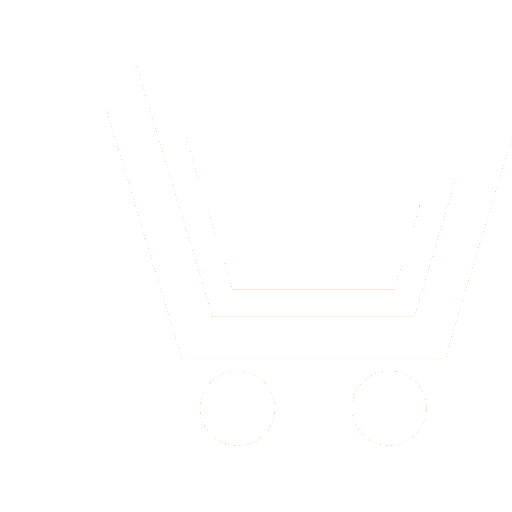
G. V. Rybina1
1 Federal State Budgetary Educational Institution of Higher Vocational Education National
Research Nuclear University MEPhI (Moscow, Russia)
Today, the scope of dynamic intelligent systems and, in particular, dynamic integrated expert systems have broadened significantly. Thus, automation of work of experts and knowledge engineers by software tools of knowledge base construction is of great importance. There are many research works related to automated acquisition, presentation, and processing of temporal knowledge (i.e., knowledge that uses time as an entity) within a development environment that integrates the capabilities of expert systems (formalisms of knowledge representation and processing) with tools for text mining and natural language processing together with tools for data
mining and temporal knowledge discovery in databases. However, analysis of modern tools for knowledge base construction in dynamic intelligent systems has shown that even such powerful systems as G2 (Gensym Corp., US) lack special tools of automated knowledge acquisition from basic knowledge sources (experts, natural language texts, databases). Various methods and tools that address problems of temporal knowledge acquisition from NL-texts are not focused on temporal knowledge base construction in dynamic intelligent systems.
Temporal data is arbitrary data explicitly or implicitly associated with certain dates or time intervals; temporal databases are databases that store temporal data taking into account specific nature of time and the variability of data in time. Methods of acquisition of such data are quite specialized as well. Classical DBMS can also manage temporal databases. However, the developers themselves have to provide the processing of time.
Thus, despite the presence of a significant number of methods and tools of acquiring temporal entities and dependencies in the context of Text Mining and Data Mining technologies, the problems of acquisition of temporal knowledge for constructing temporal knowledge bases in dynamic intelligent systems, and particularly in dynamic integrated expert systems, are poorly addressed. We have devoted some research works to enhancing the problem-oriented methodology for integrated expert system construction and have developed an IDE – AT-TECHNOLOGY that supports prototyping processes of applied integrated expert systems throughout their life cycle.
Our experience in the development of dynamic integrated expert systems has shown that for a number of criteria (such as knowledge representation models, reasoning tools, object-oriented design support, etc.) AT-TECHNOLOGY is comparable to G2 (Gensym corp., US) – leading software platform for real-time expert systems. Considering the built-in subsystem of outer world simulation, AT-TECHNOLOGY even goes ahead of G2. In addition, AT-TECHNOLOGY implements the original combined method of knowledge acquisition (CMKA) automating acquisition process for unreliable and temporal knowledge from various knowledge sources. Together with tools for outer world simulation AT-TECHNOLOGY significantly increases the efficiency of knowledge base construction in dynamic integrated expert systems of various architectures. This paper analyses the results of automated construction of knowledge bases in dynamic integrated expert systems based on the so-called temporal version of the CMKA.
Rybina G.V. Dynamic integrated expert systems: technology of automated acquisition, representation and processing of temporal knowledge. Information-measuring and Control Systems. 2018. V. 16. № 7. P. 20–31. DOI: https://doi.org/10.18127/j20700814-201807-03 (in Russian)
- Rybina G.V. Teoriya i tekhnologiya postroeniya integrirovannyh ehkspertnyh sistem. Monografiya. M.: Nauchtekhlitizdat. 2008. 482 s. (in Russian).
- Rybina G.V. Intellektual'nye sistemy: ot A do YA. Seriya monografij v 3 knigah. Kniga 2. Intellektual'nye dialogovye sistemy. Dinamicheskie intellektual'nye sistemy. M.: Nauchtekhlitizdat. 2015. 160 c. (in Russian).
- Rybina G.V., Mozgachev A.V. Realizaciya temporal'nogo vyvoda v dinamicheskih integrirovannyh ehkspertnyh sistemah. Iskusstvennyj intellekt i prinyatie reshenij. 2014. № 1. S. 34–45 (in Russian).
- Rybina G.V. Kombinirovannyj metod priobreteniya znanij dlya postroeniya baz znanij integrirovannyh ehkspertnyh sistem. Pribory i sistemy. Upravlenie, kontrol', diagnostika. 2011. № 8. S. 19–41 (in Russian).
- Rybina G.V., Danyakin I.D. Combined Method of Automated Temporal Information Acquisition for Development of Knowledge Bases of Intelligent Systems. Proceedings of the 2017 2nd International Conference on Knowledge Engineering and Applications, 2017. London: IEEE. P. 117–123.
- Allen J.F. Maintaining knowledge about temporal intervals. Communications of the Association for Computing Machinery. 1983. V. 22. P. 832–843.
- Osipov G.S. Dinamicheskie intellektual'nye sistemy. Iskusstvennyj intellekt i prinyatie reshenij. 2008. № 1. S. 47–54 (in Russian).
- Zaboleeva-Zotova A.V., Dmitriev A.S., Orlova Yu.A., Rozaliev V.L. Processing of Spatial and Temporal Information in the Text. World Applied Sciences Journal (WASJ). 2013. V. 24. Spec. Is. 24: Information Technologies in Modern Industry, Education & Society (in Russian).
- Pan E. Learning Temporal Information from Text. Encyclopedia of Data Warehousing and Mining, Second Edition, Montclair State University, USA, 2009. P. 1146–1149.
- Schilder F. Temporal Relations in English and German Narrative Discourse. University of Edinburgh. College of Science and Engineering. School of Informatics. 1997. 216 p.
- Yoshikawa K., Riedel S., Asahara M., Y. Matsumoto Y. Jointly Identifying Temporal Relations with Markov Logic. In Proceedings of the Joint Conference of the 47th Annual Meeting of the ACL and the 4th International Joint Conference on Natural Language Processing of the AFNLP. Suntec, Singapore. 2009. P. 405–413.
- Pustejovsky J., Castano J., Ingria R., Sauri R., Gauzauskas R., Setzer A., Katz G. TimeML: Robust Specification of Event and Temporal Expression in Text. In Proceedings of New Directions in Question Answering. 2003. P. 28–34.
- Aggarwal C. C., Zhai C. Mining Text Data. Springer. 2012. 535 p.
- Kaufmann M., Manjili A., Vagenas P., Fischer P., Kossmann D., Faerber F., May N. Timeline Index: A Unified Data Structure for Processing Queries on Temporal Data in SAP HANA. In: SIGMOD. 2013.
- Wan Ishak, Wan Hussain, Ku-Mahamud, Ku Ruhana, Md Norwawi, Norita. Mining temporal reservoir data using sliding window technique. CiiT International Journal of Data Mining and Knowledge Engineering. 2011. № 3(8). P. 473–478.
- Tzacheva A.A., Bagavathi A., Ganesan P.D. MR – Random Forest Algorithm for Distributed Action Rules Discovery. In International Journal of Data Mining & Knowledge Management Process (IJDKP). 2016. V. 6. № 5. P. 15–30.
- Efimenko I.V. Semantika vremeni: modeli, metody i algoritmy identifikacii v sistemah avtomaticheskoj obrabotki estestvennogo yazyka //Vestnik Moskovskogo gosudarstvennogo oblastnogo universiteta. Ser. Lingvistika. 2007. № 2 (in Russian).
- Arutyunova N.D., Yanko T.E. Logicheskij analiz yazyka: Yazyk i vremya. Otv. red. N.D. Arutyunova, T.E. Yanko. M.: Indrik. 1997 (in Russian).