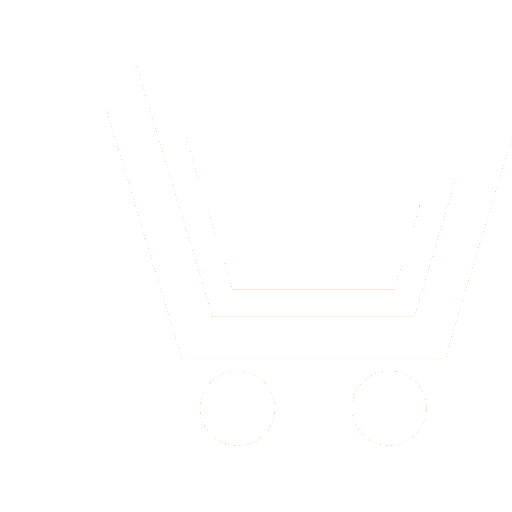
S.A. Meshkov – Ph.D. (Eng.), Associate Professor, Bauman Moscow State Technical University E-mail: sb67241@mail.ru
The numerical methods and algorithms for probabilistic analysis of the microwave microcircuits parameters for computer-aided design systems are considered. An algorithm for calculating the yield conditional probability of the multiparameter microwave devices is described. The methodological approaches to the modelling of technological spread of the parameters of microwave circuits in computer-aided design are discusses. The problem of conjugation of a subsystem for modeling design parameters technological errors with a subsystem for the deterministic analysis of microwave microcircuits electrical characteristics is discussed. The rules and examples of probabilistic modelling of the microwave circuits design parameters are provided.
- Fedorov Yu.V, Maljcev P.P, Gnatyuk D.L., Matvienko O.S., Karpukhin D.V., Gamkrelidze S.A. Sozdanie SVCh monolitnihkh IS 5-millimetrovogo diapazona dlya primeneniya v perspektivnihkh KS // Raketno-kosmicheskoe priborostroenie i informacionnihe sistemih. 2016. T. 3. V. 2. S. 73–78.
- Gudkov A.G., Bushminskiyj I.P., Dergachev F.V. i dr. Konstruktorsko-tekhnologicheskie osnovih proektirovaniya poloskovihkh mikroskhem // Pod red. I.P. Bushminskogo. M.: Radio i svyazj. 1987.
- Razevig V.D., Potapov Yu.V., Kurushin A.A. Proektirovanie SVCh ustroyjstv s pomothjyu Microwave Office. M.: Solon-Press. 2003.
- Kremer N.Sh. Teoriya veroyatnosteyj i matematicheskaya statistika. Izd. 2-e, pererab. i dop. M.: 2004.
- Mikhayjlov G.A., Voyjtishek A.V. Chislennoe statisticheskoe modelirovanie. Metodih Monte-Karlo. M.: Izdateljskiyj centr «Akademiya». 2006.
- Singhee A., Rutenbar R.A. Why Quasi-Monte Carlo is Better Than Monte Carlo or Latin Hypercube Sampling for Statistical Circuit Analysis // IEEE Transactions On Computer-Aided Design Of Integrated Circuits And Systems. 2010. V. 29. № 11. P. 1763–1776.
- Afacan E. et al. Adaptive sized quasi-monte carlo based yield aware analog circuit optimization tool // 2014 5th European Workshop on CMOS Variability (VARI). IEEE. P. 1–6.
- Canelas A. et al. Yield optimization using k-means clustering algorithm to reduce Monte Carlo simulations // 2016 13th International Conference on Synthesis, Modeling, Analysis and Simulation Methods and Applications to Circuit Design (SMACD). IEEE, P. 1–4.
- Okobiah O., Mohanty S. P., Kougianos E. Fast statistical process variation analysis using universal Kriging metamodeling: A PLL example // 2013 IEEE 56th International Midwest Symposium on Circuits and Systems (MWSCAS). IEEE. P. 277–280.
- Koziel S., Bandler J.W. Rapid Yield Estimation and Optimization of Microwave Structures Exploiting Feature-Based Statistical Analysis // IEEE Transactions On Microwave Theory And Techniques. 2015. V. 63. № 1. P. 107–114.