350 rub
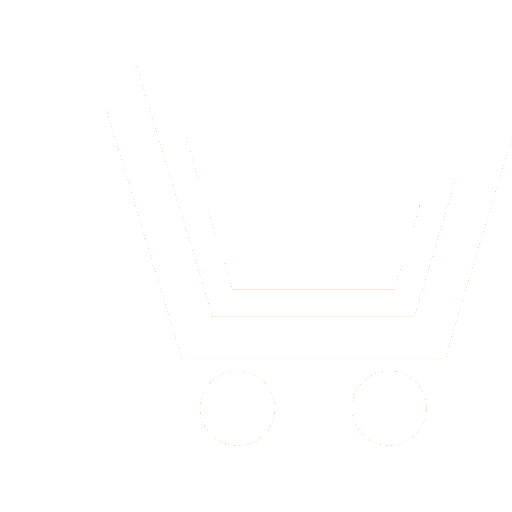
Journal Dynamics of Complex Systems - XXI century №3 for 2016 г.
Article in number:
The model order reduction method on bases Volterra series
Authors:
A.A. Dolinina - Post-graduate Student, Department of Computer Engineering, Vladimir State University named after A.&N. Stoletovs. E-mail: Nastya.doly@gmail.com
V.N. Lantsov - Dr. Sc. (Eng.), Professor, Head of Department of Computer Engineering, Vladimir State University named after A.&N. Stoletovs. E-mail: lantsov@vlsu.ru
Abstract:
Over the past decade a large body of work on model order reduction of complex circuits and systems has emerged from the design automation community. The purpose of model reduction is to generate models that are orders of magnitude smaller than the original system, while accurately approximating the input-output relationships of the original system. Compared to the success of model order reduction for linear time invariant resistance-inducatance-capacitance networks, the problem of reducing nonlinear systems has been less understood and explored.
There are numerous applications, however, where abstracting transistor-level circuit details that include important weakly nonlinear effects into a compact macromodel is important. For instance, in RF communication IC design there is a growing interest in extracting efficient circuit-level models which are capable of capturing system nonlinear distortion. While circuit blocks in these applications often exhibit weak nonlinearities, the design specification for linearity is often extremely important and very stringent.
Model order reduction for a class of weakly nonlinear systems that can be well characterized by low-order Volterra functional series is now the area of interests. The automatically extracted reduced circuits (or macromodels) capture not only the first-order (linear) system properties, but also the important second-order and third-order effects of interest that cannot be neglected for a broad range of applications. Unlike the existing projection-based reduction methods for weakly nonlinear systems (for example NORM) begins with the general matrix-form Volterra nonlinear transfer functions to derive a set of Krylov subspaces for order reduction. Moment matching of the nonlinear transfer functions by projection of the original system onto this set of minimum Krylov subspaces leads to reduction of model size. But the simulation time is still large.
In this paper we will demonstrate new algorithms that accelerate simulation. The comparison with existing methods shows the efficacy of model reduction for weakly nonlinear systems is determined by the achievable model compactness. Our results indicate that singular value decomposition (SVD) approach can substantially improve the model compactness for nonlinear system reduction. Furthermore, we show that the structure of the nonlinear system can be exploited to simplify the reduced model in practice, which is particularly effective for circuits with frequency selectivity.
Pages: 50-54
References
- Lancov V.N. Metody ponizhenija porjadka modelejj slozhnykh ehlektronnykh skhem (obzor) // Radiotekhnicheskie i telekommunikacionnye sistemy. 2012. № 3. S. 59−65.
- Lancov V.N., Dolinina A.A. Pankratov A.V. Algoritmy makromodelirovanija slozhnykh nelinejjnykh sistem // Dinamika slozhnykh sistem - XXI vek. 2014. T. 8. № 5. S. 23−30.
- Celik M., Cangellaris A.C. Simulation of multiconductor transmission lines using Krylov subspace order-reduction techniques // IEEE Trans. Computer-Aided Design. May 1997. V. 16. P. 485−496.
- Heydari P., Pedram M. Model-order reduction using variational balanced truncation with spectral shaping // IEEE Trans. on Circuits and Systems. Pt. 1 - Regular papers. 2006. V. 53. № 4. P. 879−891.
- Dong N., Roychowdhury J. General-purpose nonlinear model-order reduction using picewise-polynomial representations // IEEE Trans. on Computer-Aided Design of Integr. Circuits and Systems. 2008. V. 27. № 2. P. 249−264.
- Ilin V.N., ZHigalov I.E., Lancov V.N. Metody avtomatizirovannogo skhemotekhnicheskogo proektirovanija nelinejjnykh radiotekhnicheskikh cepejj (obzor) // Izvestija VUZov. Radioehlektronika. 1985. № 6. S. 7−17.
- Li P., Pileggi L. Compact reduced-order modeling of weakly nonlinear analog and RF circuits // IEEE Trans. Comput.-Aided Des. Integr. Circuits Syst. Feb. 2005. V. 24. № 2. P. 184−203.
- Roychowdhury J. Reduced-order modelling of time-varying systems // IEEE Trans. Circuits Syst.-II: Analog Digital Signal Process. Oct. 1999. V. 46. № 10. P. 1273−1288.
- Phillips J.R. Projection-based approaches for model reduction of weakly nonlinear time-varying systems // IEEE Trans. Comput.-Aided Des. Feb. 2003. V. 22. № 2. P. 171−187.
- Lantsov V.N. Methods of analysis of nonlinear RF circuits in CAD tools // Proc. of Conference the Experience of Designing and Application of CAD System in Microelectronics, CADSM-2005. 23−26 February 2005. Lviv-Slavske (Ukraine). P. 23−28.
- Bond B.N., Daniel L. Stable reduced model for nonlinear descriptor systems through piecewise-linear approximation and projection // IEEE Trans. Computer-Aided Design. 2009. V. 26. № 12. P. 2116−2129.
- Dolinina A. Macromodeling of Electronic Circuits Based on Model Order Reduction // IEEE North West Russia Section Young Researchers in Electrical and Electronic Engineering Conference. February 2015. P. 146−150.
- Dolinina A.A., Lancov V.N. Algoritm modelirovanija nelinejjnykh ustrojjstv na osnove metodov ponizhenija porjadka modelejj i kusochno-linejjnojj approksimacii // Radiotekhnicheskie i telekommunikacionnye sistemy. 2014. № 3. S. 65−69.