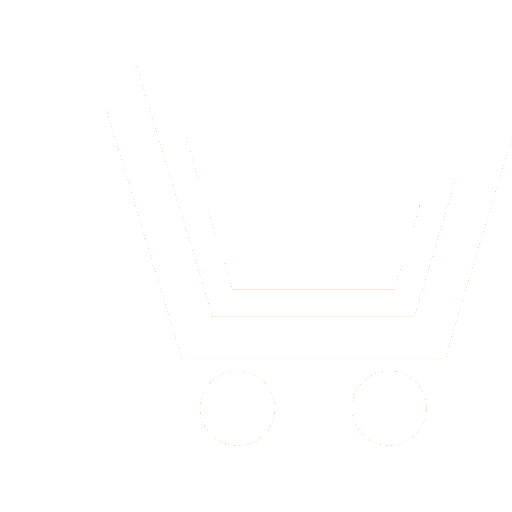
S.S. Volkov1, D.A. Devyatkin2, I.V. Sochenkov3, A.O. Shelmanov4
1–3 Federal Research Center “Computer Science and Control” of the Russian Academy of Sciences (Moscow, Russia)
1 Peoples’ Friendship University of Russia (Moscow, Russia)
4 Artificial Intelligence Research Institute (AIRI) (Moscow, Russia)
Labeled message datasets are required to train tools to detect hatred messages in social media, and the collection of those datasets is a laborious task. Such sets are widely available in the public domain for English and Arabic but can be hardly found for the Russian and other languages. The article presents an automated approach to creating labeled sets of religious hatred messages from social media. This approach combines focus crawling of social network messages and active learning approaches. Crawling is a step-by-step procedure that uses active learning methods to correct message labeling and to train the classifier used to filter irrelevant texts. The developed approach makes it possible to simultaneously form a multilingual corpus of religious hatred messages and train a classifier to identify them.
Volkov S.S., Devyatkin D.A., Sochenkov I.V., Shelmanov A.O. Automated approach to collect religious hatred messages from social media. Highly Available Systems. 2023. V. 19. № 2. P. 70−80. DOI: https://doi.org/ 10.18127/j20729472-202204-06 (in Russian)
- Alfina I., Mulia R., Fanany M.I., Ekanata Y. Hate speech detection in the Indonesian language: A dataset and preliminary study. 2017 International Conference on Advanced Computer Science and Information Systems (ICACSIS). IEEE. 2017. P. 233–238.
- Pant K., Dadu T. Cross-lingual inductive transfer to detect offensive language. arXiv preprint arXiv:2007.03771. 2020.
- Stappen L., Brunn F., Schuller B. Cross-lingual zero-and few-shot hate speech detection utilising frozen transformer language models and AXEL. arXiv preprint arXiv:2004.13850. 2020.
- Kauranen M. Cross-lingual Comment Toxicity Classification. LU-CS-EX. 2020.
- Balayn A., Yang J., Szlavik Z., Bozzon A. Automatic identification of harmful, aggressive, abusive, and offensive language on the web: a survey of technical biases informed by psychology literature. ACM Transactions on Social Computing (TSC). 2021. V. 4. № 3. p. 1–56. https://doi.org/10.1145/3479158.
- Cowan G., Khatchadourian D. Empathy, ways of knowing, and interdependence as mediators of gender differences in attitudes toward hate speech and freedom of speech. Psychology of women quarterly. 2003. V. 27. № 4. P. 300–308.
- Ren S., Deng Y., He K., Che W. Generating natural language adversarial examples through probability weighted word saliency. Proceedings of the 57th annual meeting of the association for computational linguistics. 2019. P. 1085–1097.
- Li L., Ma R., Guo Q., Xue X., Qiu X. Bert-attack: Adversarial attack against bert using bert. arXiv preprint arXiv:2004.09984. 2020.
- Jin D., Jin Z., Zhou J.T., Szolovits P. Is bert really robust? a strong baseline for natural language attack on text classification and entailment. Proceedings of the AAAI conference on artificial intelligence. 2020. V. 34. № 05. P. 8018–8025.
- Garg S., Ramakrishnan G. Bae: Bert-based adversarial examples for text classification. arXiv preprint arXiv:2004.01970. 2020.
- Devlin J., Chang M.W., Lee, K., Toutanova, K. Pre-training of deep bidirectional transformers for language understanding. arXiv preprint arXiv:1810.04805. 2018.
- Ryzhova A. et al. Training Multilingual and Adversarial Attack-Robust Models for Hate Detection on Social Media. Procedia Computer Science. 2022. V. 213. P. 196–202.
- Charitidis P. et al. Towards countering hate speech against journalists on social media. Online Social Networks and Media. 2020. V. 17. P. 100071.
- Luu S.T., Nguyen K.V., Nguyen N.L.T. A large-scale dataset for hate speech detection on vietnamese social media texts. Advances and Trends in Artificial Intelligence. Artificial Intelligence Practices: 34th International Conference on Industrial, Engineering and Other Applications of Applied Intelligent Systems, IEA/AIE 2021, Kuala Lumpur, Malaysia, July 26–29, 2021, Proceedings, Part I 34. Springer International Publishing, 2021. P. 415–426.
- Liu Y. et al. Roberta: A robustly optimized bert pretraining approach. arXiv preprint arXiv:1907.11692. 2019.
- Podolskiy A. et al. Revisiting mahalanobis distance for transformer-based out-of-domain detection. Proceedings of the AAAI Conference on Artificial Intelligence. 2021. V. 35. № 15. P. 13675–13682.
- Hu Y., Khan L. Uncertainty-aware reliable text classification. Proceedings of the 27th ACM SIGKDD Conference on Knowledge Discovery & Data Mining. 2021. P. 628–636.
- Lewis D.D., Catlett J. Heterogeneous uncertainty sampling for supervised learning. Machine learning proceedings 1994. Morgan Kaufmann. 1994. P. 148–156.
- Gal Y., Ghahramani Z. Dropout as a bayesian approximation: Representing model uncertainty in deep learning. International conference on machine learning. PMLR, 2016. P. 1050–1059.
- Gissin D., Shalev-Shwartz S. Discriminative active learning. arXiv preprint arXiv:1907.06347. 2019.
- Vazhentsev A. et al. Uncertainty Estimation of Transformer Predictions for Misclassification Detection. Proceedings of the 60th Annual Meeting of the Association for Computational Linguistics (V. 1: Long Papers). 2022. P. 8237–8252.
- Hersovici M. et al. The shark-search algorithm. An application: tailored web site mapping. Computer Networks and ISDN Systems. 1998. Т. 30. № 1–7. P. 317–326.
- Devyatkin D.A. et al. Intelligent analysis of manifestations of verbal aggressiveness in network community texts. Scientific and Technical Information Processing. 2014. V. 41. P. 377–389.
- Manikandan N.K. et al. Focused Web Crawler For Retrieving Relevant Contents. Turkish Journal of Computer and Mathematics Education (TURCOMAT). 2021. V. 12. № 10. P. 2025–2028.
- Kumar S. et al. Decision tree Thompson sampling for mining hidden populations through attributed search. Social Network Analysis and Mining. 2022. V. 12. № 1. P. 1–21.
- Conneau A. et al. Unsupervised cross-lingual representation learning at scale. arXiv preprint arXiv:1911.02116. 2019.
- Devyatkin D., Smirnov I., Ananyeva M., Kobozeva M., Chepovskiy A., Solovyev F. 2017, July. Exploring linguistic features for extremist texts detection (on the material of Russian-speaking illegal texts). In 2017 IEEE International Conference on Intelligence and Security Informatics (ISI) (P. 188–190). IEEE.
- Набор сообщений на русском языке с признаками ненависти. https://www.kaggle.com/datasets/blackmoon/russian-language-toxic-comments. Доступ: 10 апреля 2023.
- Mandl T., Modha S., Majumder P., Patel D., Dave M., Mandlia C., Patel A. Overview of the hasoc track at fire 2019: Hate speech and offensive content identification in indo-european languages. Proceedings of the 11th forum for information retrieval evaluation. 2019. P. 14–17.
- El-Yaniv R. On the Foundations of Noise-free Selective Classification. Journal of Machine Learning Research. 2010. V. 11. № 5.
- Xin J., Tang R., Yu Y., Lin J. The art of abstention: Selective prediction and error regularization for natural language processing. Proceedings of the 59th Annual Meeting of the Association for Computational Linguistics and the 11th International Joint Conference on Natural Language Processing (V. 1: Long Papers). 2021. P. 1040–1051.