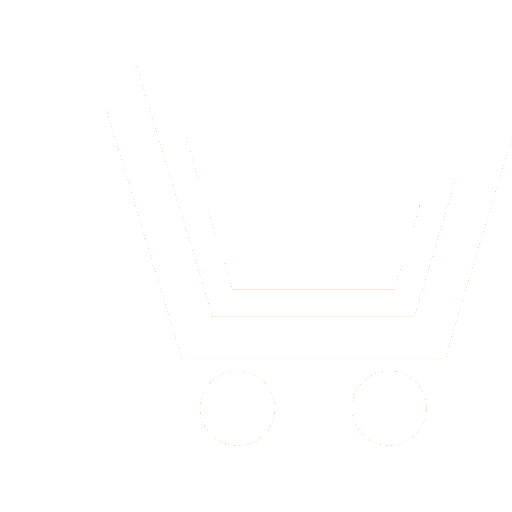
V.I. Budzko1, D.A. Melikov2, V.G. Belenkov3
1–3 FRC CSC RAS (Moscow, Russia)
1 National Research Nuclear University MEPhI (Moscow, Russia)
2 Financial University under the Government of the Russian Federation (Moscow, Russia)
1 vbudzko@ipiran.ru, 2 mda-17@yandex.ru; 3 vbelenkov@ipiran.ru
The current stage of development of Russian society is characterized by the digital transformation of all its spheres, including economics, science, healthcare, education, culture, etc. One of the directions of such transformation is the widespread use of artificial intelligence (AI) technologies (AIT). AIT have a significant potential to transform society and people's lives – from trade and healthcare to transport and cybersecurity, as well as the environment. At the same time, AIT entail risks of managing information security (IS), which can negatively affect individuals, groups, organizations, sectors of the economy and society as a whole. The article analyzes the types of attacks on automated systems (AS) using multilayer neural networks (MNS). It identifies the characteristic features of training and testing of MNS that affect the AI risks for these AS. The main ways of parrying the AIT threats of the AS caused by the use of MNS in them are considered. A generalized classification of attacks on AS using vulnerabilities specific to MNS is presented. The differences between the AI risks of software components using and not using MNS are determined. The main ways to reduce the negative consequences of attacks using vulnerabilities specific to the MNS are described. The main types of attacks on the AS using vulnerabilities specific to the MNS, as well as the main ways to reduce the negative consequences of these attacks, are identified.
Budzko V.I., Belenkov V.G., Korolev V.I., Melikov D.A. About the features of managing the security of automated systems that include neural network technologies. Highly Available Systems. 2023. V. 19. № 4. P. 5−20. DOI: https://doi.org/ 10.18127/j20729472-202304-01 (in Russian)
- Budzko V.I., Korolyov V.I., Mel'nikov D.A., Belenkov V.G. Osobennosti obespecheniya informacionnoj bezopasnosti avtomatizirovannyh sistem, kotorye ispol'zuyut tekhnologii nejronnyh setej. Sistemy vysokoj dostupnosti. 2023. T. 19. № 3. S. 5–17. DOI: https://doi.org/ 10.18127/j20729472-202303-01
- Nitika Khurana, Sudip Mittal, Aritran Piplai, Anupam Joshi. Preventing Poisoning Attacks On AI Based Threat Intelligence Systems. IEEE International Workshop on Machine Learning for Signal Processing (MLSP) 2019: 1-6.
- Blaine Nelson, Marco Barreno, Fuching Jack Chi, Anthony D. Joseph, Benjamin I.P. Rubinstein, Udam Saini, Charles A. Sutton, J. Doug Tygar, Kai Xia. Exploiting Machine Learning to Subvert Your Spam Filter. Usenix Workshop on Large-Scale Exploits and Emergent Threats (LEET). 2008.
- Matthew Jagielski, Alina Oprea, Battista Biggio, Chang Liu, Cristina Nita-Rotaru, Bo Li. Manipulating Machine Learning: Poisoning Attacks and Countermeasures for Regression Learning. IEEE Symposium on Security and Privacy. 2018: 19–35.
- Nathalie Baracaldo, Bryant Chen, Heiko Ludwig, Jaehoon Amir Safavi. Mitigating Poisoning Attacks on Machine Learning Models: A Data Provenance Based Approach. AISec@CCS 2017: 103–110.
- Yao Cheng, Cheng-Kang Chu, Hsiao-Ying Lin, Marius Lombard-Platet, David Naccache. Keyed Non-parametric Hypothesis Tests. International Conference on Network and System Security (NSS). 2019: 632–645.
- Sanghyun Hong, Varun Chandrasekaran, Yigitcan Kaya, Tudor Dumitras, Nicolas Papernot. On the Effectiveness of Mitigating Data Poisoning Attacks with Gradient Shaping. arXiv: 2002.11497v2.
- Tran, Brandon, Jerry Li, and Aleksander Madry. Spectral signatures in backdoor attacks. In Advances in Neural Information Processing Systems. 2018. Р. 8000–8010.
- Chen, Bryant, Wilka Carvalho, Nathalie Baracaldo, Heiko Ludwig, Benjamin Edwards, Taesung Lee, Ian Molloy and Biplav Srivastava. Detecting backdoor attacks on deep neural networks by activation clustering. Artificial Intelligence Safety Workshop @ AAAI. 2019.
- Yuntao Liu, Yang Xie, Ankur Srivastava. Neural Trojans. 2017 IEEE International Conference on Computer Design (ICCD). Boston, MA. 2017. Р. 45–48, doi: 10.1109/ICCD.2017.16
- Liu, Kang, Brendan Dolan-Gavitt and Siddharth Garg. Fine-pruning: Defending against backdooring attacks on deep neural networks. In International Symposium on Research in Attacks, Intrusions and Defenses. 2018. Р. 273–294. Springer, Cham, 2018.
- Wang, Bolun, Yuanshun Yao, Shawn Shan, Huiying Li, Bimal Viswanath, Haitao Zheng and Ben Y. Zhao. Neural cleanse: Identifying and mitigating backdoor attacks in neural networks. In 2019 IEEE Symposium on Security and Privacy (SP). Р. 707–723.
- Wenbo Guo, Lun Wang, Xinyu Xing, Min Du, and Dawn Song. Tabor: A highly accurate approach to inspecting and restoring trojan backdoors in ai systems. arXiv preprint arXiv:1908.01763v2 (2019).
- Yansong Gao, Chang Xu, Derui Wang, Shiping Chen, Damith C.Ranasinghe, Surya Nepal. STRIP: A Defence Against Trojan Attacks on Deep Neural Networks. 2019 Annual Computer Security Applications Conference (ACSAC '19).
- Sakshi Udeshi, Shanshan Peng, Gerald Woo, Lionell Loh, Louth Rawshan and Sudipta Chattopadhyay. Model Agnostic Defence against Backdoor Attacks in Machine Learning. arXiv preprint arXiv:1908.02203v2 (2019).
- Chou Edward, Florian Tramèr, Giancarlo Pellegrino. sentiNet: Detecting Localized Universal Attack Against Deep Learning Systems / The 3rd Deep Learning and Security Workshop. 2020.
- Bao Gia Doan, Ehsan Abbasnejad, and Damith Ranasinghe. Februus: Input Purification Defense Against Trojan Attacks on Deep Neural Network Systems. The 36th Annual Computer Security Applications Conference (ACSAC). 2020.
- Xiaojun Xu, Qi Wang, Huichen Li, Nikita Borisov, Carl A Gunter and Bo Li. Detecting AI Trojans Using Meta Neural Analysis. IEEE S&P. 2021.
- Huili Chen, Cheng Fu, Jishen Zhao, Farinaz Koushanfar. DeepInspect: A Black-box Trojan Detection and Mitigation Framework for Deep Neural Networks. IJCAI 2019: 4658–4664.
- Soheil Kolouri, Aniruddha Saha, Hamed Pirsiavash, Heiko Hoffmann, Universal Litmus Patterns. Revealing Backdoor Attacks in CNNs. CVPR. 2020.
- Gintare Karolina Dziugaite, Zoubin Ghahramani, Daniel M. Roy. A study of the effect of JPG compression on adversarial images. International Society for Bayesian Analysis (ISBA 2016) World Meeting.
- Hossein Hosseini, Yize Chen, Sreeram Kannan, Baosen Zhang, Radha Poovendran. Blocking Transferability of Adversarial Examples in Black-Box Learning Systems. ArXiv 2017.
- Nicolas Papernot, Patrick McDaniel, Xi Wu, Somesh Jha and Ananthram Swami. Distillation as a defense to adversarial perturbations against deep neural networks. IEEE Symposium S&P. 2016
- Shixin Tian, Guolei Yang, Ying Cai. Detecting Adversarial Examples Through Image Transformation. AAAI. 2018.
- Dongyu Meng, Hao Chen. MagNet: A Two Pronged Defense against adversarial examples. ACM Conference on Computer and Communications Security (CCS). 2017.
- Faiq Khalid, Hassan Ali, Hammad Tariq, Muhammad Abdullah Hanif, Semeen Rehman, Rehan Ahmed, Muhammad Shafique: QuSecNets: Quantization-based Defense Mechanism for Securing Deep Neural Network against Adversarial Attacks. IEEE 25th International Symposium on On-Line Testing and Robust System Design (IOLTS). 2019.
- Jialong Zhang, Zhongshu Gu, Jiyong Jang, Hui Wu, Marc Ph. Stoecklin, Heqing Huang, Ian Molloy. Protecting Intellectual Property of Deep Neural Networks with Watermarking. ASIACCS'18.
- Manish Kesarwani, Bhaskar Mukhoty, Vijay Arya, Sameep Mehta. Model Extraction Warning in MLaaS Paradigm. ACSAC 2018.
- Huadi Zheng, Qingqing Ye, Haibo Hu, Chengfang Fang, Jie Shi. BDPL: A Boundary Differentially Private Layer Against Machine Learning Model Extraction Attacks. ESORICS 2019.
- Mika Juuti, Sebastian Szyller, Samuel Marchal, N. Asokan. PRADA: Protecting Against DNN Model Stealing Attacks. 2019 IEEE European Symposium on Security and Privacy (EuroS&P).
- Florian Tramèr, Fan Zhang, Ari Juels, Michael K. Reiter, Thomas Ristenpart. Stealing Machine Learning Models via Prediction APIs. Usenix Security 2016.
- Lukas, Nils, Yuxuan Zhang, and Florian Kerschbaum. «Deep Neural Network Fingerprinting by Conferrable Adversarial Examples». arXiv preprint arXiv: 1912.00888v3, (2020).
- Cao, Xiaoyu, Jinyuan Jia, and Neil Zhenqiang Gong. IPGuard: Protecting the Intellectual Property of Deep Neural Networks via Fingerprinting the Classification Boundary. ACM ASIA Conference on Computer and Communications Security (ASIACCS). 2021.
- Nicolas Papernot, Martin Abadi, Ulfar Erlingsson, Ian Goodfellow, Kunal Talwar. Semisupervised knowledge transfer for deep learning from private training data. ICLR 2017.
- Martín Abadi, Andy Chu, Ian Goodfellow, H. Brendan McMahan, Ilya Mironov, Kunal Talwar, Li Zhang. Deep learning with differential privacy. Proceedings of the 2016 ACM CCS, 2016.
- Milad Nasr, Reza Shokri, and Amir Houmansadr. Machine learning with membership privacy using adversarial regularization. Proceedings of the 2018 ACM CCS. 2018.
- Jinyuan Jia, Ahmed Salem, Michael Backes, Yang Zhang, Neil Zhenqiang Gong. MemGuard: Defending against Black-Box Membership Inference Attacks via Adversarial Examples. ACM CCS. 2019.
- Ziqi Yang, Bin Shao, Bohan Xuan, Ee-Chien Chang, and Fan Zhang. Defending Model Inversion and Membership Inference Attacks via Prediction Purification. ArXiv, arXiv:2005.03915v2. 2020.