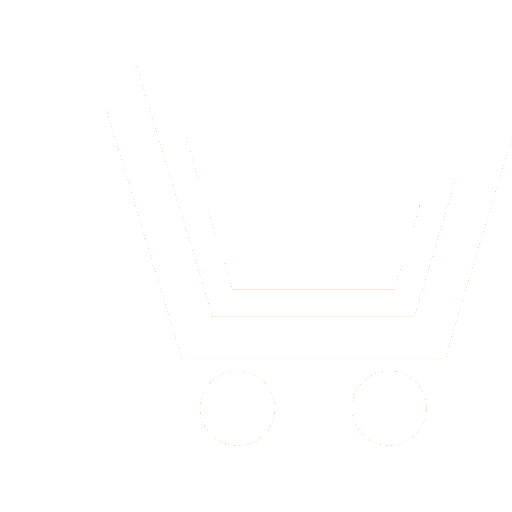
V.K. Khokhlov – Dr.Sc. (Eng.), Professor,
Bauman Moscow State Technical University
E-mail: valerykhokhlov@bmstu.ru
V.V. Glazkov – Ph.D. (Eng.), Associate Professor,
Bauman Moscow State Technical University
E-mail: glazkovv@bmstu.ru
A.K. Likhoedenko – Assistent,
Bauman Moscow State Technical University
E-mail: likhoedenkoak@bmstu.ru
In this paper, we consider the issues of selection of informative features, dimension reduction of feature vectors in regression algorithms of detection and recognition of signals and interference, as well as the issues of obtaining informative features using neural network algorithms with ill-conditioned data.
The problem is considered in relation to the short-range location, with large dynamic ranges of informative features and small decision intervals, when it is impossible to estimate mathematical expectations, that is, it is impossible to use adaptive algorithms. Regression algorithms for processing non-centered random signals are presented, with a priori unknown mathematical expectations of informative parameters, which consider the specificity of short-range location and use a priori information about the initial regression characteristics of informative features – multiple initial regression coefficients. Unlike it is in traditional regression analysis, the coefficients are determined through the elements of the matrices that are inverse to the matrices of the initial correlation moments. In regression algorithms, it is necessary to calculate the square error of multiple initial regression representations. The residual mean of squares of the initial regression representations are used to justify the methods for selection and dimension reduction of informative features of signals in the problems of detection and recognition of signals and interference.
We give examples of application of the proposed methods for the problems of detection and recognition of a helicopter and airplane by acoustic signals when processing histograms of the distributions of the durations of intervals between zeros, samples of envelopes and samples of local extrema of the power spectral density. Good separability of the {airplane} and {helicopter} classes in the space of non-centered parameters of signals (features) is shown. The issue of obtaining regression statistical characteristics with illconditioned data is considered. If the matrices of the correlation moments of the informative features of signals and noise are illconditioned, it becomes impossible to obtain a priori information about the multiple initial regression coefficients.
The possibility of using neural network algorithms to obtain estimates of the residual mean squares of regression representations and multiple initial regression coefficients through the weight coefficients on the inputs of neurons with ill-conditioned data is shown. The results can be used in short-range location systems with a large dynamic range of non-centered informative parameters, when it is not possible to estimate the mathematical expectations of the signal parameters due to the limited observation interval.
Khokhlov V.K., Glazkov V.V., Likhoedenko A.K. Substantiation of selection and dimension reduction of non-centered informative features of signals in information systems of short-range location. Achievements of modern radioelectronics. 2020. V. 74. № 8. P. 22–33. DOI: 10.18127/j20700784-202008-02. [in Russian]
- Avtonomnye informatsionnye i upravlyayushchie sistemy. V 4–kh tomakh. T. 1. Trudy kafedry «Avtonomnye informatsionnye i upravlyayushchie sistemy» MGTU im. N.E. Baumana. Pod red. A.B. Borzova. M.: OOO NITs «Inzhener», OOO «Oniko-M». 2011. [in Russian]
- Zening Fu, Shing-Chow Chan, Xin Di, Biswal B., Zhiguo Zhang Adaptive Covariance Estimation of Non-Stationary Processes and its Application to Infer Dynamic Connectivity From fMRI. IEEE Transactions on Biomedical Circuits and Systems. V. 8. № 2. DOI: 10.1109/TBCAS.2014.2306732. Publication Year: 2014. P. 228–239.
- Kirshner H., Unser M., Ward J.P. On the Unique Identification of Continuous-Time Autoregressive Models From Sampled Data. IEEE Transactions on Signal Processing. V. 62. № 6. DOI: 10.1109/TSP.2013.2296879. Publication Year: 2014. P. 1361–1376.
- Nguyen Hien D., McLachlan Geoffrey J. Asymptotic inference for hidden process regression models. Statistical Signal Processing (SSP). 2014 IEEE Workshop on. DOI: 10.1109/SSP.2014.6884624. Publication Year: 2014. P. 256–259.
- Fedorov A.V., Omelchenko A.V. Designing a polynomial regression experiment at researching into decision rules of signal recognition by modeling. Intelligent Data Acquisition and Advanced Computing Systems (IDAACS). 2013 IEEE 7th International Conference on. V. 01. DOI: 10.1109/IDAACS.2013.6662654. Publication Year: 2013. P. 124–128.
- Kheirati Roonizi E. A New Algorithm for Fitting a Gaussian Function Riding on the Polynomial Background. IEEE Journals & Magazines Signal Processing Letters. V. 20. № 11. DOI: 10.1109/LSP.2013.2280577. Publication Year: 2013. P. 1062–1065.
- Wenrui Dai, Hongkai Xiong Gaussian Process Regression Based Prediction for Lossless Image Coding. IEEE Conference Publications Data Compression Conference (DCC). DOI: 10.1109/DCC.2014.72. Publication Year: 2014. P. 93–102.
- Lin Yun, Li Jing-chao Radar signal recognition algorithms based on neural network and grey relation theory. Cross Strait Quad-Regional Radio Science and Wireless Technology Conference (CSQRWC), 2011. V. 2. DOI: 10.1109/CSQRWC.2011.6037247. Publication Year: 2011. P. 1482–1485.
- Javed A., Ejaz A., Liaqat S., Ashraf A., Ihsan M.B. Automatic target classifier for a Ground Surveillance Radar using linear discriminant analysis and Logistic regression. Radar Conference (EuRAD). 9th European Publication. Year: 2012. P. 302–305.
- Ali S.F., Jaffar J., Malik A.S. Proposed framework of Intelligent Video Automatic Target Recognition System (IVATRs). National Postgraduate Conference (NPC). DOI: 10.1109/NatPC.2011.6136315. Publication Year: 2011. P. 1–5.
- Khokhlov V.K., Likhoedenko A.K., Molchanov S.A. Regressionnye algoritmy obnaruzheniya i raspoznavaniya netsentrirovannykh nestatsionarnykh sluchaynykh protsessov v avtonomnykh informatsionnykh sistemakh blizhney lokatsii. Nauka i obrazovanie: nauchnoe izdanie MGTU im. N.E. Baumana. 2017. № 3. S. 150–169. [in Russian]
- Barabash Yu.L. i dr. Voprosy statisticheskoy teorii raspoznavaniya obrazov. M.: Sov. radio. 1967. [in Russian]
- Levin B.R. Teoreticheskie osnovy statisticheskoy radiotekhniki. Izd. 3–e, pererab. i dop. M.: Radio i svyaz'. 1989. [in Russian]
- Khokhlov V.K. Obnaruzhenie, raspoznavanie i pelengatsiya ob«ektov v blizhney lokatsii. M.: MGTU im. N.E. Baumana. 2005. [in Russian]
- Khokhlov V.K. Nachal'nye regressionnye statisticheskie kharakteristiki intervalov mezhdu nulyami sluchaynykh protsessov. Nauka i obrazovanie: nauchnoe izdanie MGTU im. N.E. Baumana. 2014. № 9. S. 132–147. [in Russian]