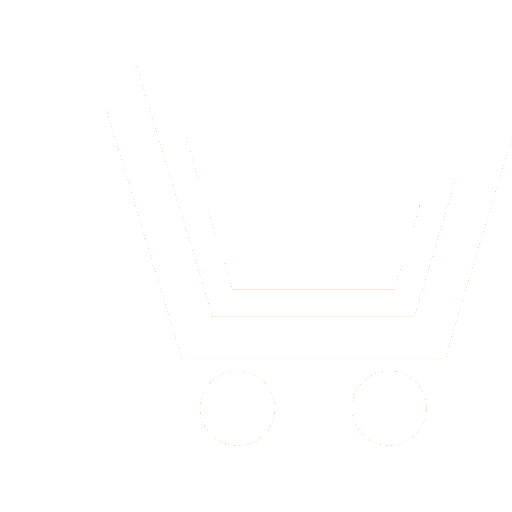
N.M. Ryabkov – Research Scientist,
JSC «Concern «Constellation» (Voronezh)
E-mail: n.m.ryabkov@sozvezdie.su
A.N. Golubinskiy – Dr.Sc. (Eng.), Associate Professor, Deputy Scientific Manager,
JSC «Concern «Constellation» (Voronezh)
E-mail: a.n.golubinskiy@ sozvezdie.su
The development of wireless technologies naturally leads to an increase in demand for a frequency resource. As a result, the existing frequency resource becomes insufficient to provide reliable and uninterrupted communication. Based on this problem, one of the promising areas of research in the field of wireless communication networks is the problem of frequency resource allocation, which can now be solved by cognitive radio technology. According to the cognitive radio system, it is a radio system that uses technology that allows this system to gain knowledge about its operating environment and geographical environment, about established rules and its internal state, dynamically and autonomously adjust its operational parameters and protocols according to the acquired knowledge to achieve predetermined goals and learn from the results. The functioning of these systems is based on monitoring the radio frequency spectrum in order to identify and further use free frequency bands. The solution to this problem is often associated with the need to process large volumes of heterogeneous weakly formalized information with non-linear dependencies in the data.
In such conditions, classical approaches do not always allow obtaining an acceptable result in practice.
The purpose of the work is to show the use of artificial neural network apparatus for determining the rating of frequency channels in order to correctly identify the most suitable frequency range for communication.
The solution of the problem of forming the rating of frequency channels using the apparatus of artificial neural networks for use in a cognitive communication system is given. A method for solving the problem using a neoclassifier based on a multilayer perceptron is proposed, the corresponding simulation is carried out. Graphs of changes in the relative error from the era of training were obtained, the most effective architecture of the artificial neural network was determined. It is noted that the results can be applied in promising communication and control systems.
The proposed method allows to obtain the output of trained artificial neural network ratings of frequency channels for the input signal-to-noise ratio values and identify the frequency channel most suitable for communication.
- Mikhalev O.A., Avanesov M.Yu., Sorokin K.N. Upravlenie radiochastotnym spektrom v kognitivnoy seti radiosvyazi. Informatsiya i kosmos. 2017. № 1. S. 57–66. [in Russian]
- Otchet (Sektora svyazi Mezhdunarodnogo soyuza elektrosvyazi) MSE-R SM.2152 (09/2009) «Opredeleniya sistemy radiosvyazi s programmiruemymi parametrami (SDR) i sistemy kognitivnogo radio (CRS)» («Definitions of Software Defined Radio (SDR) and Cognitive Radio System (CRS)»). [in Russian]
- Komashinskiy V.I., Smirnov D.A. Neyronnye seti i ikh primenenie v sistemakh upravleniya i svyazi. M.: Goryachaya liniya-Telekom. 2003. [in Russian]
- Ukaz Prezidenta Rossiyskoy Federatsii o razvitii iskusstvennogo intellekta v Rossiyskoy Federatsii № 490 ot 10.10.2019. Natsional'naya strategiya razvitiya iskusstvennogo intellekta na period do 2030 goda. http://static.kremlin.ru/media/events/files/ru/ AH4x6HgKWANwVtMOfPDhcbRpvd1HCCsv.pdf. Data obrashcheniya: 05.11.2019. [in Russian]
- Rutkovskaya D., Pil'min'skiy M., Rutkovskiy A. Neyronnye seti, geneticheskie algoritmy i nechetkie sistemy. M.: Goryachaya liniyaTelekom. 2006. [in Russian]
- Gudfellou Ya., Bendzhio I., Kurvill' A. Glubokoe obuchenie. M.: Dmk-Press. 2017. [in Russian]