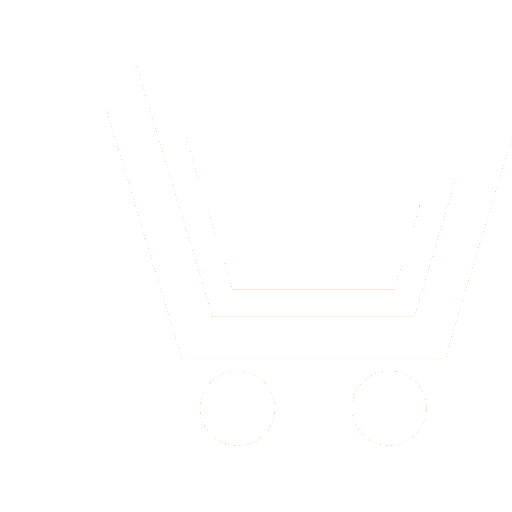
I.M. Akhmetov1, L.V. Labunets2, Ya.I. Efryushkina3
1–3 Bauman Moscow State Technical University (Moscow, Russia)
2 Russian New University (Moscow, Russia)
The article is devoted to the actual problem of recognition of 3D objects by a laser location system based on the detection of patterns hidden in the time profile of reflected signals.
Target. Substantiation of the stages of a systematic approach to the automated design of blocks for intelligent analysis of signals of optoelectronic location systems for the synthesis and analysis of algorithms for classifying 3D objects.
Based on the morphological analysis of the impulse reflection characteristics, an expert model of six initial features characterizing the dimensions, energy properties, and shape of 3D objects is proposed. The main component method is used to map informative features into a two-dimensional space. The cluster data structure in the space of the main features is investigated. Machine learning methods have been used to obtain optimal robust estimates for the parameters of alternative algorithms for classifying 3D objects. The cross-validation method confirmed the presence of the generalization property of the discriminant functions of classifiers.
Based on the morphological analysis of reflected signals, the efficiency of solving the problem of recognizing objects with a complex electrophysical structure and shape by means of a single-position short-pulse laser location system by the price-quality criterion is demonstrated.
Akhmetov I.M., Labunets L.V., Efryushkina Ya.I. The 3D-objects recognition by a short-pulse laser location system based on morphological analysis of reflected signals. Dynamics of complex systems. 2023. V. 17. № 3. P. 51−57. DOI: 10.18127/j19997493-202303-07 (in Russian).
- Labunets L.V., Borzov A.B., Akhmetov I.M. Regularized parametric model of the angular distribution of the brightness factor of a rough surface. Journal of Optical Technology. 2019. V. 86. № 10. P. 618–626. https://doi.org/10.1364/JOT.86.000618.
- Labunets L.V., Anishchenko N.N. Structural analysis of transient characteristics of 3D-objects in a monostatic optical location system. Journal of Communications Technology and Electronics. 2011. V. 56. № 2. P. 145–159. DOI: 10.1134/S1064226911010050.
- Labunets L.V., Lukin D.S., Chervyakov A.A. Reconsrtuction of Reflection Characteristics of 3D Objects in a Monostatic Optical Location System. Journal of Communications Technology and Electronics. 2012. V. 57. № 12. P. 1265–1275. DOI: 10.1134/S1064226 912110058.
- Labunets L.V., Borzov A.B., Akhmetov I.M. Real-time models of pulsed reflectance profiles of 3D objects in a monostatic laser location system. Journal of Optical Technology. 2020. V. 87. № 9. P. 513–520.
- Labunets L.V., Borzov A.B., Akhmetov I.M. Recognition of 3D objects in a monostatic laser location system via intelligent analysis of pulsed reflectance profiles. Journal of Optical Technology. 2022. V. 89. № 4. P. 217–224.
- Breuß M., Bruckstein A.M., Kiselman C.O., Maragos P. Shape Analysis: Euclidean, Discrete and Algebraic Geometric Methods (Dagstuhl Seminar 18422) – Dagstuhl Reports. 2019. 17 p. https://drops.dagstuhl.de/opus/volltexte/2019/10349/pdf/dagrep_v008_i010_p087_ 18422.pdf
- Batista G., Eamonn J. Keogh, Oben Moses Tataw, Vinícius M.A. de Souza CID: an efficient complexity-invariant distance for time series. Data Mining and Knowledge Discovery. 2014. V. 28. № 3. P. 634–669.
- Martin Ester, Hans-Peter Kriegel, Jörg Sander, Xiaowei Xu A density-based algorithm for discovering clusters in large spatial databases with noise. Proceedings of the Second International Conference on Knowledge Discovery and Data Mining (KDD-96). Evangelos Si-moudis, Jiawei Han, Usama M. Fayyad. AAAI Press. 1996. P. 226–231.
- Simon S. Haykin, Neural Networks. A Comprehensive Foundation. 2nd ed. N.Y.: Prentice Hall. 1999. 842 p.
- Platt John, Scholkopf B., Burges C.J.C., Smola A.J., eds. Fast Training of Support Vector Machines using Sequential Minimal Optimization. Advances in Kernel Methods – Support Vector Learning. Cambridge, MA, MIT Press. 1999. P. 185–208.