350 rub
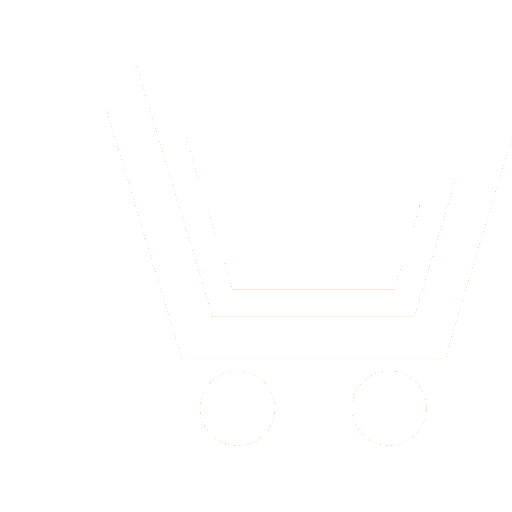
Journal Information-measuring and Control Systems №11 for 2011 г.
Article in number:
Acoustic Event Identification System in Smart Space
Authors:
I. S. Kipyatkova
Abstract:
This paper presents the acoustic event identification system for detection of emergency situations in living room. Because human activity often reproduces a lot of acoustic events, identification or classification of events may help with its detection.
For event detection hidden Markov models were used in the system. A database for model training was developed and it consists of 12 types of acoustic events. About 100 audio files were recorded for each event and total amount of audio files in the database is 1200.
Evaluation of acoustic event identification system work was implemented in two stages. In first stage a user was modeling different events in predefinition locations of a room and in second stage a user might move arbitrary. During the experiments 1226 audio files, which were used for evaluation of the system work, were recorded. Experiment results have shown that 10 types of events were recognized with accuracy more than 90% and other events were recognized with lesser accuracy. Such error rate is connected with the difficulty of detection low-energy acoustic events with high-energy noise overlap.
Pages: 27-30
References
- Gerhard, D., Audio Signal Classification: History and Current Techniques // Technical Report TR-CS 2003-07. 2003.
- Eronen, A.J., Peltonen, V. T., Tuomi, J.T., Klapuri, A. P., Fagerlund, S., Sorsa, T., Lorho, G., and Huopaniemi, J., Audio-based context recognition // IEEE Transactions on Audio, Speech, and Language Processing. 2006. V. 14 № 1. P. 321-329.
- Mesaros A., Heittola, T., Eronen, A., Virtanen, T., Aсoustic event detection in real life recordings // In Proc. European Signal Processing Conference. 2010. P. 1267-1271.
- Peltonen, V. T. K., Eronen, A. J., Parviainen, M. P., and Klapuri, A. P., Recognition of everyday auditory scenes: Potentials, latencies and cues // in In Proc. 110th Audio Eng. Soc. Convention. Hall. 2001.
- Ma, L., Milner, B., and Smith, D., Acoustic environment classification // ACM Trans. Speech Lang. Process. 2006. V. 3.
№ 2.
P. 1-22. - Chu, S., Narayanan, S., Kuo, C.-C. J., Environmental sound recognition with time-frequency audio features // IEEE Trans. on Audio, Speech and Language Process. 2009. V. 17. № 6. P. 1142-1158.
- Heittola, T., Mesaros, A., Eronen, A., Virtanen, T., Audio context recognition using audio event histograms // 18th European Signal Processing Conference (EUSIPCO-2010). 2010. P. 1272-1276.
- Кипяткова И. С. Комплекс программных средств обработки и распознавания разговорной русской речи // Информационно-управляющие системы. 2011. Т. 53. № 4. С. 53-59.
- Кипяткова И. С., Карпов А. А.Аналитический обзор систем распознавания русской речи с большим словарем // Труды СПИИРАН. Вып. 12. СПб.: Наука. 2010. С. 7-20.
- Ронжин Ал. Л., Ронжин Ан. Л.Система аудиовизуального мониторинга участников совещания в интеллектуальном зале // Доклады ТУСУРа. 2011. № 1 (22). Ч. 1. С. 153-157.