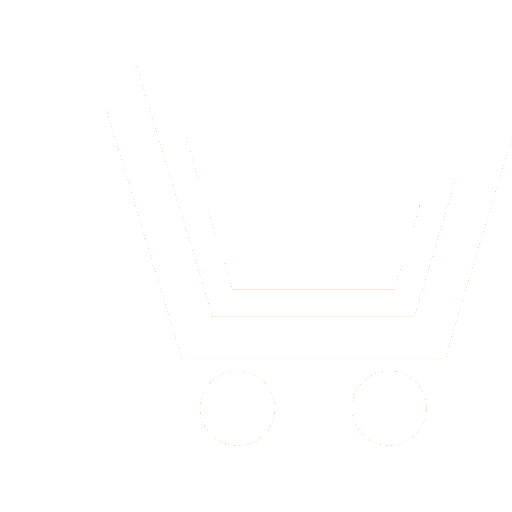
V.К. Khokhlov – Dr.Sc. (Eng.), Professor,
Department «Autonomous information and operating systems», Bauman Moscow State Technical University E-mail: khokhlov2010@yandex.ru
S.L. Sumin – Ph.D. (Eng.),
General Director, LLC «Ingenium»
E-mail: ingenium@mail.ru
А.К. Likhoedenko – Engineer,
Department «Autonomous information and operating systems», Bauman Moscow State Technical University E-mail: kost21v@rambler.ru
This paper deals with the justification of the regression algorithm for detecting and recognizing signals by the relative width of the energy spectrum, which is invariant to the average frequency of the energy spectrum of the signal and its variance, that is using the intervals between zeroes of the input realizations as informative parameters, and the initial regression coefficients as a priori information. Regression algorithms for processing non-centered random signals with a priori unknown mathematical expectation of informative parameters, using a priori information about the initial regression characteristics are given. The regression detection algorithm is justified when considering only the pair correlation in two adjacent samples of signals. The question of the initial regression characteristics of intervals between zeroes of signals stationary in the decision interval is considered, and it is shown that the initial regression coefficients of adjacent intervals between zeroes for Gaussian and rectangular energy spectra are a function only of the relative bandwidth of the energy spectra and do not depend on their average frequency and variance. A regression signal processing algorithm is justified, which is using the intervals between the zeroes of input realizations as a priori information. The statistical characteristics of the absolute value of the error of regression representation of the intervals between zeroes are studied, and expressions for the expectation and variance of the decision function are obtained. The dependencies of the mathematical expectation and variance of the signal on the relative bandwidth of the energy spectrum at the input of the threshold device for a process with a Gaussian energy spectrum are obtained, and it is shown that these characteristics depend only on the relative bandwidth of the signal energy spectrum and do not depend on its variance. The performance characteristics of the regression path, which processes the intervals between the zeroes of the input realizations are calculated, while processing 10 periods of the input realization, and the possibility of detecting and recognizing signals from the relative bandwidth of the energy spectrum is shown. The obtained results can be used in radar systems of the near location when processing signals at the Doppler frequency to increase their noise immunity when operating under active and passive interference, as well as in information systems in noise channels.
- Zening Fu, Shing-Chow Chan, Xin Di, Biswal B. Adaptive Covariance Estimation of Non-Stationary Processes and its Application to Infer Dynamic Connectivity From fMRI. IEEE Transactions. Zhiguo Zhang Biomedical Circuits and Systems. 2014. V. 8. № 2. P. 228–239. DOI: 10.1109/TBCAS.2014.2306732.
- Kirshner H., Unser M., Ward J.P. On the Unique Identification of Continuous-Time Autoregressive Models From Sampled Data. IEEE Transactions. Signal Processing. 2014. V. 62, № 6. P. 1361–1376. DOI: 10.1109/TSP.2013.2296879.
- Nguyen Hien D., McLachlan Geoffrey J. Asymptotic inference for hidden process regression models. IEEE Workshop. Statistical Signal Processing (SSP). 2014. P. 256–259. DOI: 10.1109/SSP.2014.6884624.
- Fedorov A.V., Omelchenko A.V. Designing a polynomial regression experiment at researching into decision rules of signal recognition by modeling. IEEE 7th International Conference. Intelligent Data Acquisition and Advanced Computing Systems (IDAACS). 2013. V. 01. P. 124–128. DOI: 10.1109/IDAACS.2013.6662654.
- Kheirati Roonizi E. A New Algorithm for Fitting a Gaussian Function Riding on the Polynomial Background. IEEE Journals & Magazines. Signal Processing Letters. 2013. V. 20. № 11. P. 1062–1065. DOI: 10.1109/LSP.2013.2280577.
- Wenrui Dai, Hongkai Xiong Gaussian Process Regression Based Prediction for Lossless Image Coding. IEEE Conference Publications. Data Compression Conference (DCC). 2014. P. 93–102. DOI: 10.1109/DCC.2014.72
- Signal Processing and Communications Applications (SIU). IEEE 19th Conference. 2011. P. 1008–1011. DOI: 10.1109/SIU.2011.5929824.
- Grillo D., Pasquino N., Angrisani L., Schiano Lo Moriello R. An efficient extension of the zero-crossing technique to measure frequency of noisy signals. IEEE International. Instrumentation and Measurement Technology Conference (I2MTC). 2012. P. 2706– 2709. DOI: 10.1109/I2MTC.2012.6229703.
- Khokhlov V.K., Likhoedenko A.K., Molchanov S.A. Regressionnye algoritmy obnaruzheniya i raspoznavaniya netsentrirovannykh nestatsionarnykh sluchaynykh protsessov v avtonomnykh informatsionnykh sistemakh blizhney lokatsii. Mashinostroenie i komp'yuternye tekhnologii. 2017. № 3. S. 150–169. [in Russian]
- Khokhlov V.K. Nachal'nye regressionnye statisticheskie kharakteristiki intervalov mezhdu nulyami sluchaynykh protsessov. Nauka i obrazovanie: nauchnoe izdanie MGTU im. N.E. Baumana. 2014. № 9. S. 132–147. [in Russian]
- Levin B.R. Teoreticheskie osnovy statisticheskoy radiotekhniki. V 3-kh knigakh. Kn. 1. M.: Sov. radio. 1974. [in Russian]