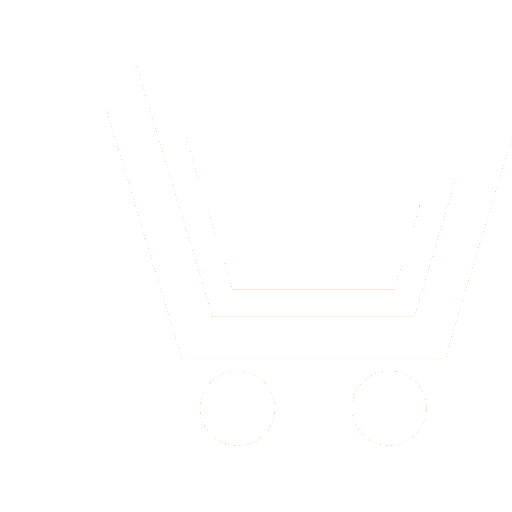
A.V. Shilonosov1, N.V. Sukhanov2
1 VA RVSN (Balashikha, Russia), 2 GosNIIAS (Moscow, Russia)
The article suggests the use of an inverse-adaptive scheme in the management of a complex dynamic object operating under conditions of many non-stationary parameters and nonlinearities. The considered synthesis of regulators using a neural network approach allows us to take into account the specifics of the functioning of the object in conditions of uncertainty
The article deals with the problematic issue of obtaining the desired control of a complex dynamic object, for this purpose, as a rule, it is necessary to obtain a model of the object, however, taking into account the set of non-stationary parameters and nonlinearities, obtaining such a model is a difficult task, even more difficult is to synthesize regulators that take into account the specifics of the functioning of the object in conditions of uncertainty.
The following main factors can be attributed to the main sources of uncertainty in solving control problems: the non-stationarity of the parameters of the control object; the complexity of the formalized description of the object, taking into account the errors of the necessary calculations and measurements; the uncertainty of the operating conditions and the situation; the vagueness of the functioning goals and the need for their correction; the presence of accidental environmental influences, the opposition of the enemy; distortion of incoming input information.
The development of control algorithms for dynamic objects based on traditional mathematical methods, taking into account stability, does not always give the desired result, since the complexity of the algorithms leads to difficulties in their implementation on computer technology, therefore, the paper proposes to improve the quality of control of a complex dynamic object under uncertainty by implementing a control algorithm using neural networks.
The application of an inverse adaptive scheme consisting of two dynamically trained models, a direct neural network model (neural network identifier) and an inverse neural network model (neural network controller) in the control system of a complex dynamic object is proposed. A distinctive feature of the proposed scheme is the use of the output of the direct model of the object and the current changes in the setting function, which are used to train the inverse model. The results obtained during simulation modeling of the operation of the control system with artificial neural networks demonstrate the adaptive properties of the neural network controller in response to a step signal and when changing the characteristics of the control object.
An adaptive control system scheme is proposed, including a direct neural network model (neural network identifier and an inverse model (neural network controller). The results obtained using simulation clearly demonstrate the adaptive properties of a neural network controller when simulating external influences and when changing the dynamic characteristics of the control object.
Shilonosov A.V., Sukhanov N.V. Application of the neural network approach in the control system of a complex dynamic object. Dynamics of complex systems. 2022. V. 16. № 1. P. 46−56. DOI: 10.18127/j19997493-202201-05 (In Russian)
- Makarov I.M., Lohin V.M., Man'ko S.V., Romanov M. P. Sitnikov M. S. Ustojchivost' intellektual'nyh sistem avtomaticheskogo upravleniya. Informacionnye tekhnologii. Prilozhenie. 2013. № 2. S. 1–32 (In Russian).
- Balyukov A.A., Zajcev A.V., Kanushkin S.V. Model' dvizheniya bespilotnogo letatel'nogo apparata s ispol'zovaniem intellektual'nyh tekhnologij. Trudy voenno-nauchnoj konferencii «Robotizaciya Vooruzhennyh Sil Rossijskoj Federacii». M.: 2016. S. 470. S. 198–204 (In Russian).
- Le Hi Fong. Nejrosetevaya sistema desantirovaniya gruzov transportnogo vertoleta v slozhnyh pogodnyh usloviyah: Dis. ... kand. tekhn. nauk: 05.13.01.: M. 2004. 128 s. (In Russian).
- Ejkkhoff P. Osnovy identifikacii sistem upravleniya. M.: Mir. 1975. 680 s. (In Russian).
- Cypkin YA.Z. Osnovy informacionnoj teorii identifikacii. M.: Nauka. 1984. 320 s. (In Russian).
- Paluzo-Hidalgo E., Gonzalez-Diaz R., Gutiérrez-Naranjo MA. Two-hidden-layer feed-forward networks are universal approxi¬mators: A constructive approach. Neural Networks. 2020. V. 7. P. 29–36 (In Russian).
- Hajkin S. Nejronnye seti: polnyj kurs: Per. s angl. Izd. 2-e, ispr. M.: Izdatel'skij dom «Vil'yams». 2016. 1104 s. (In Russian).
- Buyankin V.M. Primenenie iskusstvennoj nejronnoj seti v rezhime identifikacii dinamicheskih parametrov elektrodvigatelya. Vestnik MGTU im. N.E. Baumana. Ser. Priborostroenie. 2006. № 3. S. 25–30 (In Russian).
- Buyankin V. M. Nejroidentifikaciya staticheskih i dinamicheskih harakteristik elektroprivoda. Izv. vuzov. Ser. Mashinostroenie. 2008.
№ 9. S. 43–45 (In Russian). - Buyankin V.M., Pantyuhin D.V. Nejroidentifikaciya staticheskih i dinamicheskih harakteristik asinhronnogo elektrodvigatelya peremennogo toka. Nejrokomp'yutery razrabotka i primenenie. 2009. № 7. S. 69–73 (In Russian).
- Kileel J., Trager M., Bruna J. 2019. On the expressive power of deep polynomial neural networks. In: Wallach H.M., Larochelle H., Beygelzimer A., d’Alché-Buc F., Fox E.B., Garnett R. (Eds.), Advances in Neural Information Processing Systems 32: Annual Conference on Neural Information Processing Systems 2019. NeurIPS 2019. 8–14 December 2019. Vancouver BC. Canada, pp. 10310-10319. URL: http://papers.nips.cc/paper/9219-on-the-expressive-power-of-deep-polynomial-neural-networks. (Data obrashcheniya 21.09.2021)
- D'yakonov V.P., Kruglov V.V. MATLAB 6.5 SP1/7/7 SP1/7 SP2 Simulink 5/6 Instrumenty iskusstvennogo intellekta i bioinformatiki. M.: Solon-press. 2006 (In Russian).
- Osovskij S. Nejronnye seti dlya obrabotki informacii: Per. s pol'sk. I.D. Rudinskogo. Izd. 2-e, izd. pererab. i dop. M.: Goryachaya liniya – Telekom. 2017. 448 s. (In Russian).