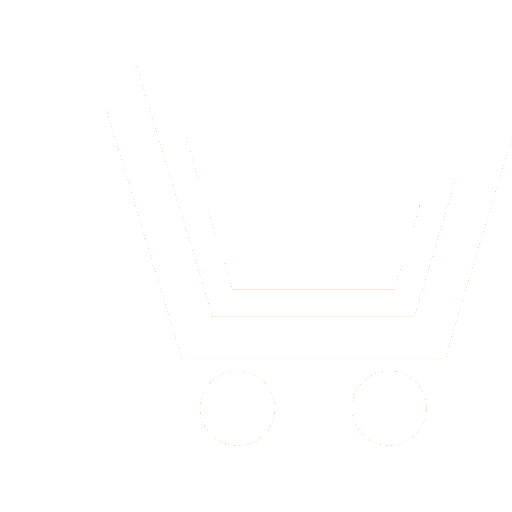
A.O. Markov – Senior Research Scientist,
LTD «STC» (St. Petersburg)
E-mail: alex.o.markov@gmail.com
S.F. Burdakov – Dr.Sc. (Eng.), Professor,
Peter the Great St. Peterburg Polytechnic University
E-mail: burdakov.s@yandex.ru
Integrated strapdown inertial and satellite navigation systems (SINS/GNSS) used for small UAVs real-time position and attitude determination basically include MEMS sensors as inertial measurement unit (IMU) with sample frequency about 500…2000 Hz. Such systems are known to be efficient within permanent availability of GNSS (GPS/GLONASS) data required for complementary filtering corrections, providing accuracy up to 0,1° for roll and pitch estimates [2]. However, since IMU-based prediction error of navigation data is growing fast with time due to MEMS sensor noise and parameter instability GNSS corrections ought to repeat at about 1 Hz frequency. This cause makes conventional integrated SINS/GNSS highly dependent on GNSS constellations visibility as well as on electromagnetic environment surrounding a UAV and thus raises the problem of its navigation system autonomy improvement. In this paper authors present the autonomous attitude determination system based on Kalman filtering process of inertial data fused with measurements of UAV accelerations (gravity-biased) and height.
Section 2 describes general principles of dynamic systems state estimation with a Kalman filter [3].
Section 3 lists typical parameters characterizing utilized sensors (Tab. 1) and UAV main properties (Tab. 2) considered in the scope of this work.
Section 4 lays out the proposed approach to roll and pitch estimation. Main features of the estimator are the following: state-space equations of the object (6) represent a kinematic relation between attitude and angular velocity directly in Euler angles formulation where singularity at pitch θ = π/2 is taken into account and resolved without auxiliary set of Euler angles; IMU bias estimations tracking is included; vertical estimation channel uses accelerometers data to predict height and make utility corrections of roll and pitch angles; discretization of continuous differential equations is performed by adaptive explicit Runge–Kutta method; accelerometers and vertical gyro measurements smoothing in order to reduce high frequency vibrations (7) and to detect whether UAV is maneuvering (8); observation model (9) operates in switching mode so that at least rough estimation of body accelerations wcntrp [4] in absence of the aircraft dynamics model could be taken into account when UAV is turning; correction steps are made to appear rarely enough, specifically at 2 Hz frequency, so that observation model errors could be regarded uncorrelated and the condition of unbiased ness kept satisfied; mechanization of covariance matrices calculation is accomplished in UD-factorized form [5].
Section 5 shows verification results of the proposed attitude determination system obtained through a test flight of «Orlan-10» UAV manufactured by LTD «STC». During the flight (Fig. 1) sensor data was collected and then postprocessed to get UAV roll and pitch estimates for different modifications of the proposed Kalman filter. For error calculation in-flight recorded data from precise integrated SINS/GNSS navigation system designed in LTD «STC» is taken as reference roll and pitch angles (Fig. 2). Comparison of switching and non-switching observation models (Fig. 3.) shows that the use of the first results in less estimation error, reducing it from 4,5° to rms. If corrections from vertical channel are added (Fig. 4.) the proposed attitude determination system achieves accuracy of 0,3°. Setting time of the filter is about 100 sec.
- Farrell J.A. Aided Navigation: GPS with High Rate Sensors. NY: McGraw-Hill. 2008.
- Kulakova V.I., Soharev A.Ju. Navigacionnaja sistema dlja soprovozhdenija traektorii dvizhenija antenny na malogabaritnom BLA. Uspehi sovremennoj radiojelektroniki. 2017. № 10. S. 5–14. [in Russian]
- Lewis F.L., Xie L., Popa D. Optimal and Robust Estimation. FL: Taylor & Francis. 2008.
- Biard R.U., MakLjejn T.U. Malye bespilotnye letatel'nye apparaty: teorija i praktika. M.: Tehnosfera. 2015. [in Russian]
- Thornton C.L. Triangular Covariance Factorization for Kalman Filtering. CA: Jet Propulsion Laboratory. 1976.