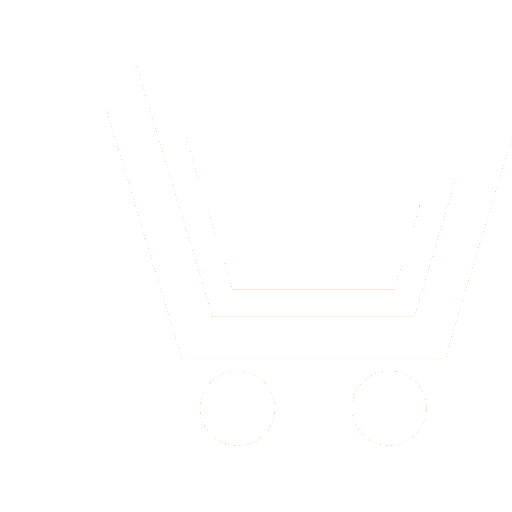
A.V. Baldin – Associate Professor,
Department «Data science and control systems», Bauman Moscow State Technical University
E-mail: bal@bmstu.ru
I.D. Eroshok – Assistant,
Department «Data science and control systems», Bauman Moscow State Technical University
E-mail: ideroshok@gmail.com
Thus, the article conducted a statistical passenger flow (Section 1) at the stopping points of the city route network. Correlation analysis showed the presence of significant correlations between different flows. Estimates of the autocorrelation functions of passenger flows are obtained and it is shown that they also have significant correlations, which suggests the possibility of constructing adequate forecast models. Spectral analysis gave the results of the presence of seasonality, and with cycles of not only the weekly component. Factor analysis gave a rather interesting result. Only the first three main components, and the route was analyzed with sixty stopping points (respectively, the number of passenger flows is also 65) give more than 90% of information. Using the multiple regression model, this allows, based on models of only three time series of the main components, to model 65 interrelated time series of passenger flows with specified correlation characteristics with a loss of accuracy not exceeding 10%.
Based on the statistical analysis, a methodology for generating passenger flows of a set of interconnected passenger flows is proposed (Section 2). An algorithm has been developed and the corresponding software components of a stationary time series generation model with a given autocovariance function. By selecting the weighting coefficients as the output, a series of moving average is formed, in which all values of the autocovariance function within a certain finite interval are equal to the specified values. The moving average coefficients are determined based on the solution of the system of nonlinear equations by the Seidel method. The simulation results showed that the estimation of the accuracy of the auto-covariance of the generated time series model with a length of about 10,000 units of model time does not exceed 0.1%, which is acceptable for modeling the urban route network.
As a result, using the proposed algorithm for generating stationary time series of the first three (or more to achieve the required information content) main components, based on the inverse transformation of the factor model, time series of passenger flows are generated at all stops (Section 3). In addition, if necessary, a non-stationary component can be superimposed on the obtained selective implementation. The results of modeling passenger flows at all stopping points of the chosen route showed that the multiple correlation coefficient, which serves as a measure of the adequacy of the multiple regression model for the formation of passenger flows, exceeds 0.97, which is also acceptable.
Thus, the approach proposed in the article gives an adequate model of the interconnected multidimensional time series of passenger flows of the route network and can be included in the generalized simulation model of transport services, which represent a theoretical basis for organizing the movement of rolling stock of vehicles.
- Volodchenko S.V. Modelirovanie raspredeleniya passazhirskih potokov v krupnyh gorodah: Avtoref. diss. … kand. tekh. nauk. SPb. 2005. 184 s. (in Russian).
- Lipenkov A.V., Maslova O.A., Eliseev M.E. O podhodah k modelirovaniyu vremeni prostoya avtobusov na ostanovochnyh punktah gorodskogo passazhirskogo transporta. Mir transporta i tekhnologicheskih mashin. 2012. № 3. S. 84−93 (in Russian).
- Nurgaliev E.R. Imitacionnoe modelirovanie passazhiropotokov pri pomoshchi programmnogo produkta ANYLOGIC s cel'yu povysheniya kachestva passazhiroperevozok. Sb. nauch. statej «Nauka: poisk-2009». Astrahan': Izd-vo AGTU. 2009. S. 223–227 (in Russian).
- Panfilov A.E., Berisheva E.D., Krushel' E.G., Stepanchenko I.V. O postroenii modeli poyavleniya passazhirov na ostanovkah gorodskogo passazhirskogo transporta. Sovremennye problemy nauki i obrazovaniya. 2009. № 5. S. 118−120 (in Russian).
- Gubanov V.A., Koval'dzhi A.K. Vydelenie sezonnyh kolebanij na osnove variacionnyh principov. Ekonomika i matematicheskie metody. 2001. T. 37. № 1. S. 91−102 (in Russian).
- Ajvazyan S.A., Mhitaryan B.C. Prikladnaya statistika i osnovy ekonometriki. M.: YUniti. 2001. 405 s. (in Russian).
- Drejper N., Smit G. Prikladnoj regressionnyj analiz: Per. s angl. Izd. 3-e. M.: Vil'yams. 2007. 912 s. (in Russian).
- Brandt Z. Analiz dannyh. Statisticheskie i vychislitel'nye metody dlya nauchnyh rabotnikov i inzhenerov. M.: Mir. OOO «Izdatel'stvo ACT». 2003. 686 s. (in Russian).
- Vorobejchikov S.E., Kabanova T.V. Ocenka parametra processa avtoregressii pervogo poryadka pri nalichii meshayushchego parametra. Vestnik Tomskogo gosudarstvennogo universiteta. Upravlenie, vychislitel'naya tekhnika i informatika, 2009. № 4. S. 26−31 (in Russian).
- Ganceva E.A., Kaladze V.A., Kaladze G.A. Dinamicheskie modeli nestacionarnyh sluchajnyh processov. Vestnik VGTU. 2006. T. 2. № 5. S. 4−8 (in Russian).
- Thomsen E. OLAP Solutions: Building multidimensional information systems. Wiley computer publishing. 2007.