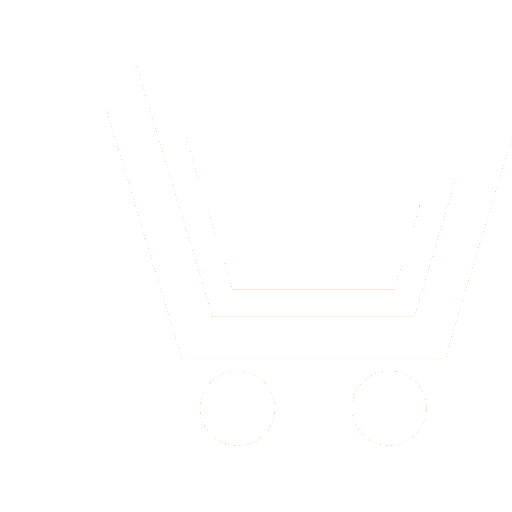
А.V. Kvasnov – Ph.D. (Eng.), Associate Professor,
Peter the Great St.Petersburg Polytechnic University
E-mail: Kvasnov_AV@spbstu.ru
P.Е. Gladilin – Ph.D. (Phys.-Math.), Associate Professor,
ITMO University (St. Petersburg)
E-mail: peter.gladilin@gmail.com
А.E. Pershutkin – Master Student, ITMO University (St. Petersburg)
E-mail: alex.neurocoder@gmail.com
The article discusses the methodology for the classification of fixed group objects which received from airborne radar in the synthetic aperture range. The radar image contains the detected artifacts of the targets for the considered frequency and distance. Group objects can be both civilian infra-structure (city blocks, industrial facilities, car parks, etc.) and military facilities at positions. The mutual arrangement of objects is regulated by construction documents and combat manuals.
Recognition features are the signal characteristics of targets and their spatial position according to some typical schemes. On the one hand, the scattered signal allows estimating the target dimension and obtaining the correlation between intensities. In the other hand, characteristics of mutual position (the number of elementary objects, the average distance between objects, distance from the location area, etc.) provide additional features for classification.
There was carried out simulation modeling for the recognition of 70 different classes of group objects by 9 independent features. It was envisaged to use an artificial neural network of the perceptron type with 10 hidden layers, which was constructed by the gradient descent method. It was found that the accuracy of target classification can reach P=0,964.
The result accuracies were carried out on based the principal component analysis. It was shown that the most uncorrelated features are the number of objects, distance from the required deployment area and list of object with different signal intensity.
Kvasnov А.V., Gladilin P.Е., Pershutkin А.E. Recognition method of fixed group objects by radar image based on artificial neural networks. Achievements of modern radioelectronics. 2020. V. 74. № 8. P. 63–71. DOI: 10.18127/j20700784-202008-07. [in Russian]
- Gini F.R.M. Knowledge based radar detection, tracking, and classification. A Wiley-Interscience publication. 2008.
- Kuz'min S.Z. Tsifrovaya radiolokatsiya. Vvedenie v teoriyu. Kiev: KVITs. 2000. [in Russian]
- Konovalov A.A. Osnovy traektornoy obrabotki radiolokatsionnoy informatsii. Ch. 1. SPb: SPbGETU «LETI». 2013. [in Russian]
- Turov V.E., Gvozdarev A.S., Krenev A.N., Polubekhin A.I., Il'in E.M. Primenenie radiogolograficheskogo podkhoda k zadache identifikatsii gruppovykh tseley v RLS Kh-diapazona. Vestnik SibGUTI. 2016. № 3. S. 139–147. [in Russian]
- Unnikrishna Pillai S., Li K.Y., Himed B. Space Based Radar: Theory and Applications. McGraw-Hill Education, 2008.
- Soumekh M. Synthetic aperture radar signal processing with MATLAB algorithms. New York: Wiley. 1999.
- Il'in E.M., Polubekhin A.I., Savost'yanov V.Yu., Samarin O.F., Cherevko A.G. Malogabaritnyy mnogofunktsional'nyy bortovoy RLK dlya bespilotnykh letatel'nykh apparatov maloy dal'nosti. Vestnik SibGUTI. 2017. № 4. S. 104–109. [in Russian]
- Savost'yanov V.Yu., Karpov O.A., Efimov A.V. Mnogofunktsional'naya malogabaritnaya RLS Ku-diapazona dlya legkogo BLA. Vseross. nauch. konf. «Sovremennye problemy distantsionnogo zondirovaniya, radiolokatsii, rasprostraneniya i difraktsii voln». Murom. 2019. S. 389–394. [in Russian]
- Il'in E.M., Repnikov D.A., Savost'yanov V.Yu., Samarin O.F., Polubekhin A.I., Cherevko A.G. Rezhimy funktsionirovaniya mnogofunktsional'noy bortovoy RLS BLA maloy i sredney dal'nosti. Vestnik SibGUTI. 2019. № 2. S. 68–86. [in Russian]
- Kvasnov A.V. Method of classification of fixed ground objects by radar images with the use of artificial neural networks. The International Conference on Cyber-Physical Systems and Control. St. Petersburg. 2019. V. 95. P. 608–616.
- Halversen S.D., Owirka G.J., Novak L.M. New Approaches for Detecting Groups of Targets. Proceedings of 1994 28th Asilomar Conference on Signals, Systems and Computers. Pacific Grove. 1995. V. 1. P. 137–140.
- Owirka G.J., Halversen S.D., Hiett M., Novak L.M. An algorithm for detecting groups of targets. Proceedings International Radar Conference. Alexandria, VA, USA. 1995. P. 641–643.
- Liu Zhoufeng, Ping Qingwei, He Peikun An improved algorithm for the detection of vehicle group targets in high-resolution SAR images. 3rd International Conference on Microwave and Millimeter Wave Technology. Beijing, China. 2002. P. 572–575.
- Huimin C., Baoshu W. A Template-based Method for Force Group Classification in Situation Assessment. 2007 IEEE Symposium on Computational Intelligence in Security and Defense Applications. Honolulu. 2007. P. 85–91.
- Kvasnov A.V., Shkodyrev V.P., Arsen'ev D.G. Vysokotochnye modeli raspoznavaniya ob"ektov na osnove rekurrentnykh bayesovskikh algoritmov. Konf. «Upravlenie v aerokosmicheskikh sistemakh» (UAS-2108). Sankt-Peterburg. 2018. S. 90–96. [in Russian]
- Kvasnov A.V. Primeneniya bayesovskogo programmirovaniya v zadachakh raspoznavaniya i klassifikatsii istochnikov radioizlucheniya. Radiotekhnika. 2020. T. 84. № 3(5). S. 5–14. [in Russian]
- Veb-sayt «Sandia National Laboratories». URL: https://www.sandia.gov/radar/imagery/index.html (data obrashcheniya: 29.05.2020).
- Monakov A.A., Mishura T.P. Radiolokatsiya protyazhennykh tseley: izmerenie dal'nosti, razreshenie i sintez signalov. Sankt-Peterburg: GUAP. 2012. [in Russian]
- Knott E., Shaeffer J., Tuley M. Radar cross section. 2nd ed. Boston: SciTech Publishing Inc., 2004.
- Kvasnov A.V. Methodology of classification and recognition of the radar emission sources based on Bayesian programming. IET Radar, Sonar & Navigation. August 2020. V. 14. № 8. P. 1175–1182. [in Russian]