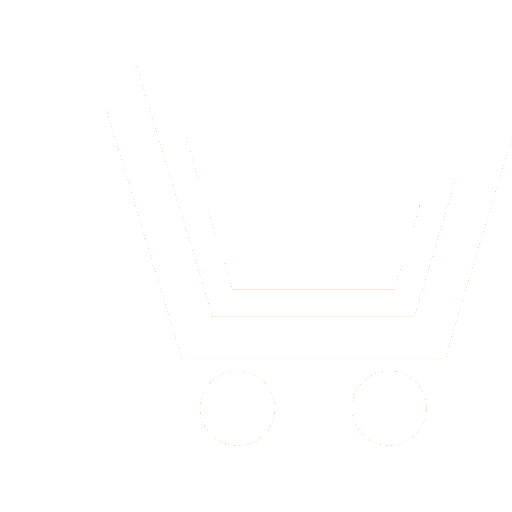
N.S. Akinshin – Dr.Sc. (Eng.), Professor,
JSC «Central Design Bureau of Apparatus Engineering» (Tula)
E-mail: cdbae@cdbae.ru
R.P. Bystrov – Dr.Sc. (Eng.), Professor, Leading Research Scientist,
Kotelnikov Institute of Radio Engineering and Electronics of RAS (Moscow)
E-mail: rudolf@cplire.ru
O.V. Esikov – Dr.Sc. (Eng.), Professor,
JSC «Central Design Bureau of Apparatus Engineering» (Tula)
E-mail: inf@cdbae.ru
A.I. Chernyshkov – Adjunct,
Penza Artillery Engineering Institute
E-mail: niriopaii@mail.ru
Modern means of remote sensing of the Earth are multi-channel, that is, receiving images from different sources and in different ranges. For such systems it is important to build an effective pattern recognition system.
An approach to the construction of a multi-channel image recognition system for sensing complexes is proposed. The list of tasks to be solved in the construction of the object recognition system in the sensing complexes in the following composition: pre-processing of images; determination of effective schemes of artificial neural networks for solving recognition problems; application of additional characteristics of objects to improve the efficiency of the recognition system; selection of the equipment of sensing complexes for solving recognition problems. The problem of image preprocessing is formulated as an ill-posed problem. It is proposed to use artificial neural networks with direct signal propagation to solve the problem of recognition of contours of image objects. The variants of increasing the efficiency of learning algorithms used to solve the problem of recognition of artificial neural networks are considered. To assess the quality of the training sample it is proposed to use Hamming network. The effectiveness of artificial neural networks for recognition of object contours was tested experimentally using the developed software. To form an additional characteristic of the objects, the application of the fractal dimension value of the images of their contours is proposed and experimentally verified. The problem of optimizing the equipment composition of the object recognition system according to the minimum cost criterion is formalized. This task is reduced to the problem of choosing the composition of the complex of technical means. Its peculiarity is the presence of restrictions on the compatibility of the means included in the complex equipment of the recognition system. The characteristic of ways of the solution of the formulated problems of construction of systems of recognition of objects is given.
- Baklanov A.I. Gruppirovki mikrosputnikov optiko-elektronnogo nablyudeniya vysokogo razresheniya. Materialy XV nauchno– tehnicheskoj konferencii «Sistemy nablyudeniya, monitoringa i distancionnogo zondirovaniya Zemli». Alushta. 2018. P. 43–62. [in Russian]
- Baklanov A.I. Novye tendencii razvitiya kosmicheskih sistem optiko-elektronnogo nablyudeniya Zemli vysokogo razresheniya. Materialy XIV nauchno-tehnicheskoj konferencii «Sistemy nablyudeniya, monitoringa i distancionnogo zondirovaniya Zemli». Sochi. 2017. P. 32–49. [in Russian]
- Shut O.V. Metod resheniya zadach raspoznavaniya na osnove logicheskoj i precedentnoj modelej. Informatika. 2012. №3. P. 35–50. [in Russian]
- Shut O.V. Sintez algoritmov dlya resheniya zadach raspoznavaniya obrazov v konechnomernyh diskretnyh prostranstvah. Vestnik BGU. Ser.1. 2014. №1. P. 56–62. [in Russian]
- Akinshin N.S., Rumyancev V.L., Mamon Yu.I., Boldin A.V. Razlichenie slabokontrastnyh radiolokacionnyh celej na fone zemnoj poverhnosti. Tula: TulGU. 2006. [in Russian]
- Zaharov I. G. Obosnovanie vybora. Teoriya praktiki. SPb: Sudostroenie. 2006. [in Russian]
- Esikov O.V., Suharev E.M., Altuhov A.V., Tarasov E.A. Ocenka effektivnosti metodov i algoritmov obrabotki graficheskoj informacii i raspoznavaniya ob'ektov monitoringa. Naukoemkie tehnologii. 2011. №4. P. 54–61. [in Russian]
- Andreev A.V., Esikov O.V., Mamon Yu.I., Akinshin R.N., Zatuchnyj D.A., Feoktistova O.G. Primenenie iskusstvennyh nejronnyh setej dlya raspoznavaniya ob'ektov na graficheskih izobrazheniyah. Pod. red. M.Yu. Smurova. Vestnik Sankt-Peterburgskogo gosudarstvennogo universiteta grazhdanskoj aviacii. S.Peterburg: SPbGUGA. 2017. № 1(14). P. 131–141. [in Russian]
- Esikov O.V., Chernyshkov A.I., Fomichev M.Yu. Struktura i osobennosti resheniya zadachi avtomatizirovannoj obrabotki i analiza izobrazhenij. Materialy XXXVIII Vserossijskoj konferencii, posvyaschennoj 75-letiyu Yuzhno-Ural'skogo gosudarstvennogo universiteta. M.: RAN. 2018. P. 136–140. [in Russian]
- Alekseev O.G. Kompleksnoe primenenie metodov diskretnoj optimizacii. M.: Nauka. 1987. [in Russian]
- Tihonov A.N., Goncharskij A.V., Stepanov V.V., Yagoda A.G. Chislennye metody resheniya nekorrektnyh zadach. M.: Nauka. 1990. [in Russian]
- Bakushinskij A.B., Goncharskij A.V. Nekorrektnye zadachi. Chislennye metody i prilozheniya. M.: Izd-vo MGU. 1989. [in Russian]
- Tatuzov A.L. Nejronnye seti v zadachah radiolokacii. M.: Radiotehnika. 2009. [in Russian]
- Hajkin S. Nejronnye seti: polnyj kurs. 2-e izd., ispr.. Per. s angl. M.: OOO «I. D. Vil'yams». 2006. [in Russian]
- Potapov A.A. Fraktaly v radiofizike i radiolokacii: Topologiya vyborki. M.: Universitetskaya kniga. 2005. [in Russian]
- Potapov A.A. Fraktaly v distancionnom zondirovanii. Zarubezhnaya radioelektronika. Uspehi sovremennoj radioelektroniki. 2000. № 6. P. 3–65. [in Russian]