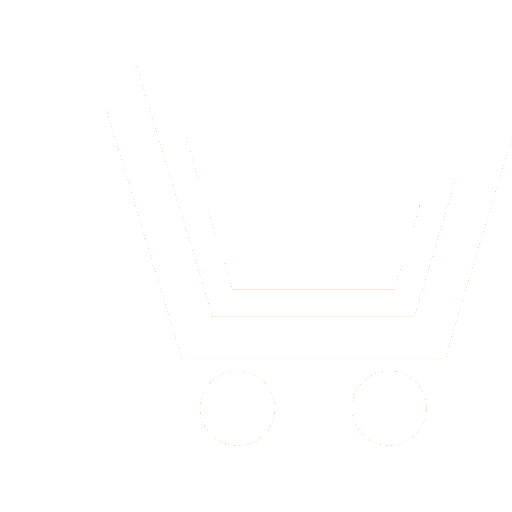
D.V. Berezkin1, Ahmad Hamed2, Moudar Kiwan3
1 Bauman Moscow State Technical University (Moscow, Russia)
The article considers the methods of extraction and representation of image features, which play an important role in the processing of multimedia data. An overview of the latest developments in the field of image feature extraction is performed: extraction of color features from images where color has many functions, such as color moments(CM), color coherence vector (CCV), etc., extraction of texture features using the Gabor filter and extraction of shape features using methods based on contours and regions. An overview of the methods for representing image features is performed. The efficiency of merging global and local features in image processing was analyzed, as well as another way of presenting features like a visual word package.
In the course of further work, the authors plan to study the relationship between the number of functions and the final performance.
Intuitively, the larger the number of functions, the better the final performance. Secondly, the study of the relationship between the representation of functions and the final performance is also a very interesting and complex topic. It includes methods for representing objects (global, block, and regional). In the case of using block and regional functions, it is necessary to investigate the performance, which depends on the size of the partition or segmentation. Third, it is supposed to investigate the relationship between a suitable combination of image functions and the final performance in order to assess whether this combination can further improve performance.
The results of the research are supposed to be used in the geolocation system of photos based on the automatic analysis of their images. Improving the accuracy and performance of image feature extraction will help improve the quality of the geopositioning system proposed in the article [28].
Berezkin D.V., Ahmad Hamed, Moudar Kiwan. Analysis of methods of image feature extraction. Dynamics of complex systems. 2021. T. 15. № 4. Р. 54−65. DOI: 10.18127/j19997493-202104-07 (In Russian)
- Stanchev P.L., Green Jr. D., Dimitrov B. High level colour similarity retrieval. International Journal of Information Theories and Applications. 2003. V. 10. № 3. P. 363–369.
- Chow T.W.S., Rahman M.K.M. A new image classification technique using tree-structured regional features. Neurocomputing. 2007. V. 70. № 4–6. P. 1040–1050.
- Tsai C.F., Lin W.C. A comparative study of global and local feature representations in image database categorization. In Proc. 5 th International Joint Conference on INC. IMS & IDC. 2009. P. 1563–1566.
- Jain A.K., Vailaya A. Image retrieval using colour and shape. Pattern Recognition. 1996. V. 29. № 8. P. 1233–1244.
- Flickner M., Sawhney H., Niblack W. et al. Query by image and video content: the QBIC system. IEEE Computer. 1995. V. 28, № 9. P. 23–32.
- Pass G., Zabith R. Histogram refinement for content-based image retrieval, In Proc. Workshop on Applications of Computer Vision. 1996. P. 96–102.
- Huang J., Kuamr S., Mitra M. et al. Image indexing using colour correlogram. In Proc. CVPR. 1997. P. 762–765.
- Pass G., Zabih R. Comparing images using color coherence vectors. I4th ACM Multimedia Conference. P. 65–73.
- Manjunath B.S., Ma W.Y. Texture features for browsing and retrieval of large image data. IEEE PAMI. 1996. V. 18. № 8. P. 837–842.
- Grigorescu S.E., Petkov N., Kruizinga P. Comparison of texture features based on Gabor filters. IEEE TIP. 2002. V. 11. № 10. P. 1160–1167.
- Zhang D., Lu G. Review of shape representation and description techniques, Pattern Recognition. 2004. V. 37. № 1. P. 1–19.
- Yang C., Dong M., Fotouhi F. Image content annotation using Bayesian framework and complement components analysis. In Proc. ICIP. 2005.
- Mezaris V., Kompatsiaris I., Strintzis M.G. An ontology approach to object-based image retrieval. In Proc. ICIP. 2003. P. 511–514.
- Zhang D., Islam M.M., Lu G. et al. Semantic image retrieval using region based inverted file. In Proc. DICTA. 2009. P. 242–249.
- Yang M., Kpalma K., Ronsin J. A survey of shape feature extraction techniques. Pattern Recognition, 2008. P. 43–90.
- Deng Y.N., Manjunath B.S. Unsupervised segmentation of color-texture regions in images and video. IEEE PAMI. 2001. V. 23. № 8.
- 800–810.
- Lowe D.G. Distinctive image features from scale-invariant keypoints. International Journal of Computer Vision. 2004. V. 60. № 2.
- 91–110.
- Chow T.W.S., Rahman M.K.M. A new image classification technique using tree-structured regional features. Neurocomputing. 2007. V. 70. № 4–6. P. 1040–1050.
- Tsai C.F., Lin W.C. A comparative study of global and local feature representations in image database categorization. In Proc. 5 th International Joint Conference on INC. IMS & IDC. 2009. P. 1563–1566.
- Zhu J., Hoi S., Lyu M. et al. Near-duplicate keyframe retrieval by nonrigid image matching. In Proc. ACM MM. 2008. P. 41–50.
- Tian D., Zhao X.F., Shi Z. Support vector machine with mixture of kernels for image classification. In Proc. ICIIP. 2012. P. 67–75.
- Lisin D.A., Mattar M.A., Blaschko M.B. et al., Combining local and global image features for object class recognition. In Proc. Workshop on CVPR. 2005.
- Zhao J., Fan Y., Fan W. Fusion of global and local feature using KCCA for automatic target recognition. In Proc. ICIG. 2009. P. 958–962.
- Zhou X., Cui N., Li Z. et al. Hierarchical gaussianization for image classification. In Proc. ICCV. 2009. P. 1971–1977.
- Monay F., Gatica-Perez D. Modeling semantic aspects for cross-media image indexing. IEEE PAMI. 2007. V. 29. № 10. P. 1802–1817.
- Tian D., Zhao X., Shi Z. Refining image annotation by integrating PLSA with random walk model. In Proc. MMM. Part I, LNCS 7732. 2013. P. 13–23.
- Yang J., Jiang Y., Hauptmann A.G., Ngo C. Evaluating bag-of-visual-words representations in scene classification. In Proc. Workshop on MIR. 2007. P. 197–206.
- Berezkin D., Proletarsky A., Sukhorukova N., Kamalov R. Specifics of implementing a hybrid intelligent image georeferencing system. Proceedings of the 17th International Conference on Applied Computing 2020. Virtual. 2020. Nov. 18–20. P. 115–118.
- Mingqiang Yang, Kidiyo Kpalma, Ronsin J. A Survey of Shape Feature Extraction Techniques. Peng-Yeng Yin. Pattern Recognition, INTECH. 2008. P. 43–90. ffhal-00446037f
- Weinzaepfel P., Jegou H., Perez P. Reconstructing an image from its local descriptors. In Computer Vision and Pattern Recognition. Colorado Springs, United States. Jun. 2011.
- Dipankar Hazra, Retrieval of Color Image using Color Correlogram and Wavelet Filters. Proc. of Int. Conf. on Advances in Computer Engineering. 2011.
- Wang Surong, Chia Liang-Tien, Deepu Rajan. Image Retrieval Using Dominant Color Descriptor. Conference: Proceedings of the Inter-national Conference on Imaging Science. Systems and Technology. CISST '03, June 23–26. 2003.