350 rub
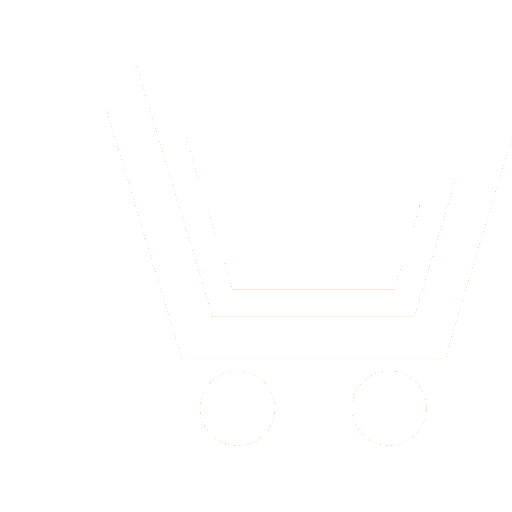
Journal Achievements of Modern Radioelectronics №6 for 2016 г.
Article in number:
Soft mask estimation technique in the problem of noisy speech signals preprocessing for speaker identification systems
Authors:
G.S. Tupitsin - Post-graduate Student, P.G. Demidov Yaroslavl State University. E-mail: genichyar@genichyar.com
А.I. Topnikov - Ph.D. (Eng.), Assistent, P.G. Demidov Yaroslavl State University. E-mail: topartgroup@gmail.com
А.L. Priorov - Dr.Sc. (Eng.), Associate Professor, P.G. Demidov Yaroslavl State University. E-mail: andcat@yandex.ru
Abstract:
Speaker identification can be performed reliably in clean acoustic conditions but their performance level severely degrade in acoustic noise presence. In this case one of the most effective ways to provide more robustness to the recognizer is using noise reduction algorithms for speech signals.
In this paper noise reduction technique based on soft mask was considered. The soft mask algorithm is similar to other algorithms in the frequency domain, but soft mask-s gain function is a probability of speech presence in each point of the time-frequency representation of the speech signal.
Soft mask was generalized: it can be raised to arbitrary power determined based on chosen optimality criterion. Dependence of the power of soft mask was analyzed. Higher value of the power provides more noise suppression, and in this case soft mask is closer to binary mask.
A technique of soft mask estimation was introduced. It uses modified decision-directed approach, Wiener gain function, and assumption that the noise amplitude spectrum is Rayleigh distributed in each frequency band.
The obtained algorithm was used as first step in two-step noise reduction algorithm. Minimum mean square error short-time spectral amplitude estimator as spectral gain function was chosen for the second step.
Smoothing a priori signal-to-noise ratio for the second step using exponential moving average with upper limit was proposed. It can reduce level of «musical» noise, but speech signals become less intelligibly.
In our experiments signals were corrupted by additive white Gaussian noise, Speech babble and Vehicle interior noise from NOISEX-92 library. Three values of signal-to-noise ratio were used. There are 5, 10, 15 dB. Three algorithms were used in our experiments: the algorithm based on decision-directed approach and Wiener gain function, the two-step algorithm based on minimum mean square error short-time spectral amplitude estimator, the proposed two-step algorithm based on soft mask and minimum mean square error short-time spectral amplitude estimator. The proposed two-step algorithm based on soft mask and minimum mean square error short-time spectral amplitude estimator demonstrates better results than existing methods in additive white Gaussian noise conditions.
Pages: 73-80
References
- Ortega-Garcia J., Gonzalez-Rodriguez J. Overview of speech enhancement techniques for automatic speaker recognition // Proceeding of Fourth International Conference on Spoken Language Processing. ICSLP - 96. IEEE. 1996. V. 2. P. 929-932.
- Lu Y., Loizou P.C. Estimators of the Magnitude-Squared Spectrum and Methods for Incorporating SNR Uncertainty // IEEE Transactions on Audio, Speech, and Language Processing. 2011. V. 19. № 5. P. 1123-1137.
- Tupicin G.S., Topnikov A.I., Priorov A.L. Predobrabotka zashumlennykh rechevykh signalov s pomoshhju binarnykh masok v zadache identifikacii diktora // Naukoemkie tekhnologii. 2015. № 11. S. 56-61.
- Tupicin G.S., Kravcov S.A., Topnikov A.I., Priorov A.L. Modifikacija algoritma ocenki binarnojj maski v zadache podavlenija shuma dlja sistemy identifikacii diktora // Proektirovanie i tekhnologija ehlektronnykh sredstv. 2015. № 3. S. 32-37.
- Renevey P., Drygajlo A. Detection of reliable features for speech recognition in noisy conditions using a statistical criterion // Proceedings of Workshop on CRAC. 2001. P. 71-74.
- Wang D. On Ideal Binary Mask As the Computational Goal of Auditory Scene Analysis // Speech Separation by Humans and Machines. - Boston: Kluwer Academic Publishers. 2005. P. 181-197.
- Wang D. Time-Frequency Masking for Speech Separation and Its Potential for Hearing Aid Design // Trends in Amplification. 2008. V. 12. № 4. P. 332-353.
- Hu Y., Loizou P. Techniques for estimating the ideal binary mask // Proc. 11th Int. Workshop Acoust. Echo Noise Control. 2008. P. 154-157.
- Jensen J., Hendriks R.C. Spectral Magnitude Minimum Mean-Square Error Estimation Using Binary and Continuous Gain Functions // IEEE Transactions on Audio, Speech, and Language Processing. 2012. V. 20. № 1. P. 92-102.
- McAulay R., Malpass M. Speech enhancement using a soft-decision noise suppression filter // IEEE Transactions on Acoustics, Speech, and Signal Processing. 1980. V. 28. № 2. P. 137-145.
- Tupicin G.S. Predobrabotka rechevykh signalov v sistemakh avtomaticheskojj identifikacii diktora // Diss. - k.t.n. Vladimir: Vladimirskijj gosudarstvennyjj universitet im. A.G. i N.G. Stoletovykh. 2015.
- Lim J., Oppenheim A. Enhancement and bandwidth compression of noisy speech // Proceedings of the IEEE. 1979. V. 67. № 12. P. 1586-1604.
- Lu Y., Loizou P.C. A geometric approach to spectral subtraction // Speech Communication. 2008. V. 50. № 6. P. 453-466.
- Plapous C., Marro C., Mauuary L., Scalart P. A two-step noise reduction technique // IEEE International Conference on Acoustics, Speech, and Signal Processing. 2004. V. 1. P. 289-292.
- Ephraim Y., Malah D. Speech enhancement using a minimum-mean square error short-time spectral amplitude estimator // IEEE Transactions on Acoustics, Speech, and Signal Processing. 1984. V. 32. № 6. P. 1109-1121.
- Ephraim Y., Malah D. Speech enhancement using a minimum mean-square error log-spectral amplitude estimator // IEEE Transactions on Acoustics, Speech, and Signal Processing. 1985. V. 33. № 2. P. 443-445.
- Tupicin G.S., Topnikov A.I., Priorov A.L. Modifikacija dvukhstupenchatogo algoritma shumopodavlenija dlja uluchshenija kachestva identifikacii diktora v uslovijakh shumov // Informacionnye sistemy i tekhnologii. 2015. № 6. S. 39-47.
- A.s. № 2015660245 Speaker Recognition Test Framework - programma dlja issledovanija algoritmov raspoznavanija diktora. Tupicin G.S., Topnikov A.I., Priorov A.L. Prioritet ot 25 sentjabrja 2015 g.
- Davis S., Mermelstein P. Comparison of parametric representations for monosyllabic word recognition in continuously spoken sentences // IEEE Transactions on Acoustics, Speech, and Signal Processing. 1980. V. 28. № 4. P. 357-366.
- Mel Frequency Cepstral Coefficient (MFCC) tutorial - Practical cryptography [EHlektronnyjj resurs]. - Rezhim dostupa: http://practicalcryptography.com/miscellaneous/machine-learning/guide-mel-frequency-cepstral-coefficients-mfccs/.
- Pervushin E.A. Obzor osnovnykh metodov raspoznavanija diktorov // Matematicheskie struktury i modelirovanie. 2011. № 24. S. 41-54.
- Reynolds D.A., Quatieri T.F., Dunn R.B. Speaker Verification Using Adapted Gaussian Mixture Models // Digital Signal Processing. 2000. V. 10. № 1-3. P. 19-41.
- Varga A., Steeneken H.J.M. Assessment for automatic speech recognition: II. NOISEX-92: A database and an experiment to study the effect of additive noise on speech recognition systems // Speech Communication. 1993. V. 12. № 3. P. 247-251.