350 rub
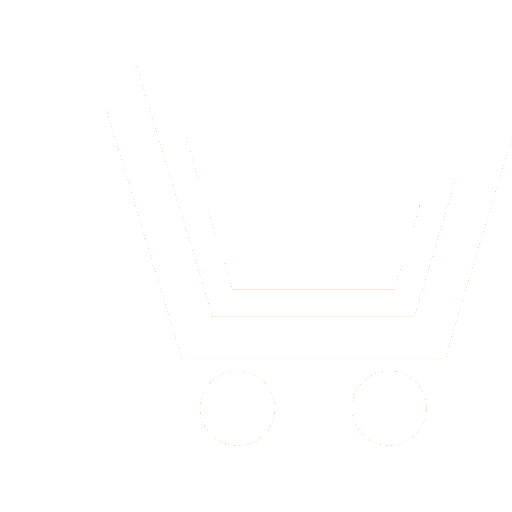
Journal Dynamics of Complex Systems - XXI century №5 for 2014 г.
Article in number:
The macromodeling algorithms for complex nonlinear electronic systems
Keywords:
nonlinear systems
macromodelling
model-order-reduction
singular values
piecewise-polynomial approximation
dynamic neural sets
simulation
Authors:
V.N. Lantsov - Dr.Sc.(Eng.), Professor, Vladimir State University named after A.&N. Stoletovs. E-mail: lant-sov@vlsu.ru
A.A. Dolinina - Post-graduate student, Vladimir State University named after A.&N. Stoletovs. E-mail: Nas-tya.doly@gmail.com
A.V. Pankratov - Post-graduate student, Vladimir State University named after A.&N. Stoletovs. E-mail: Aleksey112358@gmail.com
Abstract:
Circuit simulators and other electronic-design automation tools today are being increasingly challenged by the ever-growing size and complexity of mixed-signal integrated systems. To enable an effective verification with reasonable computation, it has become commonplace to replace large system blocks with smaller ones or, in other words, to use macromodels to speed up simulations of interconnected system blocks.
In this work we present the two algorithms of simulation and automated macromodelling of complex nonlinear electronic systems. A key feature of our algorithms is that they automate the generation of general-purpose macromodels that are suitable for a wide range of time and frequency domain analyses in mixed-signal design flows. Our macromodels not only reproduce small-signal distortion and intermodulation properties well but also retain fidelity in large-signal transient analyses. The reduced models can be used as drop-in replacements for large subsystems to achieve fast system-level simulation using a variety of time- and frequency-domain analyses (such as dc, ac, transient, harmonic balance, etc.).
Into the first algorithm we are used the model order reduction methods and piecewise-polynomial representations for nonlinear functions. Here we are firstly approximate the nonlinear system as combination of linear models, which we are obtain as piecewise-polynomial approximation of system at points along the training trajectories. Then each of linear models is reduced to a smaller one via model order reduction methods (MOR). We are used here the singular value decomposition algorithms.
The second algorithm use the dynamic neural network (DNN) model which can be developed directly from input-output data without having to rely on internal details of the circuit. An algorithm is developed to train the model with time or frequency domain information. Efficient representations of the model are proposed for convenient incorporation of the DNN into high-level circuit simulation. Compared to existing neural-based methods, the DNN retains or enhances the neural modeling speed and accuracy capabilities, and provides additional flexibility in handling diverse needs of nonlinear microwave simulation, e.g., time- and frequency-domain applications, single-tone and multitone si-mulations.
Pages: 23-31
References
- Lancov V.N. Metody ponizhenija porjadka modelejj slozhnykh ehlektronnykh skhem (obzor) // Radiotekhnicheskie i telekommunikacionnye sistemy. 2012. № 3. S. 59−65.
- Roychowdhury J. Reduced-order modelling of time-varying systems // IEEE Trans. Circuits Syst. II, Analog Digit. Signal Process. 1999. Nov. Vol. 46. № 10. P. 1273−1288.
- Phillips J.R. Projection-based approaches for model reduction of weakly nonlinear, time-varying systems // IEEE Trans. Comput.-Aided Design Integr. Circuits Syst. 2003. Feb. Vol. 22. № 2. P. 171−187.
- Rewienski M., White J. A trajectory piecewise-linear approach to model order reduction and fast simulation of nonlinear circuits and micromachined devices // IEEE Trans. Comput.-Aided Design Integr. Circuits Syst. 2003. Vol. 22. № 2. P. 155−170.
- Bond B., Daniel L. Stable Reduced Models for Nonlinear Descriptor Systems through Piecewise-Linear Approximation and Projection // IEEE Trans. on Computer-Aided Design of Integrated Circuits and Systems. 2009. Vol. 26. № 12. P. 2116−2129.
- Xu J., Yagoub M.C.E., Ding R., Zhang Q-J. Neural-based dynamic modeling of nonlinear microwave circuits // IEEE Trans. on MTT. 2002. Vol. 50. № 12. P. 2769−2780.
- Lancov V.N. Sostojanie v oblasti metodov modelirovanija nelinejjnykh VCH ehlektronnykh ustrojjstv svjazi (obzor). CHast 1 // Proektirovanie i tekhnologija ehlektronnykh sredstv. 2012. № 4. S. 2−11.
- Pankratov A.V., Lancov V.N. Nelinejjnaja makromodel v vide dinamicheskojj nejjronnojj seti dlja zadach analiza metodom garmonicheskogo balansa // Proektirovanie i tekhnologija ehlektronnykh sredstv. 2013. № 4. S. 7−11.
- Xu J., Yagoub M.C.E., Ding R., Zhang Q-J. Exact adjoint sensitivity analysis for Neural-based microwave modeling and design // IEEE Trans. on MTT. 2003. Vol. 51. № 1. P. 226−237.
- Lancov V.N. Metod prisoedinennojj skhemy dlja rascheta kharakteristik nelinejjnykh radiotekhnicheskikh ustrojjstv // Integrirovannye sredstva avtomatizirovannogo proektirovanija REHS. M.: MAI. 1991. S. 16−23.
- Pankratov A.V., Lancov V.N. Metod rascheta JAkobiana dlja analiza nelinejjnykh skhem na osnove prisoedinennojj dinamicheskojj nejjronnojj seti // Proektirovanie i tekhnologija ehlektronnykh sredstv. 2014. № 1. S. 21−24.