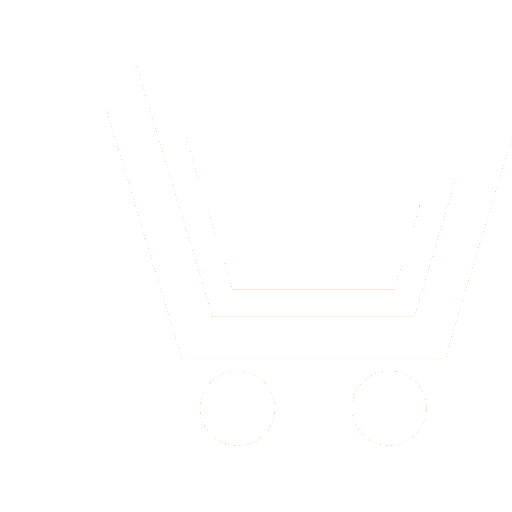
O.V. Nepomnyashchiy1, Mohammed Muanis I. Al-Sagheer2, V.N. Khaidukova3, A.V. Sharova4, S.A. Kotov5
1,2,4,5 Siberian Federal University (Krasnoyarsk, Russia)
3 JSC Academician M.F. Reshetnev «Information Satellite Systems» (Zheleznogorsk, Russia)
1 2955005@gmail.com, 2 mahamedali51@yahoo.com, 3 valeriya_iks@mail.ru, 4 withlady@yandex.ru,
5 thiskotov@yandex.ru
Problem statement. In the conditions of development of modern digital society, information technologies in medicine come to the forefront in the creation of promising scientific directions in the field of disease diagnosis, drug treatment and patient monitoring. Special attention is paid to the processing of large volumes of information when solving the tasks of analyzing the results of drug treatment or drug-drug combination interaction, the results of medical diagnosis and treatment selection. Such data are characterized not only by a large amount of data, but also by their invariance and poorly amenable to analysis by classical methods, which does not provide the required convergence of results. Thus, it is urgent to develop new, efficient computational methods and tools to solve these problems.
Goal of the work. Taking into account the need to analyze big data, the general task of creating new methods for obtaining effective solutions is highlighted. The local task of increasing the percentage of convergence - accuracy of the results obtained, as well as providing real-time diagnostics is highlighted.
Results of the work. The problem of detecting anomalies in the analysis of electrocardiograms for building systems of automated diagnostics of cardiovascular diseases is considered. The known directions in the creation of methods for determining the results of combinational analysis of cardiac signals are presented. The architecture and algorithms of the classification system functioning on the basis of neural classifier are developed. Experimental studies have been carried out on the basis of a widely used data package, the results of which have shown that the proposed approach achieves high performance in arrhythmia detection efficiency.
Practical significance. Further elaboration of this issue will make it possible to achieve the creation of a long-term prognosis system based on machine learning technologies and the proposed analyzer, which will make it possible to warn about possible heart diseases in advance based on the results of accumulated data on the patient's condition.
Nepomnyashchiy O.V., Mohammed Muanis I. Al-Sagheer, Khaidukova V.N., Sharova A.V., Aleksandrovich K.S. Convolutional neural network and deep machine learning for ECG analysis. Achievements of modern radioelectronics. 2024. V. 78. № 3. P. 70–77. DOI: https://doi.org/10.18127/j20700784-202403-08
- Acharya U.R., Fujita H., Lih O.S., Hagiwara Y., Tan J.H., Adam M. Automated detection of arrhythmias using different intervals of tachycardia ECG segments with convolutional neural network. Information sciences. 2017. V. 405. P. 81–90.
- Cao J., Brown M.L., Higgins E.J., Degroot P.J. Automatic thresholds for atrial tachyarrhythmia detection in an implantable medical device. Ed: Google Patents. 2019.
- Li F., Chen K., Ling J., Zhan Y., Manogaran G. Automatic diagnosis of cardiac arrhythmia in electrocardiograms via multigranulation computing. Applied Soft Computing. 2019. V. 80. P. 400–413.
- Wu Z., Lan T., Yang C., Nie Z. A Novel Method to Detect Multiple Arrhythmias Based on Time-frequency Analysis and Convolutional Neural Networks. IEEE Access. 2019.
- Kobernichenko V.G. Osnovy tsifrovoy obrabotki signalov: ucheb. Posobie. Ekaterinburg: Izd-vo Ural. un-ta. 2018. [in Russian]
- Alfaras M., Soriano M.C., Ortín S. A fast machine learning model for ECG-based heartbeat classification and arrhythmia detection. Frontiers in Physics. 2019. V. 7. P. 103.
- Luz E.J. d. S., Schwartz W.R., Cámara-Chávez G., Menotti D. ECG-based heartbeat classification for arrhythmia detection: A survey. Computer methods and programs in biomedicine. 2016. V. 127. P. 144–164.
- Prakash A.J., Ari S. A system for automatic cardiac arrhythmia recognition using electrocardiogram signal. Bioelectronics and Medical Devices: Elsevier. 2019. P. 891–911.
- V.H.C. de Albuquerque et al. Robust automated cardiac arrhythmia detection in ECG beat signals. Neural Computing and Applications. 2018. V. 29. № 3. P. 679–693. 2018/02/01.
- Elhaj F.A., Salim N., Ahmed T., Harris A.R., Swee T.T. Hybrid classification of Bayesian and extreme learning machine for heartbeat classification of arrhythmia detection. 6th ICT International Student Project Conference (ICT-ISPC). 2017. P. 1–4.
- Yıldırım Ö., Pławiak P., Tan R.-S., Acharya U.R. Arrhythmia detection using deep convolutional neural network with long duration ECG signals. Computers in biology and medicine. 2018. V. 102. P. 411–420.
- Isin A., Ozdalili S. Cardiac arrhythmia detection using deep learning. Procedia computer science. 2017. V. 120. P. 268–275.
- Fischer R., Akay M. Fractal analysis of heart rate variability Time Frequency and Wavelets in Biomedical Signal Processing. Wiley-IEEE Press. 1997.
- Inoue H., Miyazaki A. A noise reduction method for ECG signals using the dyadic wavelet transform. IEICE TRANSACTIONS on Fundamentals of Electronics, Communications and Computer Sciences. 1998. V. E81-A. P. 1001–1007.
- Huang J., Chen B., Yao B., He W. ECG Arrhythmia Classification Using STFT-Based Spectrogram and Convolutional Neural Network. IEEE Access. 2019. V. 7. P. 92871–92880.
- Lakhani P., Sundaram B. Deep learning at chest radiography: automated classification of pulmonary tuberculosis by using convolutional neural networks. Radiology 284:574–582, 2017.