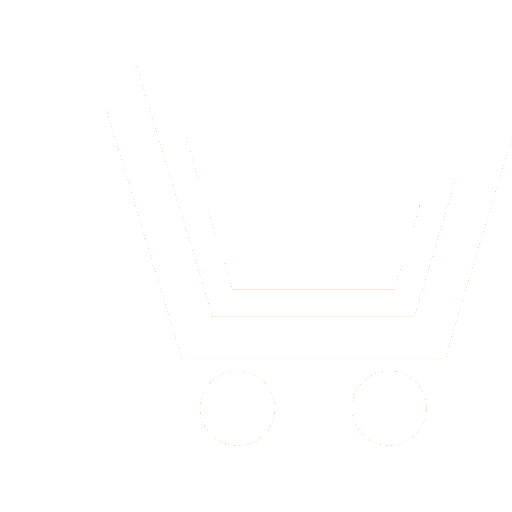
A.I. Kanev – Post-graduate Student,
Bauman Moscow State Technical University (Moscow, Russia) E-mail: kanevai@student.bmstu.ru
Using traditional information retrieval, the user is faced with the need of repeated queries and analysis of intermediate data if he cannot accurately formulate the initial query. Therefore, in recent years, ways to increase the capabilities of information retrieval have been actively developing: query cards, visual presentation of document topics, neural networks.Another direction is semantic search. The author of this article proposes to use semantic search and text mining to more accurately interpret user queries and improve search quality. One of the main tasks for text mining is representation of knowledge gained during natural language processing. Two main approaches are used for natural language processing: rule-based and statistical with machine learning. Each of them has their advantages and disadvantages. The metagraph approach allows to combine soft computing and knowledge processing methods andit is chosen to implement the representation of knowledge obtained in the process of text mining. Attributes of metavertexes and metaedgeswith real number values are used for machine learning.
The purpose of this paper is a description of requirements for a semantic search system using text mining and metagraph knowledge base.
The paper describes formulas for ranking query results using the knowledge gained in the analysis of the query and text documents. They are required to combine index results for various concepts and relations from the knowledge base.
Two variants for ranking query results differs one form each other in way of knowledge processing. The first one obtains concepts and relations only from queries and uses classic search index for words with the same meanings from knowledge base. The second variant gains concepts and relations from queries and documents and usesspecial semantic index to search documents.
The proposed information retrieval technique can be used to improve the accuracy and completeness of search engines primarily on small amounts of data that are not enough for existing methods.The emergence property of the metagraph allows to store and process data from small amount of information, gradually detailing it. Using a single knowledge base for all languages it allows to index documents and process queries simultaneously in different languages.
- Ryen W. White and Resa A. Roth. Exploratory Search: Beyond the Query-Response Paradigm, Synthesis Lectures on Information Concepts, Retrieval, and Services. Morgan and Claypool Publishers. 2009. V. 1. 98 p.
- Manicheva E., Petrova M., Kozlova E., Popova T. Compreno Semantic Model as an Integral Framework for a Multilingual Lexical Database. 24th International Conference on Computational Linguistics, Proceedings of the 3rd Workshop on Cognitive Aspects of the Lexicon (CogALex-III). 2012. P. 215−230.
- Keith van Rijsbergen. Information retrieval. Second edition. London: Butterworths. 1979. URL: http://www.dcs.gla.ac.uk/Keith/Preface.html.
- Mooers C. Information retrieval viewed as temporal signaling. Proceedings of the International Congress of Mathematicians. 1950. V. 1. P. 572–573.
- Guha R., McCoolR., Miller E. Semantic Search. WWW '03: Proceedings of the 12th international conference on World Wide Web. 2003.
- Zhang Y., Chen M., Liu L. Review on Text Mining. 2015 6th IEEE International Conference on Software Engineering and Service Science (ICSESS). 2015.
- Sukanya M., Biruntha S. Techniques on Text Mining. 2012 IEEE International Conference on Advanced Communication Control and Computing Technologies (ICACCCT). 2012.
- Sanchez D., Martın-Bautista M.J., Blanco I. Text Knowledge Mining: An Alternative to Text Data Mining. 2008 IEEE International Conference on Data Mining Workshops. 2008.
- Yin S., Qiu Y., Ge J .Research and Realization of Text Mining Algorithm on Web. 2007 International Conference on Computational Intelligence and Security Workshops (CISW 2007). 2008.
- Witten I.H., Frank E., Hall M. Data Mining: Practical Machine Learning Tools and Techniques. - Morgan Kaufmann. 2016. P. 654.
- Amarasiri R., Ceddia J., Alahakoon D. Exploratory Data Mining Lead by Text Mining Using a Novel High Dimensional Clustering Algorithm. Fourth International Conference on Machine Learning and Applications (ICMLA'05). 2005.
- Verma V.K. Text Mining and Information Professionals. 2015 4th International Symposium on Emerging Trends and Technologies in Libraries and Information Services. 2015.
- Qi Y. Text Mining for Bioinformatics: State of the Art Review. 2009 2nd IEEE International Conference on Computer Science and Information Technology. 2009.
- Pizzi N., Krishnamoorthy R. Clinical Text Mining for Improved Patient Characterization. 2014 IEEE International Congress on Big Data. 2014.
- Gong T., Tan C.L., Leong T.Y. Text Mining in Radiology Reports. 2008 Eighth IEEE International Conference on Data Mining. 2008.
- Hu Z.Z., Cohen K.B., Hirschman L., Valencia A., Liu H., Giglio M.G., Wu C.H. iProLINK: A Framework for Linking Text Mining with Ontology and Systems Biology. 2008 IEEE International Conference on Bioinformatics and Biomedicine. 2008.
- Pinho I.C., Epstein D., Berni E., Corrêa R.,Corrêa Y. The Use of Text Mining to Build a Pedagogical Agent Capable of Mediating Synchronous Online Discussions in the Context of Foreign Language Learning. 2013 IEEE Frontiers in Education Conference (FIE). 2013.
- Wang K.Q., Wu Q.K., Mao H.Y., Zhou M.B., Jiang K., Zhu X.P., Yang L., Wang T., Wang H.Q. Intelligent Text Mining Based Financial Risk Early Warning System. 2015 2nd International Conference on Information Science and Control Engineering. 2015.
- Huosong X., Zhaoyan F., Liuyan P. Chinese Web Text Outlier Mining Based on Domain Knowledge. 2010 Second WRI Global Congress on Intelligent Systems. 2010.
- Mikolov T., Le Q.V., Sutskever I. Exploiting Similarities among Languages for Machine Translation. 2013. URL: https://arxiv.org/pdf/1309.4168.pdf.
- Anisimovich K.V., Druzhkin K.Ju., Zuev K.A., Minlos F.R., Petrova M.A., Selegeĭ V.P. Sintaksicheskij i semanticheskij parter, osnovannyj na lingvisticheskih tehnologijah ABBYY Compreno. XVIII Mezhdunarodnaja konferencija «Dialog 2012». 2012. S. 91−103 (In Russian).
- Sutskever I., Vinyals O., Le Q.V. Sequence to Sequence Learning with Neural Networks. Advances in neural information processing systems. 2014.
- Cho K., van Merrienboer B.,Gulcehre C., Bahdanau D., Bougares F., Schwenk H., Bengio Y. Learning Phrase Representations using RNN Encoder–Decoder for Statistical Machine Translation. Proceedings of the 2014 Conference on Empirical Methods in Natural Language Processing (EMNLP). 2014. P. 1724−1734.
- Radford A., Narasimhan K., Salimans T., Sutskever I. Improving Language Understanding by Generative Pre-Training. 2018. URL: https://s3-us-west-2.amazonaws.com/openai-assets/research-covers/language-unsupervised/language_understanding_paper.pdf.
- Sussna M. Word sense disambiguation for free-text indexing using a Massive Semantic Network. Proceedings of the second international conference on Information and knowledge management. 1993. P. 67−74.
- Shapiro S. Encyclopedia of Artificial Intelligence. Second edition. Wiley. 1992.
- Chernen'kij V.M., Gapanjuk Ju.E., Revunkov G.I., Terehov V.I., Kaganov Ju.T. Metagrafovyj podhod dlja opisanija Gibridnyh Intellektual'nyh Informacionnyh Sistem. Prikladnaja informatika. 2017. T. 12. № 3(69). S. 57−79 (In Russian).
- Nakagochi R., Kawamoto K., Sunayama W. Acquissition of Text-Mining Skills for Beginners Using TETDM. 13th International Conference on Data Mining Workshops. 2013.
- Otsuka N., Matsushita M. Constructing Knowledge Using Exploratory Text Mining. Joint 7th International Conference on Soft Computing and Intelligent Systems (SCIS) and 15th International Symposium on Advanced Intelligent Systems (ISIS). 2014.
- Sunayama W. Knowledge Emergence using Total Environment for Text Data Mining //Joint 7th International Conference on Soft Computing and Intelligent Systems (SCIS) and 15th International Symposium on Advanced Intelligent Systems (ISIS). 2014.
- Revunkov G.I., Gapanjuk Ju.E., Fedorenko Ju.S. Opisanie nejronnoj seti s ispol'zovaniem metagrafovogo podhoda. Estestvennye i tehnicheskie nauki. 2016. № 12. S. 278−281 (In Russian).