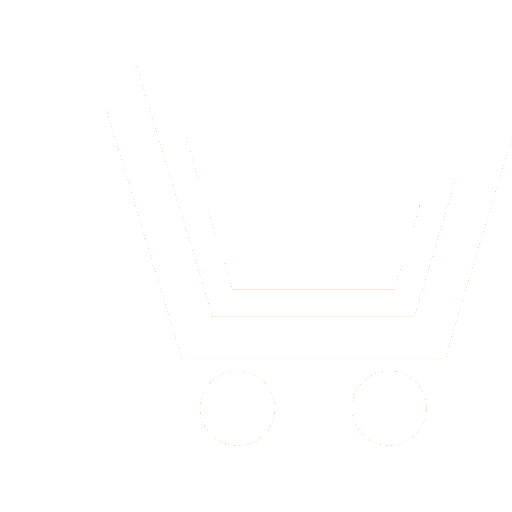
Yu.E. Gapanyuk − Ph.D. (Computer Sciences), Associate Professor,
Computer Science and Control Systems Department, Bauman Moscow State Technical University
E-mail: gapyu@bmstu.ru
T.I. Khanmurzin − Student,
Computer Science and Control Systems Department, Bauman Moscow State Technical University
E-mail: lhorifoxl@gmail.com
A.A. Kostyan− Student,
Computer Science and Control Systems Department, Bauman Moscow State Technical University
E-mail: linasmith69@yahoo.com
A.A. Fadeyev − Student,
Computer Science and Control Systems Department, Bauman Moscow State Technical University
E-mail: af0706@yandex.ru
N.R. Brysina − Student,
Computer Science and Control Systems Department, Bauman Moscow State Technical University
E-mail: brysina.natali@yandex.ru
By “conceptual model,” we mean a description of a fragment of a subject area based on concepts and the relationships between them. The most traditional graphic form for representing conceptual models is “conceptual maps.” They allow you to display concepts and the relationships between them in a graphical way. Probably the most well-known in practice approach to representing conceptual maps is a MindMap approach. From the point of view of the data model, the MindMap is a flat graph whose vertices correspond to the concepts, and the edges correspond to the relations between the concepts. Edges in this model are considered as nondirectional and not annotated (a label containing auxiliary data cannot be assigned to aa edge). From the point of view of the data model, cmap (as well as the MindMap) is a flat graph whose vertices correspond to the concepts, and the edges correspond to the relations between the concepts. In contrast to the MindMap, edges in this model are considered as directed and annotated (a label containing auxiliary data can be assigned to the edge). Having analyzed the existing approaches to presenting conceptual maps, we can conclude that the main problem of existing methods is the use of a flat graph as a model for representing a conceptual map. Therefore, to develop conceptual maps, it is proposed to use complex graphs, in particular, a metagraph model. The main element of the metagraph model is the metavertex, which includes a nested fragment of the metagraph. A metagraph model may be useful for describing concept maps. In this case, one can consider “simple” and “complex” concepts. At the same time, “simple” concepts are modeled using regular vertices, and “complex” ones using metavertices. The use of metavertices for the description of conceptual maps allows us to abandon the presentation of a conceptual map in the form of a flat graph and move on to the holonic spatial description of a conceptual map in the form of a metagraph. For visualization of conceptual maps based on a metagraph model, a first version of the Metagraph Editor software has been developed. Using the three-dimensional arrangement of concepts allows you to make conceptual models more visual, as well as model aspects that cannot be modeled using two-dimensional conceptual maps. The proposed software system allows conceptual modeling based on the metagraph approach. This eliminates the need for manual input of conceptual models, as they are recognized from a text description in a natural language.
- Averkin N.N., Gaaze-Rapoport M.G., Pospelov D.A. Tolkovyj slovar' po iskusstvennomu intellektu. M.: Radio i svjaz'. 1992. 256 s. (In Russian).
- Buzan T. Mind Map Mastery. London: Watkins Publishing. 2018. 224 p.
- Ishikawa K. Guideto Quality Control. Tokyo: Asian Productivity Organization. 1976. 244 p.
- Novak J.D., Cañas A.J. The Origins of the Concept Mapping Tool and the Continuing Evolution of the Tool. Information Visualization Journal. 2006. V. 5. № 3. Р. 175-184.
- Talvitie V. The Foundations of Psychoanalytic Theories: Project for a Scientific Enough Psychoanalysis. Karnac Books. 2018. 125 p.
- Evin I.A. Vvedenie s teoriju slozhnyh setej. Komp'juternye issledovanija i modelirovanie. 2010. T. 2. № 2. S. 121-141 (In Russian).
- Kuznecov O.P., Zhiljakova L.Ju. Slozhnye seti i kognitivnye nauki. Sb. trudov XVII Vseross. nauchno-tehnich. konf. «Nejroinformatika2015». Ch. 1. M.: MIFI. 2015. S. 18 (In Russian).
- Anohin K.V. Kognitom: gipersetevaja model'mozga. Sb. trudov XVII Vseross. nauchno-tehnich. konf. «Nejroinformatika-2015». Ch. 1. M.: NIJaU MIFI. 2015. S. 14-15 (In Russian).
- Chapela V., Criado R., Moral S., Romance M. Intentional risk management through complex networks analysis. Springer. 2015. Springer Briefs in optimization. 126 p.
- Basu A., Blanning R. Metagraphs and Their Applications. New York: Springer. 2007. 172 p.
- Chernen'kij V.M., Terehov V.I., Gapanjuk Ju.E. Struktura gibridnoj intellektual'noj informacionnoj sistemy na osnove metagrafov. Nejrokomp'jutery: razrabotka, primenenie. 2016. № 9. S. 3-14 (In Russian).
- Chernen'kij V.M., Gapanjuk Ju.E., Revunkov G.I., Terehov V.I., Kaganov Ju.T. Metagrafovyj podhod dlja opisanija gibridnyh intellektual'nyh informacionnyh sistem. Prikladnaja informatika. 2017. T. 12. № 3(69). S. 57-79 (In Russian).