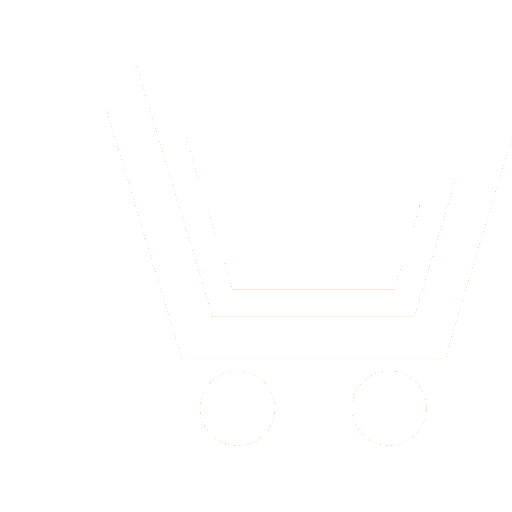
O.V. Nepomnyashchiy1, M.M.I. Al-Saghir2, A.G. Khantimirov3, S.A. Kotov4
1–4 Siberian Federal University (Krasnoyarsk, Russia)
1 2955005@gmail.com, 2 mahamedali51@yahoo.com, 4 thiskotov@yandex.ru
The challenge of identifying adverse effects resulting from intricate combinations of pharmaceuticals, known as polypragmasia, is the subject of investigation. The utilization of machine learning technologies and algorithms exhibits promising potential for significant advancements in this domain. Various methodologies for machine prediction in pharmaceutical contexts are under scrutiny, with tensor decomposition receiving particular attention due to its heightened efficiency, despite the complexities entailed in computational processes.
The primary objective is the formulation of a novel method and neural network model intended to streamline computational procedures while managing substantial datasets, thereby augmenting prediction accuracy. A novel approach to tensor decomposition has been conceived, facilitating calculations through prioritized data sampling without compromising the integrity of critical information. Additionally, an innovative approach to input vector formation and neural network architecture holds the promise of enhancing prediction precision.
Empirical validation of the proposed method illustrates its superiority over existing methodologies. Comparative analysis against established techniques reveals notable enhancements in performance metrics, computational efficiency, and prediction accuracy. Standard evaluation criteria corroborate the tangible benefits accruing from the adoption of this innovative methodology.
The considered new method and neural network model are, in our opinion, a new approach to predicting side effects of polypragmasy. In contrast to the known approaches that do not provide efficient processing of large amounts of data, the proposed method allows us to reduce processing time by using a simplified neural network model and priority principle of data sampling without loss of critical information.
The obtained results of processing available databases show that the proposed method outperforms a number of well-known methods (Deep walk, DEDICOM, RESCAL and Decagon) in terms of AUROC and AUPRC values. It should also be noted that the considered method can be most effective in identifying unique and health-critical side effects of polypragmasy.
Comparative analysis between the proposed method and the closest Decagon method, employing various data processing methodologies and algorithms, using metrics such as MCC, ACC, F-measure, recall, precision, and true positive object proportion, demonstrates the superiority of the proposed method across all indicators, except for true positives.
Nepomnyashchiy O.V., Al-Saghir M.M.I., Khantimirov A.G., Kotov S.A. Tensor decomposition method and neural network model for identifying side effects of polypragmasy. Achievements of modern radioelectronics. 2024. V. 78. № 4. P. 43–51. DOI: https://doi.org/10.18127/j20700784-202404-05 [in Russian]
- Schölkopf B., Luo Zh., Vovk V. (editors). Empirical Inference: Festschrift in Honor of Vladimir N. Vapnik. Spriger, 2013. 306 p.
- V'yugin V. Matematicheskie osnovy teorii mashinnogo obucheniya i prognozirovaniya. MCMNO, 2013. 390 s. [in Russian]
- Koren Y. Factorization Meets the Neighborhood: A Multifaceted Collaborative Filtering Model. ACM Press. 2008. Proc. 14 ACM SIGKDD Int’l Conf. Knowledge Discovery and Data Mining. P. 426–434.
- Rendle S. Factorization machines with libfm. ACM Trans. Intell. Syst. Technol. May 2012. V. 3(3). P. 57:1–57:22.
- Chollet F. Chapter 9, Section 2. Deep Learning with Python. Manning, 2017. 350 p.
- Burkov A. The Hundred-Page Machine Learning Book. Amazon Press. 2019. 160 p.
- Xie Zh., Chen C., Ma'ayan A. Dex-Benchmark: datasets and code to evaluate algorithms for transcriptomics data analysis. National Library of Medicine. 2023. Nov. V. 8. P. 11:e16351. doi: 10.7717/peerj.16351. eCollection 2023.
- Amadori, L., Calcagno, C., Fernandez, D.M. et al. Systems immunology-based drug repurposing framework to target inflammation in atherosclerosis. Nat Cardiovasc Res 2. 2023. P. 550–571. https://doi.org/10.1038/s44161-023-00278-y.
- Collins J., Sohl-Dickstein J., Sussillo D. Capacity and trainability in recurrent neural networks”. arXiv preprint arXiv:1611.09913, 2016.
- Poletaeva N.G. Klassifikaciya sistem mashinnogo obucheniya. Vestnik baltijskogo federal'nogo universiteta. 2020. № 1. S. 5–22 [in Russian].
- Zitnik M., Agrawal M., Leskovec Ju. Modeling polypharmacy side effects with graph convolutional networks. Bioinformatics. 2018. V. 34(13). P. 1457–1466.
- Nickel M., Tresp V., Kriegel H. P. A three-way model for collective learning on multi-relational data. Proceedings of the 28th International Conference on Machine Learning. ICML. 2011. V. 1. P. 809–816.
- Papalexakis, Evangelos E., Faloutsos, Christos, Sidiropoulos, Nicholas D. Tensors for data mining and data fusion: Models, applications, and scalable algorithms. ACM Transactions on Intelligent Systems and Technology. 2016. V. 8(2). P. 1–44.
- Glorot X., Bengio Yo. Understanding the difficulty of training deep feedfor-ward neural networks. Journal of Machine Learning Research. 2010. V. 9(1). P. 249–256.
- Bottou, L. Stochastic gradient descent tricks. Lecture Notes in Computer Science (including subseries Lecture Notes in Artificial Intelligence and Lecture Notes in Bioinformatics). 7700 LECTURE № 1. 2012. P. 421–436.
- Baldi P., Brunak S., Chauvin Y., Andersen C.A.F., Nielsen, H. Assessing the accuracy of prediction algorithms for classification: an overview. Bioinformatics. 2000. V. 16(5). P. 412–424.
- Vilar S., Friedman C., Hripesak G. Detection of drug-drug inter- actions through data mining studies using clinical sources, scientific literature and social media. Briefings in bioinformatics. 2018. V. 19(5). P. 863-877.
- Stein G. A., Oron G.Y., Eytan Yo. R, Roded Sh. INDI: A computational framework for inferring drug interactions and their associated recommendations. Molecular Systems Biology. 2012. V. 8(592). P. 1–12.
- Serban B., Panti Z., Nica M., Pleniceanu M., Popa M., Ene R., Cîrstoiu, C. Statistically based survival rate estimation in patients with soft tissue tumors. Romanian Journal of Orthopaedic Surgery and Traumatology. 2019. V. 1(2). P. 84–89.
- Szklarczyk D. at al. STRING v10: protein–protein interaction networks, integrated over the tree of life (angl.). Nucleic acids research (angl.) rus.: journal. 2015. V. 43. P. 447—D452. DOI:10.1093/nar/gku1003 nSIDES Drug side effect and interaction resources URL: https://nsides.io/#offsides-and-twosides (data obrashcheniya 14.03.2024).
- Stitch URL: http://stitch.embl.de/ (дата обращения 14.03.2024).