350 rub
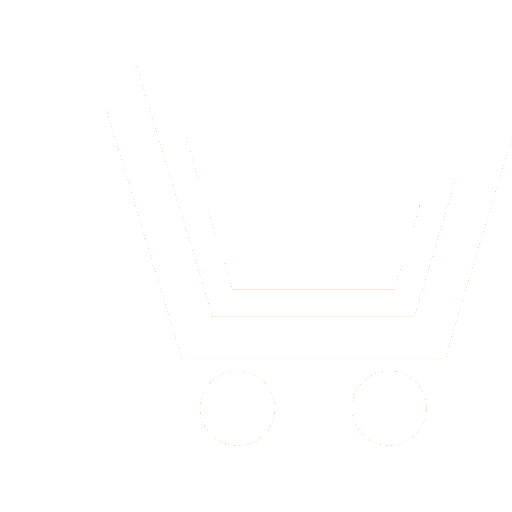
Journal Achievements of Modern Radioelectronics №10 for 2009 г.
Article in number:
TID2008 - A database for evaluation of full-reference visual quality assessment metrics
Authors:
N. N. Ponomarenko, V. V. Lukin, A. A. Zelensky, K. O. Egiazarian, J. Astola, M. Carli, F. Battisti
Abstract:
In this paper, a new image database, TID2008, for evaluation of full-reference visual quality assessment metrics is described. It contains 1700 test images (25 reference images, 17 types of distortions for each reference image, 4 different levels of each type of distortion). A comparative analysis of TID20008 and its nearest analog LIVE Database (only 5 types of distortions, less statistical accuracy) is presented. Mean Opinion Scores (MOS) for TID2008 database have been obtained as a result of more than 800 experiments. During these tests, observers from three countries (Finland, Italy, and Ukraine) have carried out about 256000 individual human quality judgments. The obtained MOS can be used for effective testing of different visual quality metrics as well as for the design of new metrics. Using the designed image database, we have tested several known quality metrics. The values of rank correlations of Spearman and Kendall with the considered metrics and Mean Opinion Score obtained by exploiting TID2008 in experiments are presented. The metrics are verified for both full set of distorted test images in TID2008 and for particular subsets of TID2008 that include distortions most important for digital image processing applications. It is demonstrated that for the considered wide range of possible distortion types no existing metric performs well enough. In aggregate, the best results are provided by Multiscale Structural Similarity Index Metric (MSSIM). For this metric Spearman Correlation with MOS is 0.853, Kendall correlation with MOS is 0.654. Analysis has been also performed for particular subsets of distortion types. This analysis has shown that for most typical practical applications like image filtering and compression the metrics PSNR-HVS and PSNR-HVS-M produce reasonably good results (Spearman correlation is 0.929, Kendall correlation is 0.765). The designed test image database is available for downloading and utilization in scientific investigations.
Pages: 30-45
References
- Keelan, B. W., Handbook of Image Quality. New York, USA: Marcel Dekker, 2002.
- Barner, K. and Arce, G., Nonlinear Signal and Image Processing: Theory, Methods, and Applications (Electrical Engineering & Applied Signal Processing Series). CRC Press, ISBN-10: 0849314275. 2003.
- Yuen, M. and Wu, H. R., A survey of hybrid MC/DPCM/DCT video coding distortions // Signal Processing. 1998. V. 70. P. 247-278.
- Watson, A. B., Digital Images and Human Vision // MIT Press. 1993. Ch. 3. P. 139-140.
- Ponomarenko, N., Lukin, V., Zelensky, A., Egiazarian, K., and Astola, J., Locally adaptive image filtering based on learning with clustering // Proc. of Image Processing: Algorithms and Systems IV. 2005. P. 94-105.
- Wang, Z., Bovik, A., Sheikh, H., andSimoncelli, E., Image quality assessment: from error visibility to structural similarity // IEEE Transactions on Image Processing. 2004. V. 13. Issue 4. P. 600-612.
- Ponomarenko, N., Silvestri, F., Egiazarian, K., Carli, M., Astola, J., and Lukin, V.,On between-coefficient contrast masking of DCT basis functions // Proc. of the Third International Workshop on Video Processing and Quality Metrics. USA. 2007.
- Wang, Z., Simoncelli, E. P., andBovik, A. C., Multi-scale structural similarity for image quality assessment // IEEE Asilomar Conference on Signals, Systems and Computers. 2003. P. 1398-1402.
- Chandler, D. M. and Hemami, S. S., VSNR: A Wavelet-Based Visual Signal-to-Noise Ratio for Natural Images // IEEE Transactions on Image Processing. 2007. V. 16 (9). P. 2284-2298.
- Sheikh, H. R., Sabir, M. F., andBovik, A. C., A Statistical Evaluation of Recent Full Reference Image Quality Assessment Algorithms // IEEE Transactions on Image Processing. 2006. V. 15. No. 11. P. 3441-3452.
- Ponomarenko, N., Carli, M., Lukin, V., Egiazarian, K., Astola, J., and Battisti, F., Color Image Database for Evaluation of Image Quality Metrics // Proc. of the International Workshop on Multimedia Signal Processing. 2008. P. 403-408.
- Ponomarenko, N., Battisti, F., Egiazarian, K., Astola, J., and Lukin, V., Metrics performance comparison for color image database // Proc. of the 4th International Workshop on Video Processing and Quality Metrics for Consumer Electronics. 2009.
- Kendall, M. G., The advanced theory of statistics. V. 1, London, UK, Charles Griffin & Company limited. 1945.
- Kodak Lossless True Color Image Suite. URL: http://r0k.us/graphics/kodak/
- Berns, R. S., Principles of Color Technology, John Wiley & Sons, ISBN 0-471-19459-X. 2000.
- Bazhyna, A. V., Egiazarian, K. O., Ponomarenko, N. N., and Lukin, V. V.,Compression of noisy Bayer pattern color filter array images?, Proc. of the SPIE Conference on Computational Imaging V. V. 6498. 2007.
- Bayer, B., Color imaging array, U.S. Patent 3971065. 1976.
- Papoulis, A., Poisson Process and Shot Noise, Ch. 16 in Probability, Random Variables, and Stochastic Processes, 2nd ed. New York: McGraw-Hill. 1984. P. 554-576.
- Foi, A., Alenius, S., Trimeche, M., Katkovnik, V., and Egiazarian, K., A spatially adaptive Poissonian image deblurring // Proc. of IEEE International Conference on Image Processing. 2005. P. 925-928.
- Han, X. H., Chen, Y. W., Nakao, Z., andLu, H., ICA-domain filtering of Poisson noise images // Proc. of the SPIE Third International Symposium on Multispectral Image Processing and Pattern Recognition. 2003. V. 5286. P. 811-814.
- Cox, I. J., Miller, M. L., Bloom, J. A., Fridrich, J., and Kalker, T., Digital Watermarking and Steganography. 2nd Edition. Morgan Kaufmann. ISBN-13: 978-0-12-372585-1. 2008.
- Carli, M., Perceptual Aspects in Data Hiding. Thesis for the degree of Doctor of Technology. Tampere University of Technology. 2008.
- Plataniotis, K. N., and Venetsanopoulos, A. N., Color Image Processing and Applications. Springer Verlag. ISBN 3-540-66953-1. 2000.
- Vansteenkiste, E., Van der Weken, D., Philips, W., and Kerre, E., Psycho-visual quality assessment of state-of-the-art denoising schemes // Proceedings of EISIPCO. 2006.
- Guleryuz, O. G., Nonlinear approximation based image recovery using adaptive sparse reconstructions and iterated denoising-part I: theory // IEEE Transaction on Image Proc. 2006. V. 15. Issue 3. P. 555-571.
- Dabov, K., Foi, A., Katkovnik, V., andEgiazarian, K., Image denoising by sparse 3-D transform-domain collaborative filtering // IEEE Transactions On Image Processing. 2007. V. 16. Issue 8. P. 2080-2095.
- ITU (2002). Methodology for Subjective Assessment of the Quality of Television Pictures Recommendation BT.500-1, International Telecommunication Union, Geneva, Switzerland.
- Egiazarian, K., Astola, J., Ponomarenko, N., Lukin, V., Battisti, F., and Carli, M., New full-reference quality metrics based on HVS // Proc. of the Second International Workshop on Video Processing and Quality Metrics. Scottsdale. 2006.
- Keelan, B. W. andUrabe, H., ISO 20462, A psychophysical image quality measurement standard, Image Quality and System Performance // Proc.of SPIE-IS&T Electronic Imaging. 2004. V. 5294. P. 181-189.
- Gaubatz, M., Metrix MUX Visual Quality Assessment Package. URL: http://foulard.ece.cornell.edu/gaubatz/metrix_mux/
- Sheikh, H. R. andBovik, A. C., Image Information and Visual Quality // IEEE Transactions on Image Processing. 2006. V. 15. P. 430-444.
- Damera-Venkata, N., Kite, T., Geisler, W., Evans, B., Bovik, A., Image Quality Assessment Based on a Degradation Model // IEEE Transanction on Image Processing. 2000. V. 9. P. 636-650.
- Wang, Z. and Bovik, A. C., A universal image quality index // IEEE Signal Processing Letters. 2002. V. 9. P. 81-84.
- Kolpatzik, B. W. andBouman, C. A., Optimized Universal Color Palette Design for Error Diffusion // Journal Electronic Imaging. 1995. V. 4. P. 131-143.
- Kolpatzik, B. andBouman, C., Optimized Error Diffusion for High Quality Image Display // Journal Electronic Imaging. 1992. V. 1. P. 277-292.
- Sheikh, H. R., Bovik, A. C., andde Veciana, G., An information fidelity criterion for image quality assessment using natural scene statistics // IEEE Transactions on Image Proc. 2005. V. 14. No. 12. P. 2117-2128.
- Mitsa, T. and Varkur, K., Evaluation of contrast sensitivity functions for the formulation of quality measures incorporated in halftoning algorithms // IEEE International Conference on Acoustic, Speech, and Signal processing. 1993. V. 5. P. 301-304.
- Watson, A. B., DCTune: A technique for visual optimization of DCT quantization matrices for individual images // Soc. Inf. Display Dig. Tech. Papers. 1993. V. XXIV. P. 946-949.
- De Simone, F., Ticca, D., Dufaux, F., Ansorge, M., and Ebrahimi, T.,A Comparative study of color image compression standards using perceptually driven quality metrics // Proc.of SPIE, Applications of Digital Image Processing XXXI. 2008. V. 7073. P. 70730Z-70730Z-11.
- PSNR-HVS-M page. URL: http://ponomarenko.info/psnrhvsm.htm
- TID2008 page. URL: http://ponomarenko.info/tid2008.htm