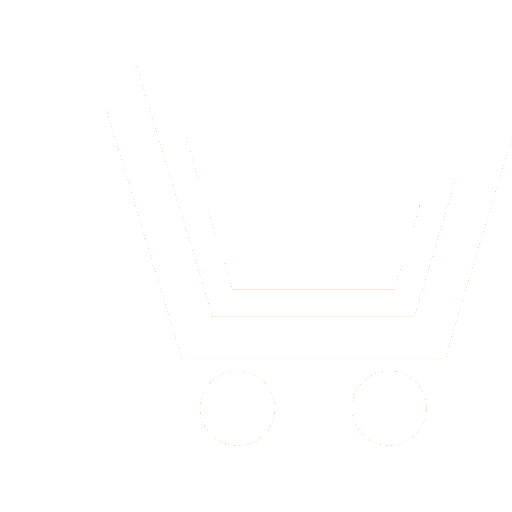
V.V. Borodin1, V.A. Shevsov2, N.G. Kovalkina3
1–3 Moscow Aviation Institute (National Research University) (Moscow, Russia)
1 doc_bor1@mail.ru, 2 vs@mai.ru, 3 natalie.life@mail.ru
Conducted studies of real traffic in various heterogeneous telecommunication environments (local networks, Internet networks, specialized networks, multiservice networks, ATM networks, etc.) show that real traffic in them meets the self-similarity property. Self-similar processes are characterized by a high degree of burstiness and have memory, their dispersion value is large (theoretically equal to infinity), and the autocorrelation series diverges. This type of traffic significantly degrades network performance (increases losses, delays, jitter) even in cases where the average traffic intensity is much lower than the potentially achievable transmission speed in a given channel. For this reason, the traditional traffic model in the form of a simple Poisson flow does not allow us to predict with a sufficient degree of accuracy the parameters of data transmission processes (in particular delays, loss levels, etc.) in packet switching networks.
The article is devoted to the study of the self-similarity properties of typical traffic models of modern data exchange networks. In practice, a large number of process models are used that, to one degree or another, meet the properties of self-similarity. Traffic models in the form of point processes have found the greatest application. The main distinguishing property of point processes is that traffic is represented as a stream of zero-duration events. The main parameter is the law of time distribution between the occurrences of neighboring events. Point processes, in turn, depending on the nature of the distribution, are divided into two groups. The first group includes recovery processes or flows, the second group includes intermittent processes or the On-Off model. The conducted studies confirmed that the traffic models used are indeed self-similar processes.
To assess the degree of self-similarity of traffic, the Hurst exponent and the total autocorrelation coefficient are used. It is shown that for traffic models in the form of point and interrupted flows, the actual self-similarity indicators differ from the theoretical ones. A new traffic model is proposed in the form of an interval random process, taking into account both the moments of packet arrival into the channel and the parameters of transmitted packets. Typical algorithms for converting traffic into the OSI model are considered.
To analyze traffic in the data exchange channel, a network simulation with the CSMA/CD protocol was carried out. This network model implements specific link and network layer protocols, generates both information and service traffic, takes into account additional delays in packet transmission due to channel occupancy, retransmissions of packets when they are distorted, the time the channel is occupied by packet transmission, as well as traffic parameters, formed by network subscribers. For this model, the self-similarity characteristics of network traffic are determined. It is shown that the self-similarity properties of traffic in a communication channel depend not so much on the type of subscriber traffic, but are induced by the communication protocols used in the data exchange network.
Borodin V.V., Shevsov V.A., Kovalkina N.G. Typical traffic models of heterogeneous networks. Achievements of modern radioelectronics. 2024. V. 78. № 10. P. 45–58. DOI: https://doi.org/10.18127/j20700784-202410-06 [in Russian]
- Al-Turjman F. Real-Time Intelligence for Heterogeneous Network: Applications, Challenges and Scenarios in IoT HetNets. Springer International Publishing. 2021.
- Tong V., Akhavain A., Chzhu P. Seti 6G. Put' ot 5G k 6G glazami razrabotchikov. Per. s angl. M.: DMK-Press. 2022. [in Russian]
- Drexler D., Takács Á., Levendovics T., Haidegger T. Handover Process of Autonomous Vehicles – Technology and Application Challenges. Acta Polytechnica Hungarica. 2019. V. 16. Doi: 10.12700/APH.16.9.2019.9.13.
- Stollings V. Sovremennye komp'yuternye seti. Per. s angl. SPB.: Piter. 2003. [in Russian]
- Shelukhin O.I., Tenyakishev A.M., Osin A.V. Fraktal'nye protsessy v telekommunikatsiyakh. Monografiya. M.: Radiotekhnika. 2003.
[in Russian] - Mah B. An empirical model of HTTP network traffic. Proceeding of INFOCOM’97. V. 2. P. 592–600.
- Moltchanov D. On-line state detection in time-varying traffic patterns. Proceedings of the 7th International Conference on Next Generation Teletraffic and Wire/Wireless Advanced Networking. 2007. P. 49–60.
- Dang T.L., Sonkoly B., Molnár S. Fractal analysis and modeling of VoIP traffic. 11th International Telecommunications Network Strategy and Planning Symposium. NETWORKS 2004. P. 123–130.
- Fedorova M.L., Ledeneva T.M. Ob issledovanii svoystva samopodobiya trafika mul'tiservisnoy seti. Vestnik VGU. Ser.: Sistemnyy analiz i informatsionnye tekhnologii. 2010. № 1. S. 46–54. [in Russian]
- Hurst H.E., Black R.P., Simaika Y.M. Long-term storage: an experimental study. London: Constable. 1965.
- Shelukhin O.I., Osin A.V., Smol'skiy S.M. Samopodobie i fraktaly. Telekommunikatsionnye prilozheniya. M.: Fizmatlit. 2008. [in Russian]
- Shelukhin O.I., Osin A.V. Vliyanie samopodobnosti trafika na optimizatsiyu parametrov telekommunikatsionnykh setey. Elektrotekhnicheskie i informatsionnye kompleksy i sistemy. 2007. № 1. T. 3. S. 55–59. [in Russian]
- Tatarnikova T.M. Statisticheskie metody issledovaniya setevogo trafika. Informatsionno-upravlyayushchie sistemy. 2018. № 5.
S. 35–43. Doi: 10.31799/1684-8853-2018-5-35-43. [in Russian] - Halgamuge S.K., Wang L. Computational Intelligence for Modelling and Prediction. Springer Science & Business Media. 2005.
- Janevski T. Traffic Analysis and Design of Wireless IP Networks. Artech House Publishers. 2003.
- Dang T.L., Sonkoly B., Molnár S. Fractal analysis and modeling of VoIP traffic. Proceedings of the 11th International Telecommunications Network Strategy and Planning Symposium. NETWORKS’2004. P. 123–130.
- Kassim M., Ismail M., Yusof M. Statistical Analysis and Modeling of Internet Traffic IP-based Network for Teletraffic Engineering. ARPN Journal of Engineering and Applied Sciences. 2015. V. 10. № 3. P. 1505–1512.
- Janevski T., Temkov D., Tudzarov A. Statistical Analysis and Modeling of the Internet Traffic. ICEST’2003. P. 170–173.
- Popoola J., Ipinyomi R.A. Empirical Performance of Weibull Self-Similar Teletraffic Model. International Journal of Engineering and Aplied Sciences (IJEAS). 2017. V. 4. № 8. P. 77–79.
- Kolesnikov A.V., Ivanov I.P., Basarab M.A. Nelineyno-dinamicheskie modeli setevogo trafika. Nelineynyy mir. 2014. T. 12. № 4. S. 44–56. [in Russian]
- Grebenshchikova A.A., Elagin V.S. Modelirovanie trafika dannykh dlya otsenki slaysinga v umnoy sisteme 5G na voskhodyashchey linii svyazi. Informatsionnye tekhnologii i telekommunikatsii. 2020. T. 8. № 2. S. 44–54. DOI: 10.31854/2307-1303-2020-8-2-44-54. [in Russian]
- Onyia C., Nnamani K., Alagbu E., Ezeagwu C. Comparative Analysis of OSI and TCP/IP Models in Network Communication. Quest Journals of Software Engineering and Simulation. 2021. V. 7. № 6. P. 8–14. DOI: 10.35629/9795-07060814.
- Baugh C., Huang J. Traffic model for 802.16 TG3 MAC/PHY simulations. IEEE 802.16 Contribution 802.16.3c-01/30r1. 2001.
- Leland W.E., Taqqu M.S., Willinger W., Wilson D.V. On the Self-Similar Nature of Ethernet Traffic (Extended Version). IEEE/ACM Transactions on Networking. 1994. V. 2. № 1. P. 1–15. DOI: 10.1109/90.282603.
- Murali K.P., Gadre V.M., Desai U.B. Broadband Network Traffic Modeling. In: Multifractal Based Network Traffic Modeling. 2003. Boston: Springer. https://doi.org/10.1007/978-1-4615-0499-3_3.
- Alheraish A., Alshebeili S., Alamri T. Regression Video Traffic Models in Broadband Networks. Journal of King Saud University - Engineering Sciences. 2005. V. 18. DOI: 10.1016/S1018-3639(18)30820-1.
- Adas A. Traffic models in broadband networks. IEEE Communications Magazine. 1997. V. 35. № 7. P. 82–89. DOI: 10.1109/35.601746.
- Cybenko G.V. Approximation by Superpositions of a Sigmoidal function. Mathematics of Control Signals and Systems. 1989. V. 2. № 4. P. 303–314.
- Kirichenko L., Radivilova T., Deineko Zh. Comparative Analysis for Estimating of the Hurst Exponent for Stationary and Nonstationary Time Series. Information Technologies & Knowledge. Kiev: ITHEA. 2011. V. 5. № 1. P. 371–388.
- Clegg R.G. A practical guide to measuring the hurst parameter. International Journal of Simulation. Systems, Science & Technology. 2006. V. 7. № 2. P. 3–14.
- Abry P., Flandrin P., Taqqu M. S., Veitch D. Self-Similar Network Traffic and Performance Evaluation. New-York: John Wiley & Sons. 2000. P. 39–88.
- Li J., Chen Y. Rescaled Range (R/S) analysis on seismic activity parameters. Acta Seismologica Sinica. 2001. V. 14. P. 148–155. DOI: 10.1007/s11589-001-0145-9.
- Zhao G., Zhou G., Wang J. Application of R/S Method for Dynamic Analysis of Additional Strain and Fracture Warning in Shaft Lining. Journal of Sensors. 2015. P. 1–7. DOI: 10.1155/2015/376498.
- Borodin V., Kolesnichenko V., Shevtsov V. Analysis of the Efficiency of Various Receipting Multiple Access Methods with Acknowledgement in IoT Networks. Inventions. 2023. V. 8. P. 105. DOI: 10.3390/inventions8040105.
- Borodin V., Shevtsov V., Petrakov А. Functioning Model of Long-Term Sensor Networks. Proceedings of 10th Computer Science On-line Conference: Lecture Notes in Networks and System. 2021. V. 228. P. 282–289. DOI: 10.1007/978-3-030-77448-6_27. [in Russian]