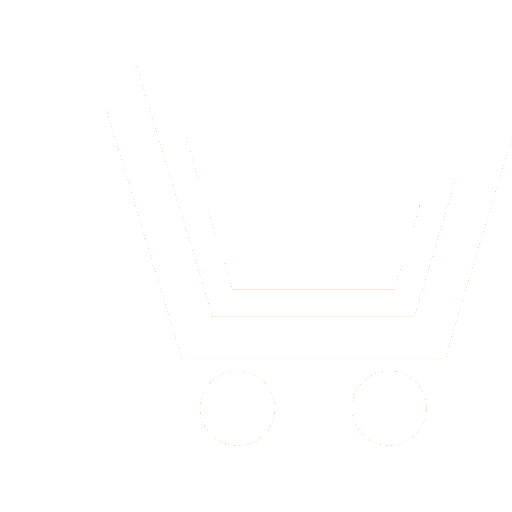
I.P. Urmanov1, S.P. Korolev2, A.N. Kamaev3
1–3 Computing Center FEB RAS (Khabarovsk, Russia)
The article discusses the analysis of images obtained from video cameras shooting in a wide range, including visible and near-infrared wavelengths. The problem of identification of thermal anomalies in the images of volcanoes obtained with such cameras is solved. The introduction contains a brief review of methods and approaches to the solution of the problem. Section 1 is devoted to the description of the developed algorithm of thermal anomalies detection. At the beginning of the section, the procedure of data sampling preparation is described and the details of thermal anomalies display on the images are shown. Then a description of all the stages of the algorithm is given, including finding the centers of potential anomalies, calculating the area and determining the features of the anomalies with further classification of the obtained data into classes of "thermal" anomaly and "non-thermal" anomaly. In Section 2, the description of the computer system for automated image analysis is given. At the beginning, the implementation of the developed algorithm in the form of a console application is shown. Then its integration into the computer system for the automated analysis of images of the system of continuous video observation of volcanoes of Kamchatka is considered. Also is given a description of all capabilities of the obtained system, a scheme of its work and examples of developed interfaces. In the conclusion of the article summarized the results of the work and the prospects for further research.
Urmanov I.P., Korolev S.P., Kamaev A.N. A computer system for searching and evaluating thermal anomalies in volcano images. Highly Available Systems. 2021. V. 17. № 4. P. 55−65. DOI: https://doi.org/10.18127/j20729472-202104-04 (in Russian)
- Sorokin A., Korolev S., Romanova I., Girina O., Urmanov I. The Kamchatka Volcano Video Monitoring System. Proceedings of 2016 6th International Workshop on Computer Science and Engineering, WCSE 2016. Tokyo, Japan. 17–19 June. 2016. P. 734–737.
- Harris A.J.L., Ripepe M., Hughes E.A. Detailed analysis of particle launch velocities, size distributions and gas densities during normal explosions at Stromboli. Journal of Volcanology and Geothermal Research. 231–232 (2012). P. 109–131.
- Diefenbach A.K., Bull K. F., Wessels R., Mcgimsey R.G. Photogrammetric monitoring of lava dome growth during the 2009 eruption of Redoubt Volcano. Journal of Volcanology and Geothermal Research. 259 (2013). P. 308–316. DOI: 10.1016/j.jvolgeores.2011.12.009
- Walter T.R., Legrand D., Delgado G.H., Reyes G., Arámbula R. Volcanic eruption monitoring by thermal image correlation: pixel offsets show episodic dome growth of the Colima volcano. JGR: Solid Earth. 118 (2013), № 4. P. 1408–1419. DOI: 10.1002/jgrb.50066
- Webb E., Varley N., Pyle D., Mather T. Thermal imaging and analysis of short-lived Vulcanian explosions at Volcán de Colima, Mexico. Journal of Volcanology and Geothermal Research. 278–279 (2014). P. 132–145. DOI: 10.1016/j.jvolgeores.2014.03.013
- Patrick M.R., Orr T.R., Antolik L., Lee R.L., Kamibayashi K.P. Continuous monitoring of Hawaiian volcanoes with thermal cameras. Journal of Applied Volcanology. 3 (2014). No. 1. DOI: 10.1186/2191-5040-3-1
- Bertucco L., Coltelli M., Nunnari G., Occhipinti L. Cellular neural networks for real-time monitoring of volcanic activity. Computers & Geosciences. 25 (1999). No. 2. P. 101–117.
- Honda K., Nagai M. Real-time volcano activity mapping using ground-based digital imagery. ISPRS Journal of Photogrammetry and Remote Sensing. 57 (2002). P. 159–168.
- Major J.J., Dzurisin D., Schilling S., Poland M. Monitoring lava-dome growth during the 2004–2008 Mount St. Helens, Washington, eruption using oblique terrestrial photography. Earth and Planetary Science Letters. 286 (2009). No. 1–2. P. 243–254.
- Arason P., Petersen G.N., Bjornsson H. Observations of the altitude of the volcanic plume during the eruption of Eyjafjallajökull, April– May 2010. Earth System Science Data. 3 (2011). No. 1. P. 9–17. DOI: 10.5194/essd-3-9-2011
- Bjornsson H., Magnusson S., Arason P., Petersen G.N. Velocities in the plume of the 2010 Eyjafjallajökull eruption. JGR: Atmospheres. 118 (2013). No. 20. P. 11698-11711. DOI: 10.1002/jgrd.50876
- Sorokin A., Korolev S., Romanova I., Girina O., Urmanov I. The Kamchatka Volcano Video Monitoring System. Proceedings of 2016 6th International Workshop on Computer Science and Engineering, WCSE 2016, Tokyo, Japan, 17–19 June, 2016. P. 734–737.
- Blackett M. An Overview of Infrared Remote Sensing of Volcanic Activity. Journal of Imaging. 3(2017). No. 2. P. 13. DOI: 10.3390/jimaging3020013
- Andò B., Pecora E. An Advanced Video-based System for Monitoring Active Volcanoes. Computers & Geosciences. 32 (2006). No. 1. P. 85-91
- Spampinato L., Calvari S., Oppenheimer C., Boschi E. Volcano Surveillance Using Infrared Cameras. Earth-Science Reviews. 106 (2011). P. 63–91
- Olivo-Marin J.C. Extraction of Spots in Biological Images Using Multiscale Products. Pattern Recognition. 35 (2002). No. 9. P. 1989–1996.
- Wang F., Liu Y., Wu Ch., Chen X., Zeng K. Spot Image Ablated by Femtosecond Laser Segmentation and Feature Clustering After Dimension Reduction Reconstruction. Optik. 164 (2018). P. 488–497.
- Mel'nikov D.V., Manevich A.G., Girina O.A. Kolichestvennye harakteristiki aktivnosti vulkanov Kamchatki po dannym veb-kamer./ Tez. Dokl. XVIII regional'noj konf., posvyashchennoj Dnyu vulkanologa. Petropavlovsk-Kamchatskij: IViS DVO RAN, 2015. S. 92–94.
- Gudfellou YA., Bendzhio I., Kurvill' A. Glubokoe obuchenie. Per. s ang. A.A. Slinkina. 2-e izd., ispr. M.: DMK Press. 2018. 652 s.: cv. il.
- Cho J., Lee K., Shin E., Choy G., Do S. How much data is needed to train a medical image deep learning system to achieve necessary high accuracy? arXiv e-print. 2016. arXiv: 1511.06348
- Hénaff O. J., Srinivas A., De Fauw J., Razavi A., Doersch C., Eslami S.M.A., van den Oord A. Data-Efficient Image Recognition with Contrastive Predictive Coding. arXiv e-prints. 2019. arXiv: 1905.09272
- Lowe D.G. Distinctive Image Features from Scale-invariant Keypoints. Intern. J. of Computer Vision. 60 (2004). No. 2. P. 91–110.
- Michael E. Tipping, Christopher M. Bishop Mixtures of Probabilistic Principal Component Analyzers. Neural Computation. 11 (1999) No. 2. P. 443–482. doi: https://doi.org/10.1162/089976699300016728
- Shmilovici A. Support Vector Machines. Data Mining and Knowledge Discovery Handbook. Boston, MA, USA: Springer. 2009. P. 257–276.
- Kamaev A.N., Korolev S. P., Sorokin A.A., Urmanov I.P. Detection of Thermal Anomalies in the Images of Volcanoes Taken at Night. Journal of Computer and Systems Sciences International. 59 (2020). No. 1. P. 119–128. DOI: 10.1134/S106423071906008X
- Sorokin A.A., Korolev S.P., Malkovsky S.I. The Signal automated information system: Research and operational monitoring of dangerous natural phenomena in the Russian Far East. Sovremennye Problemy Distantsionnogo Zondirovaniya Zemli iz Kosmosa. 16(2019). No. 3. P. 238-248. DOI: 10.21046/2070-7401-2019-16-3-238-248
- Sorokin A.A., Makogonov S.V., Korolev S.P. The Information Infrastructure for Collective Scientific Work in the Far East of Russia. Scientific and Technical Information Processing. 44 (2017). No. 4. R. 302–304.