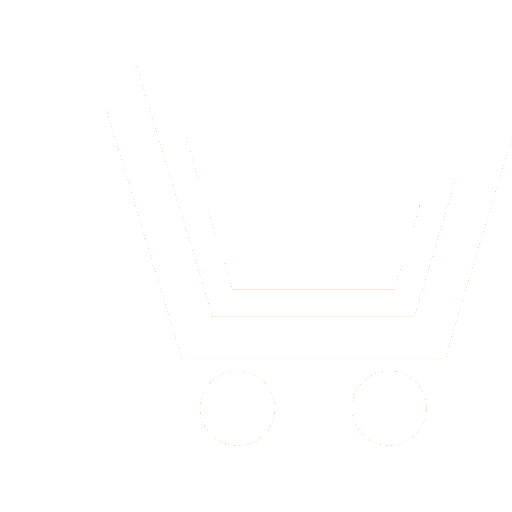
D.V. Stroganov¹, V.M. Chernenky²
1 Moscow State Automobile and Road Technical University (Moscow, Russia)
2 Bauman Moscow State Technical University (Moscow, Russia)
Thus, the formal formulation of the problem of evaluating the effectiveness of search optimization procedures on simulation models of regenerating processes under strict time constraints has been completed. A procedure for parametric tuning of the optimization algorithm has been developed, which sequentially refines the values of the functional specified by the model and redistributes the remaining model regeneration cycles between the investigated values of the controlled parameter.
The problem of maximizing the probability of the correct choice is posed and solved, i.e. selection, according to the results of the simulation experiment on the model of the regenerating process, of the value of the controlled parameter that delivers the true maximum to the investigated functional. Based on the transition to the Lagrangian, the solution to the constrained optimization problem is reduced to an unconstrained optimization problem. Analytical expressions are obtained to assess the optimal distribution of regeneration cycles. It is shown that the simulation model with the included search engine optimization algorithm provides solutions that are quite effective in terms of computational costs.
As a result, a method is proposed for a simple extension of the developed simulation models by including a search optimization algorithm, which makes it possible to move from modeling the system to optimizing its objective function on a given area of controlled parameters.
Stroganov D.V., Chernenky V.M. Evaluation of the efficiency of the problem of choosing the extreme values of the parameters of the parametric family of regenerating processes. Dynamics of complex systems. 2021. T. 15. № 1. DOI: https://doi.org/ 10.18127/j19997493-202101-01 (In Russian) Dynamics of complex systems / Dinamika slozhnykh sistem, V. 15, № 1, 2021, P. 5−12
- Attetkov A.V., Galkin S.V., Zarubin B.C. Metody optimizacii: Uchebnik dlya vuzov. Pod red. B.C. Zarubina, A.P. Krishchenko. Izd. 2-e, stereotip. M.: Izd-vo MGTU im. N.E. Baumana. 2003. 440 s. (In Russian).
- Karpenko A.P. Populyacionnye algoritmy global'noj optimizacii. Obzor novyh i maloizvestnyh algoritmov. Prilozhenie k zhurnalu «Informacionnye tekhnologii». 2012. № 7. S. 1–32 (In Russian).
- Karpov V.E. Metodologicheskie problemy evolyucionnyh vychislenij. Iskusstvennyj intellekt i prinyatie reshenij. 2012. № 4. C. 95–102 (In Russian).
- Matrenin P.V. Opisanie i realizaciya algoritmov roevogo intellekta s ispol'zovaniem sistemnogo podhoda. Programmnaya inzheneriya. 2015. № 3. S. 27–34 (In Russian).
- Kondrat'eva T.N. Prognozirovanie tendencii finansovyh vremennyh ryadov s pomoshch'yu nejronnoj seti LSTM [Elektronnyj resurs]. Vestnik evrazijskoj nauki. 2017. T. 9. № 4(41) (In Russian).
- Krejn M., Lemuan O. Vvedenie v regenerativnyj metod analiza modelej. M.: Nauka. 1982. 104 s. (In Russian).
- Kuznecov H.B. Konstruktivnyj metod postroeniya «iskusstvennyh» momentov regeneracii. Teoriya slozhnyh sistem i metody ih modelirovaniya: Trudy seminara VNIISI. M. 1903. S. 15–22 (In Russian).
- Kuleshov E.L., Babijchuk I.A. Linejnoe prognozirovanie stacionarnyh sluchajnyh processov pri izvestnom i neizvestnom trende. Avtometriya. 2005. T. 41. № 2. S. 23–35 (In Russian).
- Chernen'kij V.M., Chernen'kij M.V. Processno-agregativnye sistemy imitacionnogo modelirovaniya: Ucheb. posobie. M.: Pero. 2018. 160 s. (In Russian).
- Rejzlin V.I. Chislennye metody optimizacii: uchebnoe posobie. Tomsk: Izd-vo Tomskogo gos. un-ta. 2011. 105 s. (In Russian).
- Stroganov V.Yu., Chernen'kij V.M. Analiz povedeniya algoritmov poiskovoj optimizacii na modelyah regeneriruyushchih processov slozhnyh dinamicheskih sistem. Dinamika slozhnyh sistem. 2020. T. 14. № 4. S. 65–73. DOI: 10.18127/j19997493-202004-07 (In Russian).
- Bonte M.H.A., van den Boogaard A.H., Huétink J. An optimisation strategy for industrial metal forming processes: modelling, screening and solving of optimisation problems in metal forming. University of Twente, Enschede. The Netherlands. 18 March 2008. 16 c.
- Howson C. Successful Business Intelligence, Second Edition: Unlock the Value of BI & Big Data. McGraw-Hill. 2013. 336 p.
- Manshour P. Nonlinear correlations in multifractals: Visibility graphs of magnitude and sign series. Chaos: An Interdisciplinary Journal of Nonlinear Science. 2020. V. 30(1). P. 013151.
- Perslev M., Jensen M.H., Darkner S. et al. U-time: A fully convolutional network for time series segmentation applied to sleep staging. Advances in Neural Information Processing Systems. 2019. P. 4415–4426.