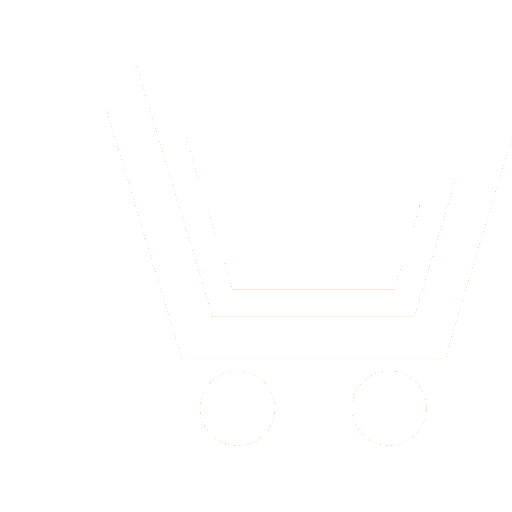
O.V. Drozd1
1 Siberian Federal University (Krasnoyarsk, Russia)
1 odrozd@sfu-kras.ru
Modern advances in smart sensors and the Industrial Internet of Things provide the ability to continuously monitor the operation of process equipment, assess its performance and operating efficiency. At the same time, a large data array is generated on the current state of the control objects, which makes it possible to use artificial intelligence methods to solve problems of predicting failures and generating recommendations for maintenance. Also, to solve the actual problem the automating operational control of technological equipment, predicting its condition and visualizing recommendations for maintenance, human-machine interfaces using augmented reality technology can be used. The aim of this work is to implement mechanisms for operational control and forecasting the state of technological equipment in the mechanical processing production conditions. To achieve this aim, the operational control system of technological equipment using augmented reality technology was developed. The technical implementation and results of the proposed operational control system of technological equipment hardware and software simulation are considered in detail.
The proposed operational control system includes: the portable terminal with built-in software blocks for image processing, obtaining information about the state of the controlled object and creating the augmented reality space; the control computer with built-in software blocks for statistical data processing, predictive analytics and the operational control database; a field-level Industrial Internet of Things observer with the set of sensors for recording the parameters of the target controlled object with an individual graphic marker. The process of technological equipment operational control involves the registration and statistical analysis of the control object parameters, training of the neural network model with subsequent formation of the predicted parameter values array, registration and operational processing the individual graphic markers of target objects with formatting and output of received recommendations using augmented reality technology.
During the implementation of the hardware and software simulation a vertical milling machining center with numerical control is considered as an object of the operational control. The simulation results showed that the used statistical analysis methods do not provide prediction the functional parameters values of the operational control object. In this case, a combined machine learning model in the form of the convolutional and recurrent neural networks sequential connection can be used to solve this problem. The portable terminal graphical user interface is implemented using augmented reality technology and provides operational updating and display of current parameters and results of forecasting the control object state with an overlay on its image.
Drozd O.V. Operational Control System of Technological Equipment Using Augmented Reality Technology. Dynamics of complex systems. 2025. V. 19. № 1. P. 24−32. DOI: 10.18127/j19997493-202501-03 (in Russian).
- Yan W., Shi Y., Ji Z., Sui Y., Tian Z., Wang W., Cao Q. Intelligent predictive maintenance of hydraulic systems based on virtual knowledge graph. Engineering Applications of Artificial Intelligence. 2023. V. 126. P. 106798. DOI: 10.1016/j.engappai.2023.106798.
- Hurtado J., Salvati D., Semola R., Bosio M., Lomonaco V. Continual learning for predictive maintenance: Overview and challenges. Intelligent Systems with Applications. 2023. V. 19. P. 200251. DOI: 10.1016/j.iswa.2023.200251.
- Liao R., He Y., Feng T., Yang X., Dai W., Zhang W. Mission reliability-driven risk-based predictive maintenance approach of multistate manufacturing system. Reliability Engineering & System Safety. 2023. V. 236. P. 109273. DOI: 10.1016/j.ress.2023.109273.
- Zhong D., Xia Z., Zhu Y., Duan J. Overview of predictive maintenance based on digital twin technology. Heliyon. 2023. V. 9, № 4. P. e14534. DOI: 10.1016/j.heliyon.2023.e14534.
- Zhuang L., Xu A., Wang X.-L. A prognostic driven predictive maintenance framework based on Bayesian deep learning. Reliability Engineering & System Safety. 2023. V. 234. P. 109181. DOI: 10.1016/j.ress.2023.109181.
- Wu D., Jennings C., Terpenny J., Gao R.X., Kumara S. A Comparative Study on Machine Learning Algorithms for Smart Manufacturing: Tool Wear Prediction Using Random Forests. ASME Journal of Manufacturing Science and Engineering. 2017. V. 139, № 7. P. 071018. DOI: 10.1115/1.4036350.
- Feng Q., Zhang Y., Sun B., Guo X., Fan D., Ren Y., Song Y., Wang Z. Multi-level predictive maintenance of smart manufacturing systems driven by digital twin: A matheuristics approach. Journal of Manufacturing Systems. 2023. V. 68. P. 443–454. DOI: 10.1016/j.jmsy.2023.05.004.
- Wang B., Zheng P., Yin Y., Shih A., Wang L. Toward human-centric smart manufacturing: A human-cyber-physical systems (HCPS) perspective. Journal of Manufacturing Systems. 2022. V. 63. P. 471–490. DOI: 10.1016/j.jmsy.2022.05.005.
- Liu Q, Liu M., Zhou H., Yan F., Ma Y., Shen W. Intelligent manufacturing system with human-cyber-physical fusion and collaboration for process fine control. Journal of Manufacturing Systems. 2022. V. 64. P. 149–169. DOI: 10.1016/j.jmsy.2022.06.004.
- Taşci B., Omar A., Ayvaz S. Remaining useful lifetime prediction for predictive maintenance in manufacturing. Computers & Industrial Engineering. 2023. V. 184. P. 109566. DOI: 10.1016/j.cie.2023.109566.
- Nasir V., Sassani F. A review on deep learning in machining and tool monitoring: methods, opportunities, and challenges. The International Journal of Advanced Manufacturing Technology. 2021. V. 115. P. 2683–2709. DOI: 10.1007/s00170-021-07325-7.