350 rub
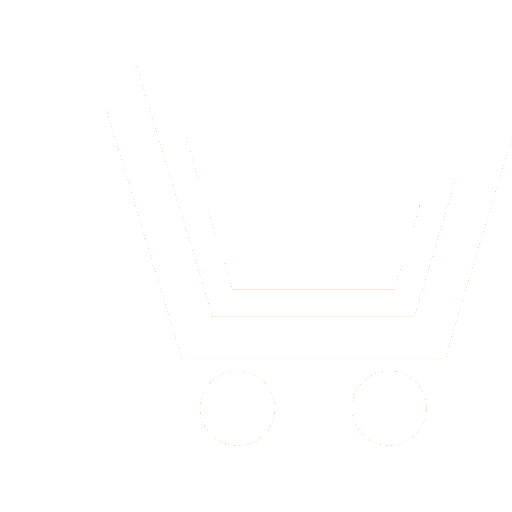
Journal Achievements of Modern Radioelectronics №11 for 2012 г.
Article in number:
Method of performance enhancement of detection algorithms of number of signal sources
Keywords:
super-resolution algorithms
information theoretic criteria
determining the number of sources
adaptive principal component extraction
Authors:
I.D. Zolotarev, K.A. Sidorenko
Abstract:
In modern radio direction-finders speed is the most important parameter along with the resolution capability. One possible way to improve response speed is to reduce the computational cost of the methods used to resolve the radio sources. Most super resolution algorithms, such as MUSIC and ESPRIT, require signal subspace dimension assessment to work correctly. The most popular methods to determine the signal subspace dimension, such as EDC, are based on finding the minimum information criteria. The present work demonstrates that for the given algorithms the most computationally expensive step is the definition of eigen-values of the correlation matrix.
To improve the performance of these methods it has been proposed to use the adaptive algorithms GHA and APEX during calculation of eigen-values. During the simulation it has been demonstrated that the APEX-EDC has comparable performance and a higher rate of convergence than the existing solution in this area - the algorithm PASTD-EDC, but it is much less resistant to noise. GHA-EDC algorithm shows even less resistance to noise than APEX-EDC, at a comparable rate of convergence. It can therefore be concluded that the use of these techniques is justified for high signal/noise ratio and high demands for speed finder.
Pages: 58-62
References
- Марпл-мл., С. Л.Цифровой спектральный анализ и его приложения. M.: Мир. 1990.
- Valizadeh, A., Karimi, M.,Subspace tracking on the constrained projection approximation approach. // 15thEuropean Signal Processing Conference (EUSIPCO 2007). 2007. P. 105-109.
- Wax, M., Kailath, T., Detection of signals by information theoretic criteria // IEEE Trans. Acoustic, Speech, and Signal Processing. 1985. V. 33. Issue 2. P. 387-392.
- Голуб Дж., Ван Лоун. Ч. Матричные вычисления. М.: Мир. 1999. С. 548.
- Haykin, S., Neural Networks: Comprehensive Foundation. 2nd edition NJ: Prentice Hall. 1998.