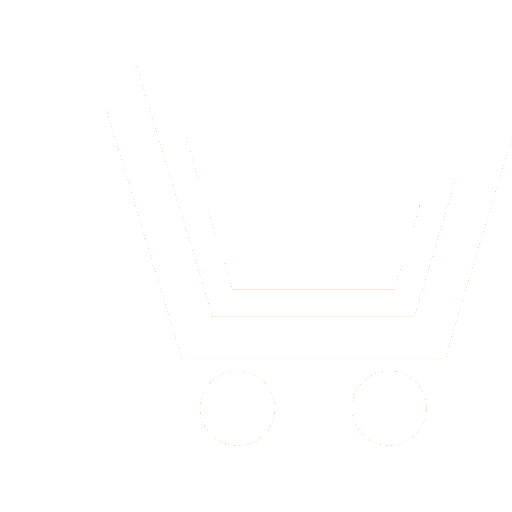
V.Ye. Marley¹, A.N. Terekhov², Yu.A. Gatchin³, V.I. Milushkov4, N.N. Limansky5
1,4,5 Admiral Makarov State University of Maritime and Inland Shipping (Saint Petersburg, Russia)
2 Saint Petersburg State University (Saint Petersburg, Russia)
3 St. Petersburg National Research University of Information Technologies, Mechanics and Optics (St. Petersburg, Russia) (Saint Petersburg, Russia)
¹vmarley@mail.ru, ²ant@tercom.ru, ³gatchin1952@mail.ru, 4info@sohoware.ru, 5info@sohoware.ru
Currently, there is a lack of accessible and open data in Russia that can be used for training neural networks necessary for accurate maritime traffic prediction. This creates challenges in developing effective models that account for the specific characteristics of Russian maritime traffic and forces the use of foreign or synthetic data, which reduces the accuracy and reliability of predictions.
Objective. The development of a method for maritime traffic prediction based on the analysis of historical Automatic Identification System (AIS) data using a Long Short-Term Memory (LSTM) network.
The effectiveness of using an LSTM network for analyzing historical AIS data has been evaluated, significantly improving the accuracy of vessel movement predictions and ensuring maritime operational safety. A model was developed that has demonstrated practical value in the absence of real-time data.
The proposed approach enhances maritime traffic management, reducing the risk of accidents and ensuring navigational safety. The model can be used for predicting vessel routes and integrating new features, such as vessel condition monitoring and collision avoidance.
Marley V.E., Terekhov A.N., Gatchin Yu.A., Milushkov V.I., Limansky N.N. Forecasting the state of shipping traffic based on time series data from an automatic identification system using a long short-term memory network. Dynamics of complex systems. 2024.
V. 18. № 4. P. 28−40. DOI: 10.18127/j19997493-202404-03 (in Russian).
- Milushkov V.I., Limanskij N.N. Integraciya gibridnogo polukontroliruemogo i kontrastnogo obucheniya dlya avtomaticheskoj klassifikacii defektov v proizvodstvennyh dannyh: povyshenie tochnosti kontrolya kachestva produkcii. Perspektivy nauki. 2024. Vyp. 6 (177) (in Russian).
- Milushkov V.I., Limanskij N.N. Obnaruzhenie anomalij v robotizirovannyh sistemah: sravnitel'nyj analiz ConvLSTM s mekhanizmom vnimaniya i tradicionnyh podhodov. Perspektivy nauki. 2024. Vyp. 6 (177) (in Russian).
- Dang X.K., Tran T.D., Tran M.H., Pham T.D.A. Inland Waterway Transport in Vietnam: Strategies to Improve Transportation Efficiency during COVID-19 Pandemic. IOP Conference Series: Earth and Environmental Science. 2022. V. 1072. № 1. P. 12006–12012. https://doi.org/10.1088/1755-1315/1072/1/012006
- The International Maritime Organization. AIS Transponders. Retrieved from http://www.imo.org/en/OurWork/Safety/Navigation/Pages/ AIS.aspx. 2022.
- Gudevada, V., Apon, A., & Ding, J. Data Quality Considerations for Big Data and Machine Learning: Going Beyond Data Cleaning and Transformations. International Journal on Advances in Software. 2017. V. 10. № 1. P. 1–20.
- Kocenko A.A., Goryachkin B.S., Bazanova A.G., Marushchenko A.V., Varlamov O.O. Model' opisaniya mivarnyh setej v formate dvudol'nyh i trekhdol'nyh orientirovannyh grafov dlya prinyatiya reshenij i obrabotki informacii v mashinostroitel'nom II. Dinamika slozhnyh sistem – XXI vek. 2024. № 1. S. 5–17 (in Russian).
- Panov M.A., Ishchenko E.A. Sovremennye kompleksy monitoringa i opoveshcheniya o sobytiyah: obespechenie optimal'nogo ispol'zovaniya resursov i funkcionirovaniya informacionnyh sistem i processov. Dinamika slozhnyh sistem – XXI vek. 2024. № 1. S. 18–31 (in Russian).
- Semenyuk S.S., Kablukov A.V., Grigorov A.S. Opredelenie mestopolozheniya istochnikov radioizluchenij raznostno-dal'nomernym metodom na osnove roevogo intellekta. Dinamika slozhnyh sistem – XXI vek. 2024. № 1. S. 51–57 (in Russian).
- Liu D., Rong H., Guedes Soares C. Shipping route modelling of AIS maritime traffic data at the approach to ports. Ocean Engineering. 2023. V. 289. Article 115868. https://doi.org/10.1016/j.oceaneng.2023.115868
- Wang X., Wang W. Study on the maritime trade pattern and freight index in the post-epidemic era: Evidence based on dry bulk market Automatic Identification System (AIS) data. The Asian Journal of Shipping and Logistics. 2024. V. 40. № 1. P. 1–10. https://doi.org/10.1016/j.ajsl.2023.09.002
- Falcon R., Abielmona R., Blasch E. Behavioral Learning of Vessel Types with Fuzzy Rough Decision Trees. FUSION 2014 – 17th International Conference on Information Fusion. Salamanca, Spain. 2014. P. 1–8.
- Mascaro S., Korb K., Nicholson A. Learning Abnormal Vessel Behaviour from AIS Data with Bayesian Networks at Two Time Scales. Tracks a Journal of Artists Writings. 2010. P. 1–34. https://doi.org/10.1016/j.ijar.2013.03.012
- Evgeniou T., Pontil M. Support Vector Machines: Theory and Applications. Conference: Machine Learning and Its Applications, Advanced Lectures. 2001. V. 2049. P. 249–257. https://doi.org/10.1007/3-540-44673-7_12
- Guyon I., Weston J., Barnhill S., Vapnik V. Gene selection for cancer classification using Support Vector Machines. Machine Learning. 2002. V. 46. № 1–3. P. 389–422. https://doi.org/10.1023/A:1012487302797
- Senthil D., Suseendran G. Sequence Mining-Based Support Vector Machine with Decision Tree Approach for Efficient Time Series Data Classification. Advances in Intelligent Systems and Computing. 2020. V. 1016. P. 3–17. https://doi.org/10.1007/978-981-13-9364-8_1
- Chih W.H., Chih C.C., Chih J.L. A Practical Guide to Support Vector Classification. Department of Computer Science, National Taiwan University. Taiwan. 2003.
- Nembrini S., König I.R., Wright M.N. The revival of the Gini importance? Bioinformatics. 2018. V. 34. № 21. P. 3711–3718. https://doi.org/10.1093/bioinformatics/bty373
- Rong H., Teixeira A.P., Guedes Soares C. Ship trajectory uncertainty prediction based on a Gaussian Process model. Ocean Engineering. 2019. V. 182. P. 499–511. https://doi.org/10.1016/j.oceaneng.2019.04.024
- Nguyen D., Fablet R. A Transformer Network With Sparse Augmented Data Representation and Cross Entropy Loss for AIS-Based Vessel Trajectory Prediction. IEEE Access. 2024. V. 12. P. 21596–21609. https://doi.org/10.1109/ACCESS.2024.3349957
- Bao K., Shang D., Wang R., Ma R. AIS big data framework for maritime safety supervision. Proceedings – 2020 International Conference on Robots and Intelligent Systems, ICRIS. 2020. P. 150–153. https://doi.org/10.1109/ICRIS52159.2020.00045
- Wang S., Li Y., Xing H. A novel method for ship trajectory prediction in complex scenarios based on spatio-temporal features extraction of AIS data. Ocean Engineering. 2023. V. 281. Article 114846. https://doi.org/10.1016/j.oceaneng.2023.114846
- Pedrielli G., Xing Y., Peh J. H., Koh K.W., Ng S.H. A real time simulation optimization framework for vessel collision avoidance and the case of Singapore strait. IEEE Transactions on Intelligent Transportation Systems. 2020. V. 21. № 3. P. 1204–1215. https://doi.org/10.1109/TITS.2019.2903824
- Shi W., Hu L., Lin Z., Zhang L., Wu J., Chai W. Short-term motion prediction of floating offshore wind turbine based on muti-input LSTM neural network. Ocean Engineering. 2023. https://doi.org/10.1016/j.oceaneng.2023.114558
- Doğan E. LSTM training set analysis and clustering model development for short-term traffic flow prediction. Neural Computing and Applications. 2021. V. 33. № 17. P. 11175–11188. https://doi.org/10.1007/s00521-020-05564-5
- Park S.H., Kim B., Kang C.M., Chung C.C., Choi J.W. Sequence-to-Sequence Prediction of Vehicle Trajectory via LSTM Encoder-Decoder Architecture. 2018 IEEE Intelligent Vehicles Symposium (IV). 2018. https://doi.org/10.1109/IVS.2018.8500658
- Cho K., Van Merriënboer B., Gulcehre C., Bahdanau D., Bougares F., Schwenk H., Bengio Y. Learning phrase representations using RNN encoder-decoder for statistical machine translation. EMNLP 2014 – 2014 Conference on Empirical Methods in Natural Language Processing, Proceedings of the Conference. 2014. P. 1724–1734. https://doi.org/10.3115/v1/d14-117
- Panchal F.S., Panchal M. Review on Methods of Selecting Number of Hidden Nodes in Artificial Neural Network. International Journal of Computer Science and Mobile Computing. 2014. V. 3. № 11. P. 455–464.
- Wen X., Li W. Time Series Prediction Based on LSTM-Attention-LSTM Model. IEEE Access. 2023. V. 11. P. 48322–48331. https://doi.org/10.1109/ACCESS.2023.3276628