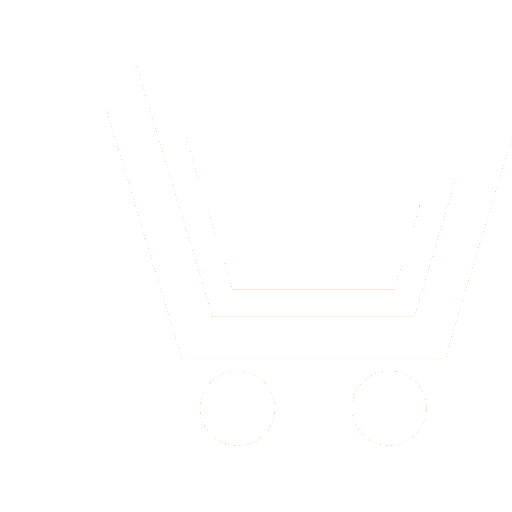
T.Yu. Tsibizova1, Abderrazek Abdessellem2
1–3 Bauman Moscow State Technical University (Moscow, Russia)
Formulation of the problem. In the manufacture of products and structures in modern technological processes, various methods of welded joints are used. The issue of quality control is relevant. This article discusses the issue of quality control of welded joints using intelligent computing methods and machine and deep learning algorithms.
The goal is to develop methods based on computer calculations that provide safe non-contact and non-destructive methods for quality control of welded joints. These methods include the method of non-destructive testing of image acquisition, the feature extraction method for extracting and converting features from an image, as well as a classifier for carrying out the procedure for classifying welded joints.
Results: A database of images of welded joints is being collected. The possibility of using convolutional neural network models VGGNET and ALEXNET for image classification of welded joints is being studied. Architectures of convolutional models are created. Models are trained and tested in the MATLAB environment. The effectiveness of VGGNET and ALEXNET is measured in terms of accuracy and speed of task execution.
Practical significance: the possibility of using ALEXNET and VGGNET convolutional neural network models for the classification of welded joints is shown, providing high accuracy of quality control.
Tsibizova T.Yu., Abderrazek Abdessellem. Use of ALEXNET and VGGNET convolutional networks in the problem of image classification of welded joints. Dynamics of complex systems. 2022. V. 16. № 2. P. 52−58. DOI: 10.18127/j19997493-202202-02 (in Russian).
- Aleshin N.P., Gladkov E.A., Gavrilov A.I., Perkovskij R.A., Rahmatullin T.A. Realizaciya adaptivnyh tekhnologij svarki kol'cevyh stykov magistral'nyh truboprovodov. Svarka i diagnostika. 2011. № 5. S. 49–53 (in Russian).
- Cibizova T.Yu., Guzeva T.A. Sistemy avtomaticheskogo upravleniya tekhnologicheskimi processami otverzhdeniya izdelij iz polimernyh kompozitov. Klei. Germetiki. Tekhnologii. 2015. № 5. S. 35–40 (in Russian).
- Gavrilov A.I., Gladkov E.A., Min M.T., Situ A.S., Thet A. Primenenie tekhnologij cifrovoj obrabotki izobrazhenij v nejrosetevyh sistemah upravleniya svarochnym oborudovaniem. Internet-zhurnal Naukovedenie. 2015. T. 7. № 1 (26). S. 61 (in Russian).
- Ovchinnikov V.V. Kontrol' kachestva svarnyh soedinenij: uchebnik dlya stud. uchrezhdenij sred. prof. obrazovaniya. Izd. 5-e, ster. M.: Izdatel'skij centr «Akademiya». 2016. 208 s. (in Russian).
- Gladkov E.A., Perkovskij R.A., Galkin V.A., Geckin B.L., Gavrilov A.I., Brodyagin V.N. Avtomatizirovannyj kompleks dlya mnogoslojnoj svarki kol'cevyh stykov trub magistral'nyh truboprovodov so sredstvami adaptacii i prognozirovaniya kachestva svarki. Nauka i tekhnika v gazovoj promyshlennosti. 2009. № 4 (40). S. 77–86 (in Russian).
- Sudheera K., Nandhitha, N.M. Computer Aided Radiograph Interpretation Tool for Defect Characterization from Weld Plates. Russian Journal of Nondestructive Testing. 2019. V. 55. P. 481–488.
- Nikishechkin A.P., Abderrazek A. Formirovanie primerov obuchayushchej i testovoj vyborki nejronnoj seti na principah planirovaniya eksperimentov. Evrazijskij soyuz uchenyh. 2016. № 3–1 (24). S. 153–156 (in Russian).
- Iskra N.A. Podhod k analizu izobrazhenij dlya sistem tekhnicheskogo zreniya. Doklady Belorusskogo gosudarstvennogo universiteta informatiki i radioelektroniki. 2020. T. 18. № 2. S. 62–70 (in Russian).
- Rawat W., Wang Z. Deep convolutional neural networks for image classification: A comprehensive review. Neural computation. 2017. T. 29. № 9. S. 2352–2449.
- Sergeev A.P. Tarasov D.A. Vvedenie v nejrosetevoe modelirovanie: ucheb. posobie. Pod obshch. red. A.P. Sergeeva. Izd. 2-e, ster. M.: FLINTA; Ekaterinburg: Izd-vo Ural'skogo universiteta. 2020. 128 s. (in Russian).
- Yadav S.S., Jadhav S.M. Deep convolutional neural network based medical image classification for disease diagnosis. Journal of Big Data. 2019. T. 6. №1. S. 113.