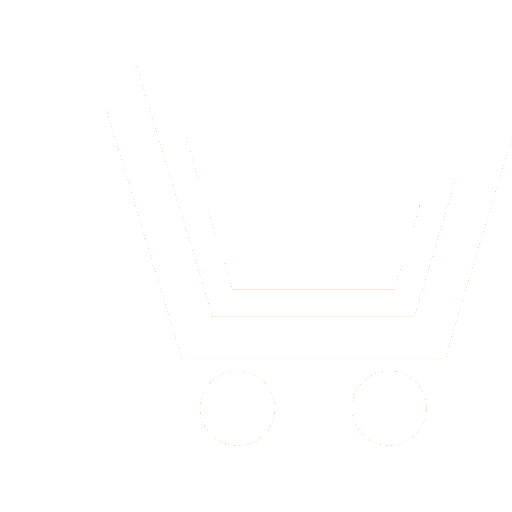
T.M. Tatarnikova1, D.D. Savelieva2
1,2 St. Petersburg State University of Aerospace Instrumentation (St. Petersburg, Russia)
1 tm-tatarn@yandex.ru
With the increase in the number of Internet of Things devices and their varieties, the threat to this segment of information and communication networks increases, which makes it relevant to create a control system for ecosystems of Internet of Things devices, such as smart home, smart city, smart manufacturing. It is important not only to detect the presence of an anomaly in the Internet of Things traffic, but also to identify the class of attack and the source of its occurrence. An analysis of work in the field of attack detection systems design has shown that their work is based either on statistical methods (allows detecting anomalies, but does not identify an attack), or on artificial intelligence methods (does not allow identifying unknown attacks), for example, expert systems or machine learning methods. The aim of the work is to propose a hybrid method for detecting anomalies in Internet of Things traffic, which allows detecting and identifying a network anomaly using the advantages of existing methods: network anomaly detection by statistical method and anomaly identification by machine learning method.
An overview of existing traffic analysis solutions for the Internet of Things system is provided. The shortcomings in the existing methods of detecting anomalies in traffic are shown. A hybrid method for detecting anomalies in Internet of Things traffic based on statistical and machine learning methods is proposed. The attack classifier was trained on the open dataset cicIoT2023. The learning error was about 1%. The proposed method makes it possible to detect anomalies in the Internet of Things traffic and identify attacks immediately after capturing traffic on the device, responding in a timely manner to emerging threats.
Tatarnikova T.M., Savelieva D.D. Hybrid method for detecting anomalies in traffic of the internet of things. Achievements of modern radioelectronics. 2024. V. 78. № 8. P. 26–32. DOI: https://doi.org/10.18127/j20700784-202408-05 [in Russian]
- Lee P. Internet of Things for Architects. Birmingham– Mumbai: Packt Publ. 2018.
- Tatarnikova T.M., Bogdanov P.Yu., Kraeva E.V. Predlozheniya po obespecheniyu bezopasnosti sistemy umnogo doma, osnovannye na otsenke potreblyaemykh resursov. Problemy informatsionnoy bezopasnosti. Komp'yuternye sistemy. 2020. № 4. S. 88–94. [in Russian]
- Pal'chevskiy E.V., Khristodulo O.I. Razrabotka metoda samoobucheniya impul'snoy neyronnoy seti dlya zashchity ot DDoS-atak. Programmnye produkty i sistemy. 2019. T. 32. № 3. S. 419–432. DOI: 10.15827/0236-235X.127.419-432. [in Russian]
- Kirichek R.V., Paramonov A.I., Prokop'ev A.V., Kucheryavyy A.E. Evolyutsiya issledovaniy v oblasti besprovodnykh sensornykh setey. Informatsionnye tekhnologii i telekommunikatsii. 2014. № 4 (8). S. 29–41. [in Russian]
- Lodneva O.N., Romasevich E.P. Analiz trafika ustroystv interneta veshchey. Sovremennye informatsionnye tekhnologii i IT-obrazovanie. 2018. T. 14. № 1. S. 149–169. [in Russian]
- Murenin I.N. Obnaruzhenie anomaliy v trafike ustroystv Interneta veshchey. Trudy uchebnykh zavedeniy svyazi. 2021 T. 7. № 4 S. 128–137. DOI:10.31854/1813-324X-2021-7-4-128-137. [in Russian]
- Safronova E.O., Zhuk G.A. Primenenie iskusstvennykh neyronnykh setey dlya prognozirovaniya DoS atak. Molodoy uchenyy. 2019. № 23. S. 27–30. [in Russian]
- Meidan Y., Bohadana M., Mathov Y., Mirsky Y., Breitenbacher D., Shabtai A., Elovici Y. N-BaIoT: Network-based Detection of IoT Botnet Attacks Using Deep Autoencoders. IEEE Pervasive Computing, Special Issue - Securing the IoT. 2018. V. 17 (3). P. 12–22.
- Tatarnikova T.M., Bogdanov P.Yu. Obnaruzhenie atak v setyakh interneta veshchey metodami mashinnogo obucheniya. Informatsionno-upravlyayushchie sistemy. 2021. № 6 (115). S. 42–52. DOI: 10.31799/1684-8853-2021-6-42-52. [in Russian]
- Tatarnikova T.M., Bimbetov F., Bogdanov P.Yu. Vyyavlenie anomaliy setevogo trafika metodom glubokogo obucheniya. SPb: Izvestiya SPbGETU LETI. 2021. № 4. S. 36–41. [in Russian]
- Sakharov D.V., Kozlov D.S. Obnaruzhenie anomal'nogo povedeniya ustroystva iot v seti na osnove modeli trafika. Informatsionnye tekhnologii i telekommunikatsii. 2019. T. 7. № 3 S. 50–55. [in Russian]
- Alzubaidi L., Zhang J., Humaidi A.J., etc. Review of deep learning: concepts, CNN architectures, challenges, applications, future directions. J. of Big Data. 2021. V. 8. № 53. P. 1–74. DOI: 10.1186/s40537-021-00444-8.
- Akcay S., Kundegorski M.E., Willcocks C.G., Breckon T.P. Using Deep Convolutional Neural Network Architectures for Object Classification and Detection Within X-Ray Baggage Security Imagery. IEEE Transact. on Information Forensics and Security. 2018. V. 13. № 9. P. 2203–2215.
- Kashkarov A.P. Datchiki v elektronnykh skhemakh. Ot prostogo k slozhnomu. M.: DMK Press. 2013. [in Russian]