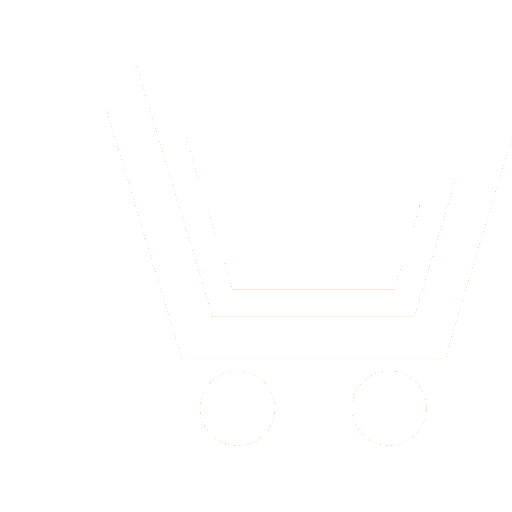
M.S. Medvedev1, A.G. Khantimirov2, D.O. Nepomnyashchy3, A.A. Charugin4
1–4 Siberian Federal University (Krasnoyarsk, Russia)
1 MMedvedev@sfu-kras.ru, 2 Akhantimirov@sfu-kras.ru
This study addresses the issue of compensating for acoustic feedback interference. It demonstrates the effectiveness of an adaptive approach for calculating the correction signal during echo filtering. The challenge of suppressing residual echo, both linear and nonlinear, is identified. A combined approach is proposed, involving the sequential use of an adaptive filter for primary suppression and a neural network for residual echo suppression. An adaptive filter model and a neural network model are developed. Data sets for testing are prepared, the network is trained, and simulation results of the noise suppression system are obtained. The results show the effectiveness of the proposed approach in suppressing acoustic feedback.
The main concern in developing systems for suppressing reverberation noise, especially in acoustic signals, is maintaining sound quality. This includes speech intelligibility and the absence of extraneous noises. These systems are widely used, from consumer audio devices to professional medical and research equipment. Adaptive filters, which calculate the correction signal based on a reference signal, yield the best results in noise suppression. Various adaptive algorithms, such as the Least Mean Squares (LMS), Normalized LMS (NLMS), and Block LMS (BLMS), are used for this purpose. However, these filters require significant computational resources, making efficient noise suppression challenging.
Fast Fourier Transform (FFT) is often used to reduce computational load. Methods that adjust sampling rates based on conditions, such as multiple speakers or changing reflective surfaces, are also known. Nevertheless, adaptive compensation algorithms do not entirely eliminate noise. After compensation, a residual signal remains, which, although smaller in amplitude, significantly affects perception. This residual signal has both linear and nonlinear components. Linear components arise from discrepancies between calculated and actual environments, while nonlinear components stem from the audio equipment's elements.
The proposed method includes a microphone block, speaker block, neural network for residual echo suppression, and an adaptive filter. The adaptive filter computes the transition characteristics of the feedback path, adjusting echo signals. This approach is suitable for linear components but not for nonlinear ones. The more electronic modules are used, the higher the nonlinear component, leading to increased residual echo amplitude. Pre-trained neural networks can compensate for this residual echo.
The proposed adaptive filter uses the Frequency-Domain Adaptive Filter (FDAF) algorithm, which allows flexible filter coefficient adjustments based on signal conditions. Error estimation and weight adaptation are performed using the FBLMS algorithm. A recurrent neural network (RNN) is used for residual echo suppression, based on spectral coefficients from both near-end and far-end signals. The RNN employs Gated Recurrent Units (GRUs) to handle sequential data, forming a vector of gain coefficients GkG_kGk for echo suppression.
A comparative analysis of common adaptive filtering algorithms and the proposed neural network module was conducted using synthesized audio data. The Echo Return Loss Enhancement (ERLE) metric was used to evaluate suppression quality. The proposed combined FDAF and neural network module showed an average ERLE value of 37.39 dB, demonstrating superior performance in both primary and residual echo suppression compared to traditional algorithms.
The preliminary study suggests that the combined method, using an FDAF adaptive filter and a pre-trained RNN, effectively suppresses both primary and residual echo. The neural network was trained using a synthesized speech database with echo effects, and the results showed significant improvements in echo suppression, as measured by the ERLE metric. Future developments may involve expanding the neural network architecture to detect and suppress additional interference sources when multiple speakers are present.
Medvedev M.S., Khantimirov A.G., Nepomnyashchy D.O., Charugin A.A. Residual echo cancellation method based on adaptive filter and machine learning technology. Achievements of modern radioelectronics. 2024. V. 78. № 12. P. 31–40. DOI: https://doi.org/10.18127/j20700784-202412-03 [in Russian]
- Vartanyan I.A. Fiziologiya sensornykh sistem: Rukovodstvo. SPb.: Lan'. 2006. [in Russian]
- Selomon D. Szhatie dannykh, izobrazheniy i zvuka: ucheb. Posobie dlya vuzov. Per. s angl. V.V. Chepyzhova. 2004. [in Russian]
- Benesty J., Rey H., Vega L.R., Tressens S. A nonparametric VSS NLMS algorithm. IEEE Signal Processing Letters. 2006. V. 13. № 10. P. 581–584.
- Paleologu C., Ciochina S., Benesty J. Double-talk robust VSS-NLMS algorithm for under-modeling acoustic echo cancellation. IEEE International Conference on Acoustics, Speech and Signal Processing. ICASSP. 2008. P. 245–248.
- Nussbaumer G. Bystroe preobrazovanie Fur'e i algoritmy vychisleniya svertok. Per. s angl. M.: Radio i svyaz'. 1985. [in Russian]
- Valin J. On adjusting the learning rate in frequency domain echo cancellation with double-talk. IEEE Transactions on Audio, Speech, and Language Processing. 2007. V. 15. № 3. P. 1030–1034.
- Yuan Z., Songtao X. Application of new LMS adaptive filtering algorithm with variable step size in adaptive echo cancellation. IEEE International Conference on Communication Technology. ICCT. 2017. P. 1715–1719.
- Mohammad A.I., Grant S.L. Novel variable step size nlms algorithms for echo cancellation. IEEE International Conference on Acoustics, Speech and Signal Processing. ICASSP. 2008. P. 241–244.
- Kondrat'ev K.V., Kochan I.V., Khantimirov A.G., Barkhatova A.A. Metod podavleniya reverberatsionnykh pomekh. Radiotekhnika. 2022. T. 86. № 12. S. 105−110. DOI: https://doi.org/10.18127/j00338486-202212-09. [in Russian]
- Tanrikulu O., Dogancay K. A new non-linear processor (NLP) for background continuity in echo control. IEEE International Conference on Acoustics, Speech, and Signal Processing. ICASSP. 2003. P. V–588.
- Doroslovacki M. Optimal non-linear processor control for residual-echo suppression. IEEE International Conference on Acoustics, Speech, and Signal Processing. ICASSP. 2003. P. V–608.
- Panda B., Kar A., Chandra M. Non-linear adaptive echo supression algorithms: A technical survey. International Conference on Communication and Signal Processing. ICCSP. 2014. P. 076–080.
- Ikram M.Z. Non-linear acoustic echo cancellation using cascaded Kalman filtering. IEEE International Conference on Acoustics, Speech and Signal Processing. ICASSP. 2014. P. 1320–1324.
- Mossi M.I., Yemdji C., Evans N. and etc. Robust and lowcost cascaded non-linear acoustic echo cancellation. IEEE International Conference on Acoustics, Speech and Signal Processing. ICASSP. 2011. P. 89–92.
- Lu Ma, Hua Huang, Pei Zhao, Tengrong Su Acoustic Echo Cancellation by Combining Adaptive Digital Filter and Recurrent Neural Network. arXiv.org, Computer Science, Engineering. 2020.
- Kondrat'ev K.V., Nepomnyashchiy D.O., Charugin A.A. Identifikatsiya neizvestnoy dinamicheskoy sistemy kak sposob opredeleniya perekhodnoy kharakteristiki obratnogo akusticheskogo trakta. Uspekhi sovremennoy radioelektroniki. 2024. T. 78. № 4. S. 36–42. DOI: https://doi.org/10.18127/j20700784-202404-04. [in Russian]
- Filter FDAF. URL: https://www.researchgate.net/publication/278177687_A_Frequency-Domain_Adaptive_Filter_FDAF_Prediction_
Error_Method_PEM_Framework_for_Double-Talk-Robust_Acoustic_Echo_Cancellation. - FBLMS. URL: https://nl.mathworks.com/help/dsp/ref/dsp.frequencydomainadaptivefilter-system-object.html
- Valin Jean-Marc A hybrid DSP/deep learning approach to realtime full-band speech enhancement. IEEE International Workshop on Multimedia Signal Processing. MMSP. 2018. P. 1–5.
- Kaval'chuk A.N., Petrovskiy A.A. Formula dlya perekhoda iz oblasti chastot k shkale barkov i obratno. Informatika. 2011. № 4(32) S. 71–81. [in Russian]
- Silero Text-To-Speech. URL: ttps://github.com/snakers4/silero-models?tab=readme-ov-file#text-to-speech.