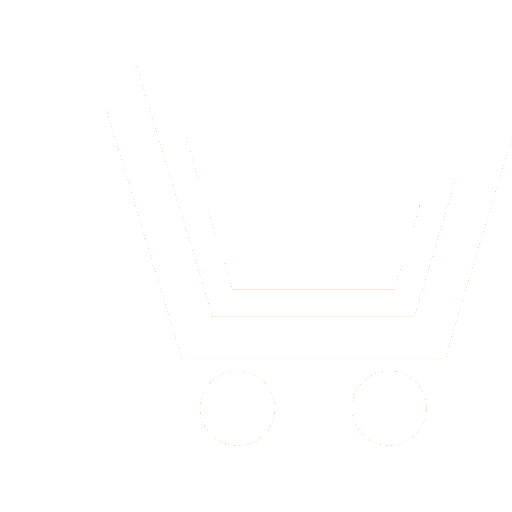
K.S. Myshenkov1, Nekoula Haddad2
1,2 Bauman Moscow State Technical University (Moscow, Russia)
1 myshenkovks@bmstu.ru, 2 nekoulahaddad@gmail.com
Artificial intelligence (AI) has notably progressed within the healthcare sector, particularly through advancements in natural language processing (NLP), which enhance data analysis efficiency and accuracy. Electronic medical records (EMRs) have become crucial data sources for enhancing healthcare quality, research, and decision-making. However, much of the valuable information within EMRs remains hidden in unstructured text, posing significant challenges for traditional data extraction and analysis methods.
Recent AI-driven innovations in clinical text analysis, employing machine learning and deep learning algorithms, have significantly improved the extraction of meaningful information from extensive medical text data, supplanting less effective rule-based approaches.
The proposed system for analyzing medical text data and evaluating physician prescriptions against standards represents a significant step towards digital transformation in healthcare. It addresses critical factors such as the exponential growth of medical data, the complexity and diversity of information, the need for standard compliance, the importance of accuracy and error minimization, and the speed of data processing. Additionally, it supports decision-making, enhances service quality, and aids scientific research by identifying new patterns and trends in large datasets.
The domain-specific terminology model developed within the system demonstrated better results in terms of data processing speed and classification accuracy compared to existing machine learning models based on the BERT model. The proposed system ensures increased efficiency of physician prescription evaluation processes, compliance with established standards, and improved patient care. The results obtained represent a significant step forward in the development of decision support systems in medicine. With further research and development aimed at improving text vectorization methods and expanding text similarity calculation methods, this approach has the potential to revolutionize evaluation procedures in healthcare, ultimately benefiting both healthcare professionals and patients.
Myshenkov K.S., Haddad N. Analysis of natural language processing methods for use in decision support systems in medicine. Dynamics of complex systems. 2024. V. 18. № 4. P. 17−27. DOI: 10.18127/j19997493-202404-02 (in Russian).
- Haddad N., Levadny I., Dmitriev A. Analysis of P300 Features for Target Stimulus Detection Using Artificial Neural Network with Small Dataset for BCI Tasks. 2021 Ural Symposium on Biomedical Engineering, Radioelectronics and Information Technology (USBEREIT). Russian Federation, Yekaterinburg, 13–14 May 2021. IEEE, 2021. P. 149–152. DOI 10.1109/USBEREIT51232.2021.9454995.
- Wang X., Dong D., Chi X. et al. sEMG-based consecutive estimation of human lower limb movement by using multi-branch neural network. Biomedical Signal Processing and Control. 2021. V. 68. № 102781. P. 1–9. DOI 10.1016/j.bspc.2021.102781.
- Kanev A., Terekhov V., Kochneva M. et al. Hybrid Intelligent System of Crisis Assessment using Natural Language Processing and Metagraph Knowledge Base. 2021 IEEE Conference of Russian Young Researchers in Electrical and Electronic Engineering (ElConRus). Russian Federation, St. Petersburg, Moscow, 26–29 Jan. 2021. IEEE. 2021. P. 2099–2103. DOI 10.1109/ElConRus51938.2021.9396100.
- Kanev A., Terekhov V., Chernenky V., Proletarsky A. Metagraph Knowledge Base and Natural Language Processing Pipeline for Event Extraction and Time Concept Analysis. 2021 IEEE Conference of Russian Young Researchers in Electrical and Electronic Engineering (ElConRus). Russian Federation, St. Petersburg, Moscow, 26–29 Jan. 2021. IEEE. 2021. P. 2104–2109. DOI 10.1109/ElConRus51938.2021.9396541.
- Gridina N.V., Zolotenkova G.V., Rogachev A.I., Pigolkin Yu.I. Instrumental'noe obespechenie resheniya zadachi klassifikacii pri sudebno-medicinskoj diagnostike biologicheskogo vozrasta neopoznannogo individuuma // Sistemy vysokoj dostupnosti. 2020. T. 16. № 4. S. 64−70. DOI 10.18127/j20729472-202004-06 (in Russian).
- Seinen T.M., Fridgeirsson E.A., Ioannou S. et al. Use of unstructured text in prognostic clinical prediction models: a systematic review. Journal of the American Medical Informatics Association. 2022. V. 29. № 7. P. 1292–1302. DOI 10.1093/jamia/ocac058.
- Datta S., Bernstam E.V., Roberts K. A frame semantic overview of NLP-based information extraction for cancer-related EHR notes. Biomedical Informatics. 2019. V. 100. № 103301. P. 1–15. DOI 10.1016/j.jbi.2019.103301.
- Volkov A.S., CHernen'kij M.V., Silant'eva E.Yu. Dvuhetapnaya procedura nejrosetevogo analiza tonal'nosti tekstov na russkom yazyke // Dinamika slozhnyh sistem – XXI vek. 2021. T. 15. № 4. S. 5−13. DOI 10.18127/j19997493-202104-01 (in Russian).
- Myshenkov K.S., Haddad N. Ispol'zovanie metodov mashinnogo obucheniya dlya prognozirovaniya nevrologicheskih zabolevanij // Dinamika slozhnyh sistem – XXI vek. 2022. T. 16. № 1. S. 66–74. DOI 10.18127/j19997493-202201-07 (in Russian).
- Wallace E., Smith S.M., Fahey T., Roland M. Reducing emergency admissions through community based interventions. British Medical Journal. 2016. V. 352. № h6817. DOI 10.1136/bmj.h6817.
- Haddad N., Myshenkov K.S., Afanasiev G.I. Introducing Text Analysis Algorithms in Decision Support Systems for Automated Evaluation of the Doctor Prescriptions. 2024 6th International Youth Conference on Radio Electronics, Electrical and Power Engineering (REEPE). Russian Federation, Moscow, 29 Feb. 2 Mar. 2024. IEEE. 2024. P. 1–5. DOI 10.1109/REEPE60449.2024.10479914.
- Rahutomo F., Kitasuka T., Aritsugi M. Semantic Cosine Similarity. 7th International Student Conference on Advanced Science and Technology (ICAST 2012). South Korea, Seoul, Oct. 2012. Seoul: University of Seoul, 2012. V. 4. № 1. P. 1–2.
- Niwattanakul S., Singthongchai J., Naenudorn E., Wanapu S. Using of Jaccard Coefficient for Keywords Similarity. International MultiConference of Engineers and Computer Scientists (IMECS 2013). Hong Kong, 13–15 Mar. 2013. 2013. V. 1. № 6. P. 380–384.
- Greenhill S.J. Levenshtein Distances Fail to Identify Language Relationships Accurately. Computational Linguistics. 2011. V. 37. № 4. P. 689–698. DOI 10.1162/COLI_a_00073.
- Sjenel L.K., Yücesoy V., Koç A., Çukur T. Semantic similarity between Turkish and European languages using word embeddings. 2017 25th Signal Processing and Communications Applications Conference (SIU). Turkey, Antalya, 15–18 May 2017. IEEE. 2017. P. 1–4. DOI 10.1109/SIU.2017.7960365.
- Yang X., Liu J., Chen Z., Wu W. Semi-supervised learning of dialogue acts using sentence similarity based on word embeddings. 2014 International Conference on Audio, Language and Image Processing. China, Shanghai, 7–9 July 2014. IEEE, 2015. P. 882–886. DOI 10.1109/ICALIP.2014.7009921.
- Gupta V., Giesselbach S., Rüping S., Bauckhage Ch. Improving Word Embeddings Using Kernel PCA. 4th Workshop on Representation Learning for NLP (RepL4NLP–2019). Italy, Florence, 2 Aug. 2019. Association for Computational Linguistics, 2019. P. 200–208. DOI 10.18653/v1/w19-4323.
- Lee S., Baker J., Song J., Wetherbe J.C. An Empirical Comparison of Four Text Mining Methods. 2010 43rd Hawaii International Conference on System Sciences (HICSS). USA, HI, Honolulu, 5–8 Jan. 2010. IEEE, 2010. P. 1–10. DOI 10.1109/HICSS.2010.48.
- Du L., Hu C. Text similarity detection method of power customer service work order based on TFIDF algorithm. 2022 IEEE 5th International Conference on Information Systems and Computer Aided Education (ICISCAE). China, Dalian. 23–25 Sept. 2022. IEEE. 2022. P. 978–982. DOI 10.1109/ICISCAE55891.2022.9927512.
- Cheng B., Li X., Chang Y. Eliminating Negative Word Similarities for Measuring Document Distances: A Thoroughly Empirical Study on Word Mover’s Distance. IEEE Transactions on Neural Networks and Learning Systems. 2024. V. 35. № 6. P. 7936–7948. DOI 10.1109/TNNLS.2022.3222336.
- Kusner M.J., Sun Y., Kolkin N.I., Weinberger K. From Word Embeddings to Document Distances. 32nd International Conference on Machine Learning (ICML-2015). France, Lille, 2015. JMLR W&CP. 2015. V. 37. P. 957–966.
- Akef S., Bokaei M.H., Sameti H. Training Doc2Vec on a Corpus of Persian Poems to Answer Thematic Similarity Multiple-Choice Questions. 2020 10th International Symposium on Telecommunications (IST). Iran. Tehran. 15–17 Dec. 2020. IEEE. 2021. P. 146–149. DOI 10.1109/IST50524.2020.9345918.
- Egger R., Yu J. A Topic Modeling Comparison Between LDA, NMF, Top2Vec, and BERTopic to Demystify Twitter Posts. Frontiers in Sociology. 2022. V. 7. P. 1–16. DOI 10.3389/fsoc.2022.886498.
- Alvi N., Talukder K.H. Sentiment Analysis of Bengali Text using CountVectorizer with Logistic Regression. 2021 12th International Conference on Computing Communication and Networking Technologies (ICCCNT). India, Kharagpur. 6–8 July 2021. IEEE. 2021. P. 1–5. DOI 10.1109/ICCCNT51525.2021.9580017.
- Goyal R. Evaluation of rule-based, CountVectorizer, and Word2Vec machine learning models for tweet analysis to improve disaster relief. 2021 IEEE Global Humanitarian Technology Conference (GHTC). USA. WA. Seattle. 19–23 Oct. 2021. IEEE. 2021. P. 16–19. DOI 10.1109/GHTC53159.2021.9612486.
- Standarty pervichnoj mediko-sanitarnoj pomoshchi. URL: https://minzdrav.gov.ru/ministry/61/22/stranitsa-979/stranitsa-983/1-standarty-pervichnoy-mediko-sanitarnoy-pomoschi (data obrashcheniya: 21.10.2023) (in Russian).
- Bulgakova O.S., Baranceva V.I. Obshchij klinicheskij analiz krovi kak metod opredeleniya poststressornoj reabilitacii // Uspekhi sovremennogo estestvoznaniya. 2009. № 6. P. 22–28 (in Russian).
- Tun N.L., Gavrilov A., Tun N.M. et al. Remote Sensing Data Classification Using A Hybrid Pre-Trained VGG16 CNN- SVM Classifier. 2021 IEEE Conference of Russian Young Researchers in Electrical and Electronic Engineering (ElConRus). Russian Federation, St. Petersburg, Moscow. 26–29 Jan. 2021. IEEE. 2021. P. 2171–2175. DOI 10.1109/ElConRus51938.2021.9396706.
- Liu N., Hu Q., Xu H. et al. Med-BERT: A Pretraining Framework for Medical Records Named Entity Recognition. IEEE Transactions on Industrial Informatics. 2022. V. 18. № 8. P. 5600–5608. DOI 10.1109/TII.2021.3131180.
- Rönnqvist S., Kanerva J., Salakoski T., Ginther F. Is Multilingual BERT Fluent in Language Generation? 22nd Nordic Conference on Computational Linguistics: First NLPL Workshop on Deep Learning for Natural Language Processing. Finland. Turku: Linköping University Electronic Press. 2019. P. 29–36. DOI 10.48550/arXiv.1910.03806.
- Pogrebnoy D., Funkner A., Kovalchuk S.V. RuMedSpellchecker: Correcting Spelling Errors for Natural Russian Language in Electronic Health Records Using Machine Learning Techniques. 23rd International Conference on Computational Science (ICCS 2023). Czech Republic, Prague, Czech Technical University in Prague, 3–5 July. 2023: Lecture Notes in Computer Science (LNCS): Proceedings. Part III. Cham: Springer. 2023. V. 10475. P. 213–227. DOI 10.1007/978-3-031-36024-4_16.