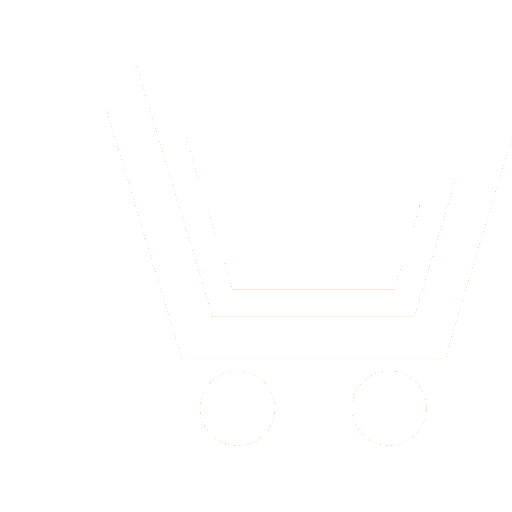
V.N. Gridin1, V.E. Sinitsyn2, V.I. Solodovnikov3, M.I. Truphanov4, N.N. Yakhno5, I.A. Evdokimov6
1–6 Design Information Technologies Center Russian Academy of Sciences (Odintsovo, Moscow oblast, Russia)
1–6 info@ditc.ras.ru
The purpose of this publication is to develop a method, algorithms and present the obtained research results in the field of analysis and recognition of properties of classes of small-sized objects in medical images and similar in visual properties to other nearby tissues of biological objects for localization and determination of properties of the entorhinal cortex of the brain. The relevance of the research is determined by the complexity of finding and measuring the parameters of the entorhinal cortex due to its features in magnetic resonance tomographic images: this cerebral cortex is located next to the hippocampus and is a small in size, complex in shape with no clear boundaries for segmentation with adjacent structures, an object with a curvilinear shape several pixels wide and several tens of pixels long with weakly defined edges. An analysis of publications on this problem revealed the absence of specific solutions, while general solutions based on well-known publicly available and paid products require adaptation for the analysis of the entorhinal cortex. The result of the work is the developed method and algorithms for detecting and determining the parameters of the entorhinal cortex and adjacent tissues for constructing a classifier for separating patients with initial cognitive impairments and healthy people. To solve the problem, iterative use of neural networks based on the yolo architecture was applied with subsequent refinement of the properties of classes that determine a patient with cognitive impairments or a healthy individual. The practical value of the obtained results is that the presented solution will be further used to implement studies at the initial stages of the formation of cognitive impairments when processing magnetic resonance imaging data of patients and healthy people, which will ensure the detection of cognitive impairments at an early stage.
Gridin V.N., Sinitsyn V.E., Solodovnikov V.I., Truphanov M.I., Yakhno N.N., Evdokimov I.A. A method for calculating the properties of the entorhinal cortex in magnetic resonance imaging of the brain. Highly Available Systems. 2024. V. 20. № 4. P. 64−77. DOI: https://doi.org/10.18127/j20729472-202404-07 (in Russian)
- A High-Resolution Model of the Human Entorhinal Cortex in the Big Brain – Use Case for Machine Learning and 3D Analyses Sabrina Behuet, Sebastian Bludau, Olga Kedo, Christian Schiffer, Timo Dickscheid, Andrea Brandstetter1, Philippe Massicotte, Mona Omidyeganeh, Alan Evans, and Katrin Amunts.
- Hippocampal subfield and amygdala nuclei volumes in schizophrenia patients with a history of violence. 2020. European Archives of Psychiatry and Clinical Neuroscience 270(10) DOI:10.1007/s00406-020-01098-y
- The Alzheimer's disease neuroimaging initiative (ADNI): MRI methods. April 2008. V. 27. Iss. 4. P. 685–691.
- Tracey I., Leknes S.G. (2013). Hippocampus and Entorhinal Complex: Functional Imaging. In: Gebhart G.F., Schmidt R.F. (eds). Encyclopedia of Pain. Springer, Berlin, Heidelberg. https://doi.org/10.1007/978-3-642-28753-4_1763
- Segmentation of the mouse hippocampal formation in magnetic resonance images. Kay Richards, Charles Watson, Rachel F. Buckley. NeuroImage. October 2011. V. 58. Iss. 3. P. 732–740.
- Transfer Learning for Alzheimer's Disease Diagnosis from MRI Slices: A Comparative Study of Deep Learning Models. Georgiana Ingrid Stoleru, Adrian Iftene Science. Procedia Computer. 2023. V. 225. P. 2614–2623.
- Diwan T., Anirudh G. & Tembhurne J.V. Object detection using YOLO: challenges, architectural successors, datasets and applications. Multimed Tools Appl, 2023. № 82. R. 9243–9275. https://doi.org/10.1007/s11042-022-13644-y
- Gridin V.N., Perepelov V.A., Panishhev V.S., Trufanov M.I., Yaxno N.N. Algoritm vy`chisleniya parametrov gippokampa pri diagnostike bolezni al`czgejmera. Biomedicinskaya radioe`lektronika. 2019. T. 22. № 7. S. 5–15.
- Gridin V.N., Trufanov M.I., Solodovnikov V.I., Panishhev V.S., Sinicyn V.E., Yaxno N.N. Avtomaticheskij analiz kolichestvenny`x xarakteristik gippokampa pri magnitno-rezonansnoj tomografii golovnogo mozga dlya diagnostiki vozmozhnoj bolezni al`czgejmera (obzor literatury` i rezul`taty` sobstvenny`x issledovanij). Radiologiya – praktika. 2017. № 6 (66). S. 41–59.
- Gridin V.N., Trufanov M.I., Yaxno N.N., Sinicyn V.E. Metod dekompozicii biologicheskix ob``ektov na MRT izobrazheniyax pri sxozhem fone. Informacionny`e texnologii i vy`chislitel`ny`e sistemy`. 2024. № 2. S. 37–51.
- Gridin V.N., Gazov A.I., Novikov I.A., Solodovnikov V.I., Trufanov M.I. Nemasshtabiruemy`e funkcii v kachestve modelej granicy biologicheskogo ob``ekta. Sistemy` vy`sokoj dostupnosti. 2021. T. 17. № 4. S. 66–75.