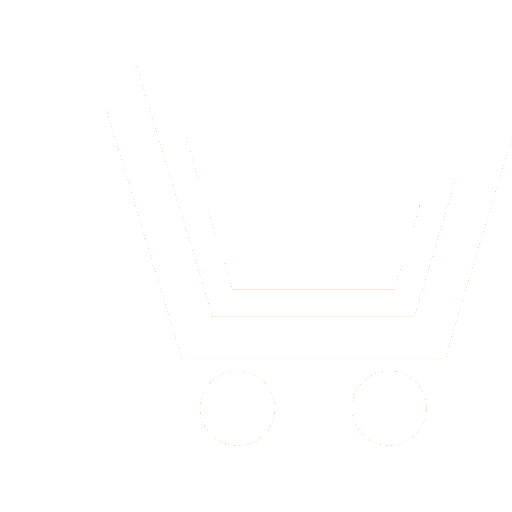
N.C. Vanroye1, V.O. Zacharova2, V.A. Shakhnov3
1–3 Bauman Moscow State Technical University (National Research-Tel University) (Moscow, Russia)
1 nvanroje@mail.ru, 2 valeriyashe@yandex.ru, 3 saluev.evgeniy@gmail.com, 4 shakhnov@iu4.bmstu.ru
Setting the problem. To quickly make timely decisions, it is important to ensure the prompt analysis of data processed in the networks of mobile operators. Mobile operators collect more and more data every day. However, due to the complexity of the network infrastructure, analysis of event metrics requires multidimensional analysis of hierarchy levels in the network topology, as opposed to processing linear events that do not require hierarchical calculation. In this case, it is necessary to aggregate the values of indicators by levels of topology and localization (geographical location – from the atomic cell to the level of the entire network).
The batch processing architecture of current performance management systems is not well suited for real-time event flow processing. In modern systems, information is stored and processed through databases or distributed file systems such as Hadoop. Such systems use an ETL (Extract Transform Load) based approach and correlate data using a batch processing model. It can take several minutes to get results from such systems.
In real-time event flow processing systems, it is more important to process and present more incoming information as quickly as possible. In this case, it is allowed to lose a small part of the number of incoming events. For anomalies detection and operational response, a vision of the full picture of the mobile network is of great value, albeit with some loss in data accuracy, but immediately than an absolutely accurate picture of all indicators of the mobile network, but with a significant delay. To solve this problem, streaming models of space-time aggregation of network indicators are used.
Objective – research of methods and models of streaming evaluation of spatially aggregated performance and security indicators of the mobile operator's network.
This article proposes a performance management approach in that network elements periodically send elementary events as a stream of technical statistics files to network management systems. This data is processed using intelligent batch processing tools to generate deferred KPIs and network quality metrics based on these counters. However, the delayed nature of calculating accumulated statistics is positioned by mobile network operators as a drawback. This necessitates the creation of methods and models for monitoring networks in near-real-time mode.
To implement real-time monitoring scenarios, it is useful to use interactive analytical and streaming models that provide aggregated results with low latency. These reports are important for mobile operators and are numeric counters such as the number of user equipment, the number of switches or various failures, and physical measurements such as user equipment signal strength and the like. In practice, these performance measurements are periodically generated and aggregated into blocks of key indicators. Their collection provides the necessary statistics to network operators, helping them determine the state of the network, for example, overloaded or underused sections of the network, as well as its faulty components.
Vanroye N.C., Zacharova V.O., Shakhnov V.A. Study of methods to ensure information security of wireless networks. Dynamics of complex systems. 2023. V. 17. № 4. P. 12−25. DOI: 10.18127/j19997493-202304-02 (in Russian).
- Murav'ev K.A., Fedorov R.A. Razvitie seti svyazi shestogo pokoleniya. Tekhnologii inzhenernyh i informacionnyh sistem. 2022. № 2.
S. 34–45 (in Russian). - Adamov A.P., Adamova A.A. O novyh uslugah setej sotovoj svyazi i problemah ih vnedreniya v Rossii. Servis: problemy i puti razvitiya v usloviyah rynochnoj ekonomiki. Tezisy dokladov regional'noj nauch.-prakt. konf. 2005. S. 9–10 (in Russian).
- Karpunin A.A., Murav'ev K.A. Metodika ocenki klyuchevyh pokazatelej effektivnosti telekommunikacionnyh sistem. V sb.: Energosberezhenie i effektivnost' v tekhnicheskih sistemah. Materialy IX Mezhdunar. nauch.-tekhn. konf. studentov, molodyh uchenyh i specialistov. 2022. S. 128–130 (in Russian).
- Leonidov V.V., Murav'ev K.A. Podhody k analizu kachestva v telekommunikacionnoj sfere// V sb.: Energosberezhenie i effektivnost' v tekhnicheskih sistemah. Mater. IX Mezhd. nauch.-tekhn. konf. studentov, molodyh uchenyh i specialistov. 2022. S. 137–138 (in Russian).
- Vlasov A.I. Vizual'noe modelirovanie effektivnosti servisov kiberfizicheskih sistem. V sb.: Energosberezhenie i effektivnost' v tekhnicheskih sistemah. Mater. IX Vseros. nauch.-tekhn. konf. s mezhdunar. uchastiem dlya studentov, molodyh uchenyh i specialistov. 2022. S. 26–27 (in Russian).
- Carbone P., Ewen S., Haridi S., Katsifodimos A., Markl V., Tzoumas K. Apache flink: Stream and batch processing in a single engine. IEEE Data Engineering Bulletin. 2015.
- Vlasov A.I., Muraviev K.A., Prudius A.A., Uzenkov D.A. Load balancing in big data processing systems. International Review of Automatic Control. 2019. V. 12. № 1. P. 42–47.
- Vlasov A.I., Kramar' D.N., Cvetkov A.A. Analiz metodov hraneniya i vizual'nyh modelej dannyh pri razrabotke prilozhenij dlya OS ANDROID. Avtomatizaciya. Sovremennye tekhnologii. 2023. T. 77. № 4. S. 181–187 (in Russian).
- Vlasov A.I., Ivanov V.V., Kosolapov I.A. Metody uprezhdayushchego prognozirovaniya sostoyaniya shirokopolosnoj seti svyazi. Programmnye produkty i sistemy. 2011. № 1. S. 3–6 (in Russian).
- Murav'ev K.A., Terekhov V.V. Analiz pokazatelej kachestva sistem peredachi dannyh. Trudy mezhdunarodnogo simpoziuma «Nadezhnost' i kachestvo». 2018. T. 1. S. 128–133 (in Russian).
- Murav'ev K.A., Demin A.A., Karpunin A.A. Voprosy obespecheniya kachestva programmnyh sistem. V sb.: Energosberezhenie i effektivnost' v tekhnicheskih sistemah. Mater. IX Mezhdunar. nauch.-tekhn. konfer. studentov, molodyh uchenyh i specialistov. 2022. S. 41–42 (in Russian).
- Dudko V.G., Verejnov K.D., Vlasov A.I., Timoshkin A.G. Sovremennye metody i sredstva obespecheniya kachestva v usloviyah kompleksnoj avtomatizacii. Voprosy radioelektroniki. 1994. № 1. S. 71–89 (in Russian).
- Murav'ev K.A., ZHalnin V.P., Spirichev I.I., Metelkin A.V., Eremin M.YU. Analiz pokazatelej kachestva besprovodnyh sensornyh setej. Trudy Mezhdunarodnogo simpoziuma «Nadezhnost' i kachestvo». 2021. № 2. S. 142–147 (in Russian).
- Svidetel'stvo o registracii programmy dlya EVM 2022665632. Kompleks prediktivnogo monitoringa raspredelennyh sensornyh setej. K.V. Selivanov, A.I. Vlasov, V.S. Klimachev. 2022 (in Russian).
- Tihvinskij V., Bajkenov A., Soloshenko A. Avtomaticheskoe upravlenie setyami 4G/5G s ispol'zovaniem tekhnologij i algoritmov SON. Pervaya milya. 2019. № 3. S. 80–91 (in Russian).
- Vlasov A.I., Nevzorov I.V. Analiz arhitektur i tekhnologij hranilishch dannyh (obzor). Informacionnye tekhnologii v proektirovanii i proizvodstve. 2021. № 4 (184). S. 13–21 (in Russian).
- Vlasov A.I., Papulin S.YU. Analiz dannyh s ispol'zovaniem gistogrammnoj modeli kombinacii priznakov. Nejrokomp'yutery: razrabotka, primenenie. 2019. T. 21. № 5. S. 18–27 (in Russian).
- Aver'yanihin A.E., Murav'ev K.A. Analiz modelej testirovaniya slozhnyh programmnyh sistem i ih vliyanie na pokazateli kachestva. V sb.: Energosberezhenie i effektivnost' v tekhnicheskih sistemah. Mater. V Mezhdunar. nauchno-tekhnicheskoj konferencii studentov, molodyh uchenyh i specialistov. 2018. S. 313–314 (in Russian).
- Nesterov YU.I., Vlasov A.I., Pershin B.N. Virtual'nyj izmeritel'nyj kompleks. Datchiki i sistemy. 2000. № 4. S. 12–22 (in Russian).
- Vlasov A.I., Koloskov S.V., Pakilev A.E. Nejrosetevye metody i sredstva obnaruzheniya atak na setevom urovne. 2-ya Vseros. nauch.-tekhn. konf. «Nejroinformatika-2000»: sb. nauch. trudov v 2 chastyah. Ser. «Nauchnaya sessiya MIFI-2000». 2000. S. 30–40 (in Russian).
- Vlasov A.I., Zaharov E.R., Zaharova V.O. Nejrosetevaya sistema obnaruzheniya i nejtralizacii udalennogo nesankcionirovannogo vmeshatel'stva v komponenty interneta veshchej. Nejrokomp'yutery: razrabotka, primenenie. 2021. T. 23. № 1. S. 63–80 (in Russian).
- Technical Specification Group Services and System Aspects. Management and orchestration. 5G end to end Key Performance Indicators (KPI) – 3rd Generation Partnership Project (Release 16).
- Marcu O.-C., Costan A., Antoniu G., Perez M.S. Spark versus flink: Understanding performance in big data analytics frameworks. Proc. in Cluster 2016 – The IEEE 2016 International Conference on Cluster Computing, 2016.
- Adamova A.A. Preprocessing i agregaciya iskhodnyh dannyh dlya upravleniya tekhnologichnost'yu v priborostroenii. Trudy Mezhdunarodnogo simpoziuma «Nadezhnost' i kachestvo». 2022. № 2. S. 142–148 (in Russian).
- Vaibhav Pandey, Amarjeet Kaur, Narottam Chand A Review on Data Aggregation Techniques in Wireless Sensor Network. Journal of Electronic and Electrical Engineering. 2010. V. 1. Is. 2.
- Gulisano V., Tudor V., Almgren M., Papatriantafilou M. Bes: Differentially private and distributed event aggregation in advanced metering infrastructures. Proceedings of the 2nd ACM International Workshop on Cyber-Physical System Security. Xi'an, China: ACM. 2016. P. 59–69.
- SHeremet. Rekursivnye mul'timnozhestva i ih prilozheniya. M.: Nauka. 2010. 291 s. (in Russian).
- Abasov R.K., Silla A.A., Vlasov A.I. Formalizaciya konstruktorsko-tekhnologicheskoj informacii s ispol'zovaniem obrabotki mul'timnozhestv. Informacionnye tekhnologii v proektirovanii i proizvodstve. 2017. № 3 (167). S. 34–43 (in Russian).
- Tyler Akidau, Robert Bradshaw, Craig Chambers, Slava Chernyak, Rafael J Fernndez-Moctezuma, Reuven Lax, Sam McVeety, Daniel Mills, Frances Perry, Eric Schmidt et al. The dataflow model: a practical approach to balancing correctness, latency, and cost in massive-scale, unbounded, out-of-order data processing. 2015.
- Kamburugamuve S., Fox G., Leake D., Qiu J. Survey of distributed stream processing for large stream sources. Grids Ucs Indiana Edu, School Inform. Comput., Indiana Univ., Bloomington, IN. USA. Tech. Rep. 2013.
- Vlasov A.I., Kramar' D.N., Cvetkov A.A. Analiz metodov hraneniya i vizual'nyh modelej dannyh pri razrabotke prilozhenij dlya OS ANDROID. Avtomatizaciya. Sovremennye tekhnologii. 2023. T. 77. № 4. S. 181–187 (in Russian).
- Apache spark: Spark streaming programming guide. https://spark.apache. org/docs/latest/streaming-programming-guide.html, 2021.