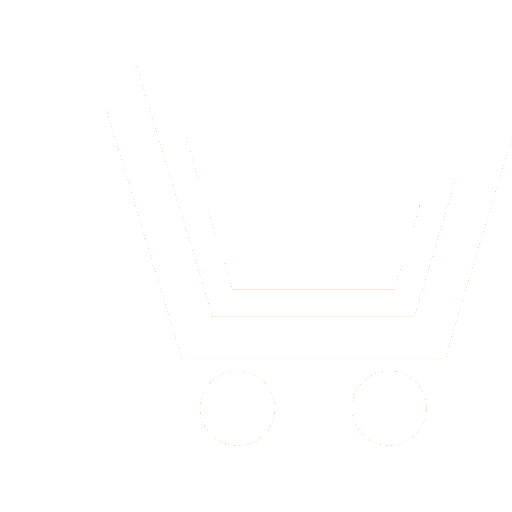
Andrey V. Avramov¹, Viktor N. Nadtochii²
1, 2 MESC «Zhukovsky–Gagarin Air Force Academy» (Voronezh, Russia)
1 andry_a@inbox.ru
The aim of the work is to describe the methodology for a priori classification of objects by classes or types in the language of information signs in the multi-sensor environment of the aircraft onboard equipment complex. The relevance of the work is caused by the need to group heterogeneous features in a multi-sensor environment that describe certain objects or their groups by classes or types. In this case, a rational division of the entire feature space into a minimum sufficient number of areas describing classes/types of objects and allowing to achieve high reliability of their recognition should be ensured. The content of the technique is as follows. Description of objects in the language of information signs is based on the presence of a priori estimated values of the parameters of the trajectory and signal signs of objects. The set of values of the attributes of a certain object, obtained from the entire set of OBE information sensors used, is represented as a multidimensional vector. It can be used to describe a unique portrait of a given object. The set of incompatible combinations of feature values allows us to describe the set of different portraits of objects.
The procedure for forming a sample of the parameters of feature vectors belonging to certain classes of objects is the division of the entire feature space into a number of feature groups that describe objects with similar properties. Such a division is carried out on the basis of determining the correlations between the grouped features within the classes, as well as assessing the correlation of the spread of the centers of objects grouped by feature classes relative to the generalized center of the area of the distribution space of all features. The above mathematical relations allow calculating these correlations and determine the conditions for weighted intra- and intergroup distribution of the values of the attributes. The minimum errors in grouping the values of features within classes are determined on the basis of a rational choice of the number of object classes and the calculation of the best average values of the grouped features of these classes. In turn, the average values of grouped features of object classes and conditional correlation matrices of errors in grouping feature values within a class represent the statistical characteristics of these classes.
The criterion for the best grouping of features of objects by classes / types is the rule that provides the best grouping of features around their average values and the separation of the average values of different classes among themselves. This criterion determines the best partitioning of the feature space into classes and allows you to find the number of groups (classes) of partitioning features, the minimum required to ensure high-quality object recognition. The described technique in a multi-sensor environment provides a partition of the feature space into a minimum sufficient number of classes / types, and also allows you to obtain statistical characteristics of features grouped by classes. It has shown its worth and should find its application in solving problems of reliable recognition of observed objects in the multi-sensor environment of the onboard equipment complex of aircraft.
- Informatsionno-izmeritelnye i upravlyayushchie sistemy radioelektronnye sistemy i kompleksy. Monografiya. Pod red. V.S. Verby. M.: Radiotekhnika. 2020. 490 s. (In Russian).
- Merkulov V.I., Mikheev V.A., Lipatov A.A., Chernov V.S. Osobennosti integratsii i kompleksnoi obrabotki informatsii v sistemakh situatsionnoi osvedomlennosti vozdushnogo bazirovaniya. Uspekhi sovremennoi radioelektroniki. 2016. № 6. S. 3−21. (In Russian).
- Abaturov V.A., Vasilev O.V., Efimov V.A., Makaev V.E. Matematicheskie modeli radiolokatsionnykh signalov, otrazhennykh ot vozdushnykh tselei raznykh klassov. Radiotekhnika. 2006. № 7. S. 28−33. (In Russian).
- Radiolokatsionnye sistemy mnogofunktsionalnykh samoletov. T. 1. RLS – informatsionnaya osnova boevykh deistvii mnogofunktsionalnykh samoletov. Sistemy i algoritmy pervichnoi obrabotki radiolokatsionnykh signalov. Pod red. A.I. Kanashchenkova i V.I. Merkulova. M.: Radiotekhnika. 2006. 656 s. (In Russian).
- Chernykh M.M., Vasilev O.V., Bogdanov A.V., Savelev A.N., Makaev V.E. Eksperimentalnye issledovaniya informatsionnykh svoistv kogerentnykh radiolokatsionnykh signalov. Radiotekhnika. 2000. № 3. S. 47−54. (In Russian).
- Shirman Ya.D., Gorshkov S.A., Leshchenko S.P., Bratchenko G.D., Orlenko V.M. Metody radiolokatsionnogo raspoznavaniya i ikh modelirovanie. V sb.: Radiolokatsionnoe raspoznavanie i metody matematicheskogo modelirovaniya. M.: Radiotekhnika. 2000. (In Russian).
- Duda R., KHart P. Raspoznavanie obrazov i analiz stsen. Per. s angl. G.G. Vainshtena i A.M. Vaskovskogo. Pod red. V.L. Stefanyuka. M.: Mir. 1976. 512 s. (In Russian).
- Gonsales R., Vuds R. Tsifrovaya obrabotka izobrazhenii. M.: Tekhnosfera. 2005. 1072 s. (In Russian).
- Merkulov V.I., Chernov V.S., Semiletov I.G. Kompleksnaya obrabotka informatsii v mnogodatchikovykh integrirovannykh sistemakh vozdushnogo bazirovaniya. Uspekhi sovremennoi radioelektroniki. 2016. № 3. S. 3−16. (In Russian).
- Venttsel E.S. Teoriya veroyatnostei: Uchebnik dlya vuzov. Izd. 7-e, ster. M.: Vysshaya shkola. 2001. 575 s. (In Russian).
- Korn G., Korn T. Spravochnik po matematike (dlya nauchnykh rabotnikov i inzhenerov). M.: Nauka. 1973. (In Russian).