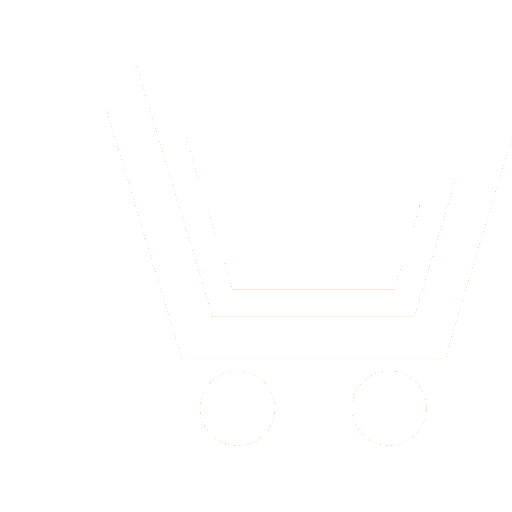
S.I. Chumachenko1, V.I. Terekhov2, E.T. Mitrofanov3, I.A. Grishin4
1, 3 Mytishchi branch of Bauman Moscow State Technical University
2, 4 Bauman Moscow State Technical University (Moscow, Russia)
LiDAR laser scanning provides three-dimensional point clouds of scanned objects. The obtained records of the coordinates of points in space open up the possibility for a detailed survey of the forest stand in remote conditions. The LiDAR data of the forest areas make it possible to implement the assessment of stands by the enumerative method, which is based on the data of a complete enumeration of trees over the entire area of the forest area, in a short time and automatically. However, methods for estimating stand parameters in dense point clouds need to be improved. The key preliminary task of forest inventory in the conditions of such processing is the extraction of individual trees from the forest area.
Target – consider and implement a procedure for determining the parameters of individual trees using LiDAR data. Compare the results of measurements of parameters on two data sets obtained by automatic and manual segmentation with the results of field measurements of the parameters of the site.
In this paper, the authors considered and implemented a procedure for determining the parameters of individual trees using LiDAR data. The sets of extracted trees for their evaluation were obtained in two different ways: manual segmentation and automatic division of the plot using a Voronoi diagram into subregions, each of which contains only one tree. The input data for splitting, which is tree coordinates, is obtained automatically by analyzing the bottom layer of the cloud using the density-based clustering method. Then, two sets of trees were processed in order to assess taxation parameters, the calculated values were compared with the results of field measurements.
The results of the estimation of the parameters, as well as insignificant differences in the parameters between automatic and manual separation, give grounds to assert that the use of such automatic separation to determine the main taxation parameters is possible in the framework of solving such problems and is recommended, especially for areas with a low planting density. The considered method of division of the site can significantly reduce the time of segmentation of simple areas of the forest.
Chumachenko S.I., Terekhov V.I., Mitrofanov E.T., Grishin I.A. An approach for estimating trees parameters using LiDAR data. Dynamics of complex systems. 2022. V. 16. № 4. P. 63−73. DOI: 10.18127/j19997493-202204-06 (in Russian).
- Rossi L. et al. Urban Planning Insights from Tree Inventories and Their Regulating Ecosystem Services Assessment. Sustainability. 2022. V. 14. № 3. P. 1684.
- Ramalho de Oliveira L. F. et al. Moving to automated tree inventory: Comparison of uas-derived lidar and photogrammetric data with manual ground estimates. Remote Sensing. 2020. V. 13. № 1. P. 72.
- Terekhov V. et al. Classification of Tree Species by Trunk Image Using Conventional Neural Network and Augmentation of the Training Sample Using a Telegram-Bot. International Conference on Neuroinformatics. Springer. Cham. 2021. P. 210–216.
- Lin Y. C. et al. Leaf-off and leaf-on uav lidar surveys for single-tree inventory in forest plantations. Drones. 2021. V. 5. № 4.
P. 115. - Hamraz H., Contreras M. A., Zhang J. A robust approach for tree segmentation in deciduous forests using small-footprint airborne LiDAR data. International journal of applied earth observation and geoinformation. 2016. V. 52. P. 532–541.
- Hamraz H., Contreras M. A., Zhang J. Forest understory trees can be segmented accurately within sufficiently dense airborne laser scanning point clouds. Scientific Reports. 2017. V. 7. № 1. P. 1–9.
- Windrim L., Bryson M. Forest tree detection and segmentation using high resolution airborne LiDAR. 2019 IEEE/RSJ International Conference on Intelligent Robots and Systems (IROS). IEEE, 2019. P. 3898–3904.
- Suárez J. C. et al. Use of airborne LiDAR and aerial photography in the estimation of individual tree heights in forestry. Computers & Geosciences. 2005. V. 31. № 2. P. 253–262.
- Popescu S. C. Estimating biomass of individual pine trees using airborne lidar. Biomass and Bioenergy. 2007. V. 31. № 9. P. 646–655.
- Dassot M., Constant T., Fournier M. The use of terrestrial LiDAR technology in forest science: application fields, benefits and challenges. Annals of forest science. 2011. V. 68. № 5. P. 959–974.
- Malhi Y. et al. New perspectives on the ecology of tree structure and tree communities through terrestrial laser scanning. Interface Focus. 2018. V. 8. № 2. P. 20170052.
- Raumonen P. et al. Fast automatic precision tree models from terrestrial laser scanner data. Remote Sensing. 2013. V. 5. № 2. P. 491–520.
- Disney M. I. et al. Weighing trees with lasers: advances, challenges and opportunities. Interface Focus. 2018. V. 8. № 2.
P. 20170048. - Åkerblom M. et al. Non-intersecting leaf insertion algorithm for tree structure models. Interface Focus. 2018. V. 8. № 2.
P. 20170045. - Gonzalez de Tanago J. et al. Estimation of above‐ground biomass of large tropical trees with terrestrial LiDAR. Methods in Ecology and Evolution. 2018. V. 9. № 2. P. 223–234.
- Dong W., Isler V. Tree morphology for phenotyping from semantics-based mapping in orchard environments //arXiv preprint arXiv:1804.05905. 2018.
- Trochta J. et al. 3D Forest: An application for descriptions of three-dimensional forest structures using terrestrial LiDAR. PloS one. 2017. V. 12. № 5. P. e0176871.
- Burt A., Disney M., Calders K. Extracting individual trees from lidar point clouds using treeseg. Methods in Ecology and Evolution. 2019. V. 10. № 3. P. 438–445.
- Eroshenkova D. A. et al. Automated determination of forest-vegetation characteristics with the use of a neural network of deep learning. International Conference on Neuroinformatics. Springer, Cham, 2019. P. 295–302.
- Hryashchev V.V. i dr. Segmentaciya ob"ektov na sputnikovyh izobrazheniyah s ispol'zovaniem svertochnyh nejronnyh setej. Uspekhi sovremennoj radioelektroniki. 2019. № 6. S. 28–34.
- Kvasnov A.V., Gladilin P.E., Pershutkin A.E. Metodika raspoznavaniya nepodvizhnyh gruppovyh ob"ektov po radiolokacionnomu izobrazheniyu na osnove iskusstvennyh nejronnyh setej. Uspekhi sovremennoj radioelektroniki. 2020. T. 74. № 8. S. 63–71 (In Russian).
- Zhang W. et al. Cloth simulation-based construction of pit-free canopy height models from airborne LiDAR data. Forest Ecosystems. 2020. V. 7. № 1. P. 1–13 (In Russian).
- Campello R. J. G. B., Moulavi D., Sander J. Density-based clustering based on hierarchical density estimates. Pacific-Asia conference on knowledge discovery and data mining. Springer, Berlin, Heidelberg, 2013. P. 160–172.
- Rangarajan P. et al. Hyper least squares and its applications. 2010 20th International Conference on Pattern Recognition. IEEE, 2010. P. 5–8.
- Barber C. B., Dobkin D. P., Huhdanpaa H. The quickhull algorithm for convex hulls. ACM Transactions on Mathematical Software (TOMS). 1996. V. 22. № 4. P. 469–483.