350 rub
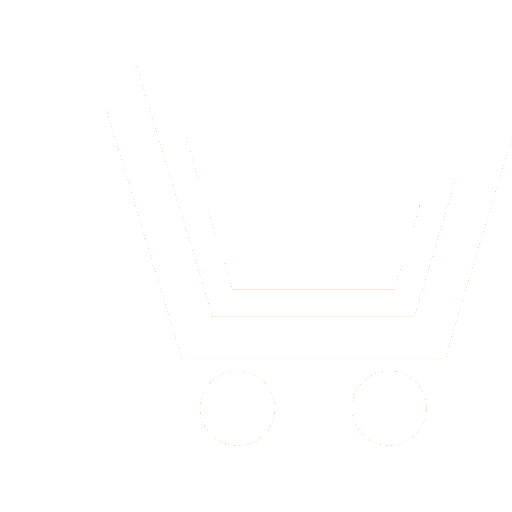
Journal Achievements of Modern Radioelectronics №12 for 2012 г.
Article in number:
Capon algorithm of direction finding with super resolution: decrease of peak and background of space spectrum under limited volume of training sample data
Keywords:
direction finding with super resolution
Capon algorithm
limited volume of training sample data
noise eigenvalues
perturbations of sample covariance matrix
Authors:
M.V. Ratynsky, A.A. Komov
Abstract:
When Capon algorithm uses true covariance matrix (CM) of array input signals, the heights of space spectrum (SS) signal peak and background are determined by signal and noise eigenvalues (EV) of CM correspondingly. If the algorithm uses sample CM under limited number K of training samples, the heights of SS peak and background decrease, and for K = N, where N is the number of antenna array elements, decrease amounts to 15-30 dB and more. The decrease on average is the same for peak and background if measured at logarithmic scale (peak and background decrease by the same factor). The decrease does not depend on antenna array configuration and grows slightly as N increases.
The peak and background decrease analysis is carried out by digital simulation and analytically. In analytical estimation the published results on largest noise EV of sample CM distribution (Tracy-Widom distribution) and on signal and noise subspaces of sample CM perturbations have been utilized.
It is proved that the background decrease is a consequence of the spread of noise EV of sample CM of array input signals, the spread is the greater the smaller is the volume of training sample data. The peak decrease is a consequence of the same noise EV spread in combination with nonorthogonality of hypothesis vector for desired signal source and CM noise eigenvectors, the nonorthogonality becomes greater as the volume of training sample data reduces.
Pages: 3-9
References
- Capon, J.,High-resolutionfrequency-wavenumberspectrumanalysis // Proc. IEEE. 1969. V. 57. № 8. P. 1408 - 1418.
- Ратынский М. В.Адаптация и сверхразрешение в антенных решетках. М.: Радио и связь. 2003.
- Krim, H., Viberg, M.,Two decades of array signal processing research // IEEE signal processing magazine. 1996. № 7. P. 67 - 94.
- Леховицкий Д. И., Флексер П. М., Атаманский Д. В., Кириллов И. Г. Статистический анализ сверхразрешающих методов пеленгации источников шумовых излучений в АР при конечном объеме обучающей выборки // Антенны. 2000. Вып.2(45). С.23 - 39.
- Richmond, C. D.,Capon algorithm mean-squared error threshold SNR prediction and probability of resolution // IEEE Trans. Signalprocess. 2005. V. 53. № 8. P. 2748 - 2764.
- Леховицкий Д. И. Статистический анализ сверхразрешающих методов пеленгации источников шумовых излучений в АР при конечном объеме обучающей выборки // Прикладная радиоэлектроника (Украина). 2009. Т. 8. № 4. С. 526 - 541.
- Johnson, B. A., Abramovich, Y. I., Mestre, X., MUSIC, G-MUSIC, and maximum-likelihood performance breakdown // IEEE Trans. Signal process. 2008. V. 56. № 8. P. 3944 - 3958.
- Abramovich, Y. I., Johnson, B. A.,Detection-estimation of very close emitters: performance breakdown, ambiguity, and general statistical analysis of maximum-likelihood estimation // IEEE Trans. Signal process. 2010. V. 58. № 7. P. 3647 - 3660.
- El Karoui, N., A rate of convergence result for the largest eigenvalue of complex white Wishart matrices // Ann. of probab. 2006. V. 34. № 6. P. 2077 - 2117.
- Tracy, C. A., Widom, H., The distributions of random matrix theory and their applications // Stanford Institute for theoretical economics. Summer 2008 workshop. Segment 7: Complex data in economics and finance - spatial models, social networks and factor models (www.stanford.edu/group/SITE/SITE_2008/segment_7/papers/tracy_SITE7_tracy.pdf).
- Kritchman, S., Nadler, B., Non-parametric detection of the number of signals: hypothesis testing and random matrix theory // IEEE Trans. Signal process. 2009. V. 57. № 10. P. 3930 - 3941.
- Li, F., Liu, H., Vaccaro, R. J., Performance analysis for DOA estimation algorithms: unification, simplification, and observations // IEEE Trans. Aerospace and electronic syst. 1993. V. 29. № 4. P.1170 - 1183.