organizma. Matematicheskie metody raspoznavaniya obrazov. 2017. T. 18. № 1. S. 160−161. (in Russian)
- Liu B., Vu-Bac N., Rabczuk T. A stochastic multiscale method for the prediction of the thermal conductivity of Polymer nanocomposites through hybrid machine learning algorithms. Composite Structures. 2021. T. 273. S. 114269.
- Shen Z.H., Bao Z.W., Cheng X.X., Li B.W., Liu H.X., Shen Y., Nan C.W. Designing polymer nanocomposites with high energy density using machine learning. npj Computational Materials. 2021. V. 7. № 1. P. 1−9.
- Liu B., Vu-Bac N., Rabczuk T. A stochastic multiscale method for the prediction of the thermal conductivity of Polymer nanocomposites through hybrid machine learning algorithms. Composite Structures. 2021. V. 273. P. 114269.
- Najjar I.M. R., Sadoun A.M., Alsoruji G.S., Abd Elaziz M., Wagih A. Predicting the mechanical properties of Cu–Al2O3 nanocomposites using machine learning and finite element simulation of indentation experiments. Ceramics International. 2022. V. 48. № 6. P. 7748−7758.
- Qiao C., Li D., Guo Y., Liu C., Jiang T., Dai Q., Li D. Evaluation and development of deep neural networks for image super-resolution in optical microscopy. Nature Methods. 2021. V. 18. № 2. P. 194−202.
- Bostan E., Heckel R., Chen M., Kellman M., Waller L. Deep phase decoder: self-calibrating phase microscopy with an untrained deep neural network. Optica. 2020. V. 7. № 6. P. 559−562.
- Dong Y., Li M., Li J. Image retrieval based on improved Canny edge detection algorithm. Proceedings International Conference on Mechatronic Sciences, Electric Engineering and Computer (MEC). IEEE. 2013. S. 1453−1457.
- Proskurin A.V. Kategorizatsiya izobrazhenii na osnove samoorganizuyushcheisya neironnoi seti. Reshetnevskie chteniya. 2014. T. 2. № 18. S. 274−276. (in Russian)
- Chary V.S.K. et al. Artificial Special Visual Geometry Group-16 (VGG) Learning Model for Analysing Accuracy and Precision of SARS-COV-2 Forecasting. International Conference on Computer Communication and Informatics (ICCCI). IEEE. 2023. S. 1−5.
- Wu Z., Shen C., Van Den Hengel A. Wider or deeper: Revisiting the resnet model for visual recognition. Pattern Recognition. 2019. T. 90. S. 119−133.
- Recht B. et al. Do imagenet classifiers generalize to imagenet?. International conference on machine learning. PMLR. 2019. S. 5389−5400.
- Zhu Y., Newsam S. Densenet for dense flow. IEEE international conference on image processing (ICIP). 2017. S. 790−794.
- Koonce B., Koonce B. EfficientNet. Convolutional Neural Networks with Swift for Tensorflow: Image Recognition and Dataset Categorization. 2021. S. 109−123.
- Zhang Y., Ruan K., Gu J. Flexible sandwich‐structured electromagnetic interference shielding nanocomposite films with excellent thermal conductivities. Small. 2021. T. 17. № 42. S. 2101951.
- Bradski G. The openCV library. Dr. Dobb's Journal: Software Tools for the Professional Programmer. 2000. T. 25. № 11. S. 120−123.
- Bagaev I.I. Analiz ponyatii neironnaya set i svertochnaya neironnaya set, obuchenie svertochnoi neiroseti pri pomoshchi modulya tensorflow. Matematicheskoe i programmnoe obespechenie sistem v promyshlennoi i sotsialnoi sferakh. 2020. T. 8. № 1. S. 15−22. (in Russian)
- Jia Y. et al. Caffe: Convolutional architecture for fast feature embedding. Proceedings of the 22nd ACM international conference on Multimedia. 2014. S. 675−678.
- Chaton T. et al. Torch-Points3D: A modular multi-task framework for reproducible deep learning on 3D point clouds. IEEE International Conference on 3D Vision (3DV). 2020. S. 1−10.
- Bergstra J. et al. Theano: Deep learning on gpus with python. NIPS 2011. BigLearning Workshop. Granada. Spain. Granada: Citeseer. 2011. T. 3.
- Andriyanov N.A., Andriyanov D.A. O vazhnosti augmentatsii dannykh pri mashinnom obuchenii v zadachakh obrabotki izobrazhenii v usloviyakh defitsita dannykh. Informatsionnye tekhnologii i nanotekhnologii (ITNT-2020): sb. tr. po materialam VI Mezhdunar. konf. i molodezh. shk. (g. Samara, 26−29 maya): v 4 t. Tek. 2020. (in Russian)
- Zhao B. et al. Achieving wideband microwave absorption properties in PVDF nanocomposite foams with an ultra-low MWCNT content by introducing a microcellular structure. Journal of Materials Chemistry C. 2020. T. 8. № 1. S. 58−70.
- Demir-Kavuk O. et al. Prediction using step-wise L1, L2 regularization and feature selection for small data sets with large number of features //BMC bioinformatics. 2011. T. 12. S. 1−10.
- Rolnick D., Kording K. Reverse-engineering deep relu networks. International Conference on Machine Learning. PMLR. 2020. S. 8178−8187.
- Wang F. et al. Additive margin softmax for face verification. IEEE Signal Processing Letters. 2018. T. 25. № 7. S. 926−930.
- Newell N. et al. Biomechanics of the human intervertebral disc: a review of testing techniques and results. Journal of the mechanical behavior of biomedical materials. 2017. T. 69. S. 420−434.